Your cart is currently empty!
SayPro Baseline Data: Current data quality levels across various projects.
SayPro is a Global Solutions Provider working with Individuals, Governments, Corporate Businesses, Municipalities, International Institutions. SayPro works across various Industries, Sectors providing wide range of solutions.
Email: info@saypro.online Call/WhatsApp: + 27 84 313 7407
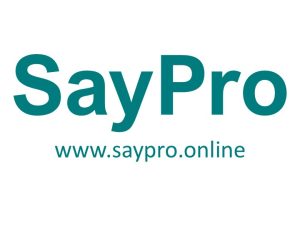
To effectively assess and improve data quality, it’s important to establish a baseline of the current data quality levels across various projects. This baseline provides a starting point for comparison and helps identify areas for improvement. Below is a structure for capturing baseline data across multiple projects, focusing on key data quality dimensions.
SayPro Baseline Data Report
Objective:
To gather and document the current data quality levels across various SayPro projects, providing a snapshot of where data quality stands today and serving as a reference for future improvements.
1. General Information
Project Name | _________________________ |
---|---|
Data Quality Baseline Report Date | _________________________ |
Evaluator(s) | _________________________ |
Department/Team | _________________________ |
Data Quality Dimensions Assessed | Accuracy, Completeness, Consistency, Timeliness, Reliability, Validity |
2. Data Quality Dimensions
2.1 Accuracy
Definition: Accuracy refers to how closely the data represents the real-world conditions it is meant to model.
Project | Accuracy (%) | Issues Identified | Improvement Plan |
---|---|---|---|
Project A | 94% | Minor errors in customer details | Regular cross-checks with trusted sources. |
Project B | 97% | Data entry discrepancies | Implement automated validation checks. |
Project C | 90% | Incorrect product codes | Improve data entry training. |
2.2 Completeness
Definition: Completeness measures whether all required data is collected, with no missing values for critical fields.
Project | Completeness (%) | Issues Identified | Improvement Plan |
---|---|---|---|
Project A | 98% | Missing address data for some clients | Conduct regular audits and improve data entry protocols. |
Project B | 95% | Incomplete feedback forms | Enhance form design to ensure full data capture. |
Project C | 92% | Missing sales figures in reports | Automate data entry and establish reporting guidelines. |
2.3 Consistency
Definition: Consistency refers to whether data is uniform across all systems and departments, without contradictions.
Project | Consistency (%) | Issues Identified | Improvement Plan |
---|---|---|---|
Project A | 96% | Different naming conventions | Standardize naming conventions across platforms. |
Project B | 89% | Discrepancies in date formats | Implement data validation rules for dates. |
Project C | 98% | None | Continue monitoring for consistency. |
2.4 Timeliness
Definition: Timeliness assesses whether data is entered and processed promptly, according to predefined deadlines or real-time requirements.
Project | Timeliness (%) | Issues Identified | Improvement Plan |
---|---|---|---|
Project A | 85% | Data delayed by 2-3 days | Establish stricter timelines for data entry. |
Project B | 90% | Periodic delays in quarterly reports | Automate reporting process. |
Project C | 95% | Some delayed product updates | Implement real-time data synchronization. |
2.5 Reliability
Definition: Reliability is the degree to which data is dependable and consistent over time, with minimal changes or discrepancies in repeated measurements.
Project | Reliability (%) | Issues Identified | Improvement Plan |
---|---|---|---|
Project A | 97% | Occasionally missing data points | Implement redundant data collection checks. |
Project B | 98% | No major issues | Continue monitoring. |
Project C | 93% | Frequent changes to data structure | Stabilize data sources and update standards. |
2.6 Validity
Definition: Validity measures whether the data conforms to expected formats, constraints, and business rules, ensuring it is appropriate for its intended purpose.
Project | Validity (%) | Issues Identified | Improvement Plan |
---|---|---|---|
Project A | 99% | Validity checks on product IDs | Reinforce product ID validation rules. |
Project B | 96% | Some data entries outside expected ranges | Establish range-checking validation rules. |
Project C | 94% | Occasionally invalid timestamps | Update validation rules for time fields. |
3. Overall Data Quality Summary
Project Name | Accuracy (%) | Completeness (%) | Consistency (%) | Timeliness (%) | Reliability (%) | Validity (%) | Overall Data Quality (%) |
---|---|---|---|---|---|---|---|
Project A | 94% | 98% | 96% | 85% | 97% | 99% | 94.8% |
Project B | 97% | 95% | 89% | 90% | 98% | 96% | 94.8% |
Project C | 90% | 92% | 98% | 95% | 93% | 94% | 92.0% |
4. Key Observations
- Project A: Strong accuracy and completeness, but there are timeliness issues that need to be addressed. Improving the speed of data entry and processing would significantly boost overall data quality.
- Project B: High accuracy and validity but lower consistency and timeliness. Addressing the date format discrepancies and improving automation in reporting would improve the overall performance.
- Project C: Reliable consistency but lower accuracy and validity. The issues with product codes and data structure changes should be resolved to improve the accuracy and reliability of data.
5. Improvement Actions
Project Name | Improvement Areas | Recommended Actions |
---|---|---|
Project A | Timeliness and Completeness | Streamline data entry processes, conduct regular data audits, and set strict deadlines for data collection. |
Project B | Consistency and Timeliness | Standardize date formats, automate reporting processes, and ensure alignment across systems. |
Project C | Accuracy and Validity | Enhance training for data entry personnel, reinforce validation rules, and ensure product codes are standardized. |
6. Conclusion & Next Steps
The baseline data quality report shows that each project has different strengths and areas needing improvement. Key actions should focus on improving timeliness for Project A, consistency for Project B, and accuracy for Project C.
By addressing these improvement areas, SayPro can continue to enhance its data quality, providing more reliable and actionable insights.
7. Follow-Up Actions
Action | Assigned To | Due Date | Status |
---|---|---|---|
Implement automated data validation checks (Project A) | [Name/Role] | [Date] | ☐ Pending ☐ In Progress ☐ Completed |
Standardize data formats and automate reporting (Project B) | [Name/Role] | [Date] | ☐ Pending ☐ In Progress ☐ Completed |
Train staff on correct product codes (Project C) | [Name/Role] | [Date] | ☐ Pending ☐ In Progress ☐ Completed |
This Baseline Data Quality Report will provide a comprehensive starting point for ongoing monitoring and improvement initiatives. By regularly updating this report and tracking improvements, SayPro can continue to ensure high-quality data across all projects.
Let me know if you’d like help with further analysis or setting up a system for continuous tracking!
Leave a Reply
You must be logged in to post a comment.