Your cart is currently empty!
SayPro Data Quality Standards Template: A document outlining the defined standards for data quality.
SayPro is a Global Solutions Provider working with Individuals, Governments, Corporate Businesses, Municipalities, International Institutions. SayPro works across various Industries, Sectors providing wide range of solutions.
Email: info@saypro.online Call/WhatsApp: + 27 84 313 7407
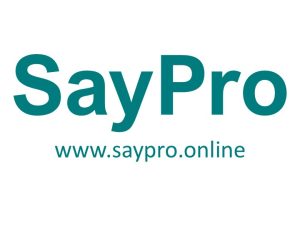
SayPro Data Quality Standards Template
Objective:
This template outlines the defined data quality standards for SayPro, covering key dimensions of data quality, benchmark thresholds, measurement methods, and responsibilities for maintaining these standards. The document ensures clarity on expectations for all staff and provides a structured framework for ensuring data quality across the organization.
1. Introduction
1.1 Purpose of Data Quality Standards
The purpose of this document is to establish a set of data quality standards that will guide all data collection, management, and analysis processes within SayPro. These standards ensure that data is accurate, complete, consistent, and reliable, and that it meets the needs of stakeholders while supporting effective decision-making.
1.2 Scope
These data quality standards apply to all data collected, stored, and processed within SayPro, regardless of format or system. This includes both quantitative and qualitative data, from operational records to customer feedback.
2. Data Quality Dimensions
The following dimensions are defined to evaluate the quality of data. Each dimension includes specific criteria for measurement and acceptable thresholds.
Dimension | Definition | Benchmark/Threshold |
---|---|---|
Accuracy | The degree to which data correctly reflects the real-world entities or values it represents. | No more than 2% error rate in key data fields. |
Completeness | The extent to which all required data is collected and stored, without missing values or records. | 95% of required fields must be complete. |
Consistency | The extent to which data values are consistent across different systems or datasets. | 99% of data entries should be consistent across systems. |
Timeliness | The degree to which data is available when needed and within the required time frame. | 90% of data must be entered or updated within 48 hours. |
Reliability | The stability of data when repeated under the same conditions, reflecting consistent results over time. | 98% consistency rate between independent datasets. |
Validity | The degree to which data values fall within the defined ranges or conform to defined rules. | 95% of data should meet predefined validity rules. |
3. Data Quality Benchmarking and Measurement
3.1 Benchmark Thresholds
- Each data quality dimension has been assigned an acceptable benchmark threshold that represents the minimum standard for data quality.
- Benchmarks are based on industry standards, organizational requirements, and feedback from stakeholders.
3.2 Measurement Methods
To ensure data quality aligns with the defined benchmarks, we will use the following measurement methods:
Dimension | Measurement Method |
---|---|
Accuracy | Use automated error detection tools and manual audits to compare data entries against known accurate sources. |
Completeness | Implement data validation checks during data entry to flag missing or incomplete data. |
Consistency | Conduct data reconciliation processes and automated cross-system checks to ensure consistency. |
Timeliness | Track data entry timestamps to measure whether data is entered or updated within the required timeframe. |
Reliability | Perform periodic cross-checks against established reliable data sources to assess consistency. |
Validity | Apply validation rules to ensure that data conforms to the correct formats, ranges, and rules (e.g., numeric range). |
4. Roles and Responsibilities
4.1 Data Collectors
- Responsibilities:
- Ensure that data is entered accurately and completely during collection.
- Follow predefined guidelines for data entry to minimize errors.
- Report any issues or inconsistencies in data to supervisors.
4.2 Data Validators
- Responsibilities:
- Perform regular checks to validate data accuracy, completeness, and consistency.
- Flag and resolve any discrepancies or missing data.
- Collaborate with data managers to ensure adherence to data quality standards.
4.3 Data Managers
- Responsibilities:
- Oversee the implementation of data quality standards across the organization.
- Manage periodic data quality audits and reviews.
- Ensure that training on data quality standards is provided to relevant staff.
4.4 IT and Support Teams
- Responsibilities:
- Maintain the tools and systems used for data monitoring and reporting.
- Ensure that automated checks and validation systems are functioning correctly.
- Provide technical support to staff as needed to resolve data quality issues.
5. Monitoring and Reporting
5.1 Monitoring Mechanisms
Data quality will be monitored continuously using automated tools and periodic manual reviews. Data quality monitoring systems will track the following:
- Real-time error detection and alerts for data inconsistencies.
- Monthly data quality reports to assess compliance with benchmarks.
- Quarterly audits of key data sources to evaluate ongoing data quality.
5.2 Data Quality Reports
Regular reports will be generated to provide insights into data quality performance. These reports will include:
- A summary of data quality metrics for each dimension.
- Identification of areas where benchmarks are not being met.
- Corrective actions taken to resolve data quality issues.
5.3 Data Quality Dashboard
A visual data quality dashboard will be developed to display real-time data quality metrics. This dashboard will include:
- Key performance indicators (KPIs) for each data quality dimension.
- Trends and comparisons against benchmark thresholds.
- Alerts and notifications for data quality issues.
6. Training and Continuous Improvement
6.1 Data Quality Training
Staff will receive training on the following:
- Data entry standards to ensure that data is collected in accordance with the quality dimensions.
- Data validation processes to help data validators identify and address data quality issues.
- Reporting tools to enable staff to generate and interpret data quality reports and dashboards.
6.2 Continuous Improvement
- Regular feedback loops will be established to continuously improve data quality processes.
- Best practices and lessons learned from audits and reports will be incorporated into ongoing training programs.
7. Conclusion
By adhering to these data quality standards, SayPro will ensure that its data remains a valuable asset, supporting effective decision-making and operational efficiency. These standards will be periodically reviewed and updated to reflect evolving organizational needs and industry best practices.
8. Appendix (Optional)
- Appendix A: Glossary of Data Quality Terms
- Appendix B: Data Quality Monitoring Tools Overview
- Appendix C: Sample Data Quality Report Template
This template should be adjusted to reflect specific organizational needs and can be used as a foundational document for communicating and implementing data quality standards across the organization. Would you like help with further customization of this document, or creating tools such as training materials or reports?
Leave a Reply
You must be logged in to post a comment.