Your cart is currently empty!
SayPro Target Benchmarks: Specific, measurable goals for each data quality standard.
SayPro is a Global Solutions Provider working with Individuals, Governments, Corporate Businesses, Municipalities, International Institutions. SayPro works across various Industries, Sectors providing wide range of solutions.
Email: info@saypro.online Call/WhatsApp: + 27 84 313 7407
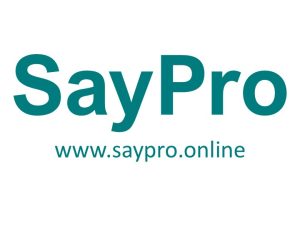
SayPro Target Benchmarks for Data Quality Standards
Objective:
The Target Benchmarks define specific, measurable goals for each data quality dimension, ensuring that SayPro’s data meets the required standards for accuracy, completeness, consistency, timeliness, reliability, and validity. These benchmarks act as clear targets for continuous data quality improvement.
1. Data Quality Dimensions and Benchmarks
1.1 Accuracy
Definition: Accuracy refers to how closely the data represents the real-world conditions it is intended to model. Accurate data reflects the actual situation or entity as intended.
Benchmark Goal | Target Percentage | Measurement Method |
---|---|---|
Accuracy of key data entries (e.g., customer names, addresses, product codes) | 98% or higher | Periodic audits and cross-checking with trusted external data sources. |
Accuracy of automated data entries | 99% or higher | Automated data entry validation checks. |
Error rate in manual data entry | Below 2% | Random spot-checks, system logs, and error-tracking reports. |
1.2 Completeness
Definition: Completeness measures whether all required data has been captured, with no missing values for critical fields.
Benchmark Goal | Target Percentage | Measurement Method |
---|---|---|
Complete data fields for all required information | 100% | Data entry forms with mandatory fields, monitored by automated completeness checks. |
Missing data rate in critical fields (e.g., customer contact details, sales data) | Below 2% | Periodic audits and automated data completeness checks. |
Percentage of datasets with no missing critical information | 100% | Data validation checks integrated into the data collection system. |
1.3 Consistency
Definition: Consistency ensures that data is uniform across systems, processes, and departments, with no contradictions or discrepancies.
Benchmark Goal | Target Percentage | Measurement Method |
---|---|---|
Consistency across systems (e.g., CRM, ERP, databases) | 99% or higher | Cross-system reconciliation checks and automated consistency checks. |
Consistency of standardized data formats (e.g., date format, currency) | 100% | Standardized input formats enforced by system rules. |
Cross-departmental data consistency | 98% or higher | Data comparison between different departments and regular audits. |
1.4 Timeliness
Definition: Timeliness measures whether data is entered and processed within the required timeframes to support effective decision-making.
Benchmark Goal | Target Percentage | Measurement Method |
---|---|---|
Data entered within the required timeframe (e.g., within 24 hours) | 95% or higher | System alerts, automated data entry deadlines, and tracking tools. |
Timely updates to critical datasets (e.g., sales, inventory) | 98% or higher | Real-time data syncing and tracking of update timestamps. |
Reporting deadlines met (e.g., monthly/quarterly reports) | 100% | Automated scheduling and reporting systems. |
1.5 Reliability
Definition: Reliability ensures that data is dependable, stable over time, and free from frequent discrepancies or errors.
Benchmark Goal | Target Percentage | Measurement Method |
---|---|---|
Data stability across time (e.g., historical trends remain consistent) | 98% or higher | Historical data trend analysis and variance checks. |
Frequency of data updates (e.g., periodic updates for ongoing projects) | 100% | Tracking system to ensure data updates occur as scheduled. |
Data accuracy under repeated measurements or entries | 98% or higher | Repeated data tests and comparison of multiple data entries for the same entity. |
1.6 Validity
Definition: Validity measures whether the data conforms to expected formats, constraints, and business rules.
Benchmark Goal | Target Percentage | Measurement Method |
---|---|---|
Data validity (e.g., correctly formatted email addresses, dates) | 99% or higher | Real-time validation rules and format checks during data entry. |
Data validation against business rules (e.g., no negative sales numbers) | 100% | Automated validation and error-checking during data input. |
Percentage of invalid data points identified and corrected within 24 hours | 98% or higher | Automated error logs, error reporting, and correction protocols. |
2. Summary of Target Benchmarks
Data Quality Dimension | Target Benchmark Goal | Target Percentage |
---|---|---|
Accuracy | Accuracy of key data entries | 98% or higher |
Accuracy of automated data entries | 99% or higher | |
Error rate in manual data entry | Below 2% | |
Completeness | Complete data fields for required info | 100% |
Missing data rate in critical fields | Below 2% | |
Datasets with no missing critical info | 100% | |
Consistency | Consistency across systems | 99% or higher |
Consistency of standardized data formats | 100% | |
Cross-departmental data consistency | 98% or higher | |
Timeliness | Data entered within required timeframes | 95% or higher |
Timely updates to critical datasets | 98% or higher | |
Reporting deadlines met | 100% | |
Reliability | Data stability across time | 98% or higher |
Frequency of data updates | 100% | |
Data accuracy under repeated measurements | 98% or higher | |
Validity | Data validity | 99% or higher |
Data validation against business rules | 100% | |
Invalid data points identified and corrected | 98% or higher |
3. Monitoring and Evaluation
Regular monitoring of data quality against these benchmarks is essential for continuous improvement. Key actions include:
- Quarterly Audits: Conducting regular audits to ensure the targets are being met.
- Real-Time Monitoring: Using automated tools to monitor data quality in real time and flag deviations.
- Feedback Loops: Ensuring that data quality issues identified are documented and actioned promptly to prevent future occurrences.
4. Next Steps
- Implement Benchmarks: Introduce these benchmarks into the data governance processes across all relevant teams.
- Set Up Automated Tracking: Utilize tools to automate data quality monitoring and alert teams when thresholds are exceeded.
- Review and Adjust: Annually review benchmarks and adjust based on evolving business needs or new data quality insights.
These Target Benchmarks provide specific, measurable goals to guide SayPro’s data quality improvement initiatives. They ensure that all teams align with the same standards and that data quality is consistently monitored and improved over time.
Let me know if you’d like any further customization or need assistance in setting up tracking systems for these benchmarks!
Leave a Reply
You must be logged in to post a comment.