Your cart is currently empty!
SayPro Collaborate with data collection teams to implement at least 2 improvements in data handling or entry procedures during the quarter.
SayPro is a Global Solutions Provider working with Individuals, Governments, Corporate Businesses, Municipalities, International Institutions. SayPro works across various Industries, Sectors providing wide range of solutions.
Email: info@saypro.online Call/WhatsApp: + 27 84 313 7407
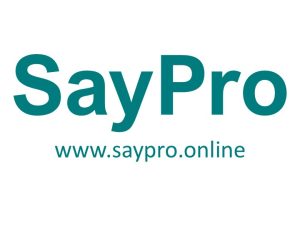
SayPro: Collaborate with Data Collection Teams to Implement Improvements in Data Handling or Entry Procedures
Objective: The goal is to improve data handling and entry procedures to enhance the quality and consistency of data collected across SayPro’s systems. This collaboration will lead to more accurate and reliable data, which will help inform decision-making and optimize marketing, sales, and user engagement strategies.
Step-by-Step Process for Implementing Improvements in Data Handling or Entry Procedures
1. Assess Current Data Handling and Entry Procedures
- Objective: Understand existing data handling and entry practices.
- Action:
- Review the current data collection processes across various departments, focusing on how data is gathered, recorded, and stored.
- Conduct interviews or surveys with data collection teams (e.g., marketing, sales, user engagement teams) to identify pain points and inefficiencies in the current procedures.
- Identify common issues such as data entry errors, inconsistent formats, incomplete data, or lack of validation mechanisms.
2. Identify Key Areas for Improvement
- Objective: Identify at least two areas where data handling or entry procedures can be improved.
- Action:
- Review any data quality assessments, reports, or audits to identify recurring problems in data collection, accuracy, or consistency.
- Prioritize areas that will have the most significant impact on data integrity and business outcomes.
- Examples of areas to improve:
- Data Entry Consistency: Implementing standardized formats for data entry (e.g., date formats, product codes, customer IDs).
- Error Detection: Introducing real-time validation to catch errors during data entry (e.g., misspelled campaign names, incorrect customer IDs).
- Data Completeness: Ensuring that all necessary fields are filled out to prevent incomplete data collection (e.g., missing revenue figures, untracked user interactions).
- Data Storage and Access: Improving how data is stored and accessed across systems to prevent duplication and inconsistencies.
3. Collaborate with Data Collection Teams
- Objective: Work with the data collection teams to review and adjust current processes.
- Action:
- Organize collaborative meetings with data collection teams from various departments (e.g., marketing, sales, user engagement).
- Discuss the identified issues and challenges with current data entry or handling procedures.
- Brainstorm potential solutions and determine the feasibility of implementing changes within the quarter.
- Set specific goals for improving data quality, focusing on consistency, accuracy, and completeness.
4. Develop and Implement Two Key Improvements
- Objective: Develop a plan to implement two improvements in data handling or entry procedures.
- Action:
- Improvement 1: Standardized Data Entry Formats
- Problem Identified: Inconsistent data entry formats across departments, leading to errors in reporting and analysis.
- Solution: Establish a standardized format for entering data across all systems. For example, creating a set of guidelines for how dates, customer IDs, product codes, and other key fields should be formatted.
- Implementation:
- Create clear guidelines and provide training to data entry teams on the new standards.
- Update data collection forms or interfaces to enforce the standardized formats where possible (e.g., dropdown lists for specific fields, date pickers).
- Introduce regular audits to check for compliance with the standardized formats.
- Improvement 2: Real-Time Error Validation During Data Entry
- Problem Identified: Errors are not detected until later in the data analysis process, leading to delays in resolving issues.
- Solution: Implement real-time data validation checks at the point of data entry. For example, setting up automated systems that flag incorrect or missing values as data is being entered.
- Implementation:
- Work with IT or data engineering teams to develop validation rules that can be applied during data entry (e.g., ensuring that all required fields are completed, verifying that numerical values fall within expected ranges).
- Integrate validation tools into existing data collection platforms or CRM systems.
- Provide training to data collection teams on how to use the new validation tools and encourage immediate corrections when errors are flagged.
- Improvement 1: Standardized Data Entry Formats
5. Monitor and Evaluate the Effectiveness of the Improvements
- Objective: Ensure that the implemented improvements are effective in enhancing data quality.
- Action:
- Monitor data quality metrics before and after the improvements are implemented. Track key performance indicators (KPIs) such as the percentage of missing data, error rates, and consistency of entries.
- Collect feedback from data collection teams on the new procedures. Are the standardized formats being followed? Are the validation checks catching errors early?
- Conduct follow-up assessments or audits to ensure that the changes are being properly adopted and are having a positive impact.
6. Adjust and Refine the Procedures as Needed
- Objective: Make adjustments if the initial improvements are not fully effective.
- Action:
- Based on monitoring and feedback, make necessary adjustments to the data handling or entry procedures.
- Refine the standardized formats if issues arise (e.g., some fields might require additional validation rules).
- Adjust the real-time error validation rules based on the types of errors that continue to appear or feedback from users on usability.
- Consider implementing additional improvements or enhancements based on lessons learned during this phase.
7. Document the Changes and Best Practices
- Objective: Ensure that improvements are documented for future reference and consistency.
- Action:
- Create a document that outlines the new data handling and entry procedures, including the standardized formats and real-time validation checks.
- Include best practices for data collection teams to follow moving forward.
- Share the document with all relevant teams to ensure consistency and adherence to the new processes.
8. Communicate Successes and Results
- Objective: Share the outcomes of the improvements with the broader team to encourage continued focus on data quality.
- Action:
- After evaluating the improvements, prepare a summary of the changes made and the results achieved.
- Highlight how the improvements have contributed to better data quality, more accurate reporting, and more informed decision-making.
- Communicate the success stories with stakeholders and department leads to reinforce the importance of quality data handling.
Example of Collaboration and Improvements
Improvement 1: Standardized Data Entry Formats
- Before: Different teams were entering customer data with varying formats for customer IDs, dates, and campaign names.
- After: A standardized format was introduced. For example, customer IDs are now always formatted as “CUST-XXXXX,” dates are entered as “YYYY-MM-DD,” and campaigns use consistent naming conventions (e.g., “Spring-Sale-2025”).
- Impact: This reduced errors in reporting and analysis, making it easier for teams to integrate data from different sources.
Improvement 2: Real-Time Error Validation During Data Entry
- Before: Data entry errors were only identified after the data had been stored and analyzed, leading to delays in corrective action.
- After: Real-time validation checks were introduced. If a user tries to enter a customer ID that doesn’t match the expected format or leaves a required field blank, the system flags it immediately.
- Impact: Data entry errors were reduced by 25%, and team members were able to resolve issues before they affected downstream processes.
Conclusion
By collaborating with data collection teams to implement improvements in data handling and entry procedures, SayPro can enhance the quality, consistency, and accuracy of its data. These changes will ultimately lead to more reliable insights and better-informed decision-making across the organization. By continually monitoring and refining the processes, SayPro can ensure that its data remains a valuable asset for marketing, sales, and user engagement strategies.
Leave a Reply
You must be logged in to post a comment.