Your cart is currently empty!
Author: Mapaseka Matabane
SayPro is a Global Solutions Provider working with Individuals, Governments, Corporate Businesses, Municipalities, International Institutions. SayPro works across various Industries, Sectors providing wide range of solutions.
Email: info@saypro.online Call/WhatsApp: Use Chat Button ๐
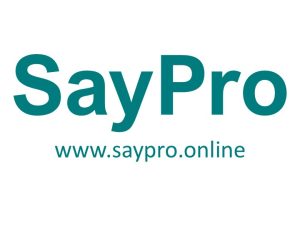
-
SayPro Research Communication Plan
Objectives
- Simplify and translate technical research into digestible content for non-expert audiences.
- Enhance stakeholder engagement by providing timely, relevant insights.
- Strengthen SayProโs visibility and credibility as a research-driven organization.
- Support evidence-based planning and development within SayPro and its partners.
Key Activities
- Development of Communication Materials
- Executive summaries
- Infographics and visual data
- Policy briefs
- Social media content
- Presentation Preparation
- Monthly slide decks for internal/external use
- Talking points for leadership
- Webinars or briefings as needed
- Internal Coordination
- Collaborate with SayPro departments (Research, Policy, Comms, Marketing)
- Ensure consistency in messaging and data interpretation
Key Messages
- SayPro research directly supports economic and social development.
- Data-driven insights promote inclusive growth and community empowerment.
- Accessible research leads to better policy and implementation outcomes.
Target Audiences
- Internal: SayPro leadership, program teams, and regional offices
- External: Policymakers, donors, NGOs, community leaders, media, academic partners
Communication Channels
- SayPro Website โ Dedicated monthly research section
- Email Newsletters โ Highlight summaries and updates
- Social Media โ Bite-sized insights and infographics
- Stakeholder Briefings โ Monthly Zoom or in-person sessions
- Press Releases and Media Kits โ For broader outreach
Timeline
- Week 1โ2: Research summary and message drafting
- Week 3: Coordination and design of materials
- Week 4: Dissemination and presentation
- Ongoing: Feedback collection and content refinement
Monitoring & Evaluation
- Engagement metrics (website views, social media reach, newsletter opens)
- Stakeholder feedback
- Usage of research in decision-making or program adjustments
-
SayPro Implement Monitoring Framework
Define Objectives and Goals
Before creating the monitoring framework, clearly define the objectives of the cost management strategies. These objectives will serve as the basis for monitoring progress and evaluating success.
Example Objectives:
- Reduce operational costs by 15% over the next 12 months.
- Improve procurement efficiency by decreasing lead times by 20%.
- Enhance resource allocation to achieve a 10% increase in ROI.
2. Identify Key Performance Indicators (KPIs)
The next step is to determine which KPIs will help measure whether the strategies are achieving their goals. These indicators should be specific, measurable, attainable, relevant, and time-bound (SMART).
Potential KPIs for Monitoring Cost Management Strategies:
- Cost Reduction
- Total Operational Cost Savings: Measure the actual savings in operational costs after implementing strategies like staffing optimization or procurement efficiency.
- Unit Cost Reduction: Monitor changes in the cost per unit produced or service delivered (useful for businesses with tangible products or services).
- Procurement and Budgeting Efficiency
- Procurement Cost Savings: Track cost savings achieved through better supplier negotiations or bulk purchasing.
- Procurement Cycle Time: Measure the time it takes to complete procurement activities (e.g., from order placement to delivery), aiming for reduced lead times.
- Resource Utilization
- Resource Utilization Rate: Measure the percentage of available resources (e.g., labor, equipment, capital) being used effectively.
- Inventory Turnover Rate: Track how often inventory is sold and replaced over a period, indicating effective stock management and cost control.
- Operational Efficiency
- Productivity Rates: Measure the output per labor hour, assessing whether the changes are improving efficiency.
- Waste Reduction: Track reductions in material waste or inefficiencies due to improved processes.
- Return on Investment (ROI)
- Cost Savings vs. Investment: Measure the ROI of the cost-reduction strategies by comparing the savings to the costs associated with implementing the strategies.
3. Develop a Timeline for Monitoring
Define a clear timeline for how frequently you will track and evaluate these KPIs. Monitoring should occur at regular intervals to ensure that issues are addressed promptly and adjustments are made if necessary.
Suggested Timeline for Monitoring:
- Monthly: Initial performance check to track immediate impacts (e.g., procurement cycle time, cost savings, resource utilization).
- Quarterly: More in-depth evaluation to analyze trends over a longer period (e.g., total operational savings, ROI).
- Annually: Comprehensive assessment to measure the long-term effectiveness of the strategies and make adjustments as necessary.
4. Define Roles and Responsibilities
Designate specific individuals or teams responsible for monitoring the performance of each KPI. Ensure they understand their role in tracking progress, collecting data, and reporting findings.
Example Roles and Responsibilities:
- Finance Team: Responsible for tracking cost savings and ROI metrics, ensuring that budget reports reflect the impact of the strategies.
- Procurement Manager: Monitors procurement cycle time and cost savings from improved supplier negotiations.
- Operations Team: Tracks resource utilization and waste reduction, ensuring operational processes are optimized.
- Project Manager: Oversees the overall implementation of cost-reduction strategies, ensuring alignment with organizational goals and timely adjustments based on performance.
5. Data Collection and Reporting Mechanisms
Establish processes for collecting data on the defined KPIs and how that data will be reported. This should include:
- Data Sources: Identify where data will be collected from (e.g., financial reports, procurement records, employee time tracking).
- Tools and Systems: Use digital tools (e.g., dashboards, project management software) to track and visualize progress. Tools like Power BI, Tableau, or Google Sheets can help monitor and visualize key metrics.
- Reporting Frequency: Define how often reports will be generated for internal stakeholders (e.g., monthly progress reports for leadership).
Example Reporting Framework:
- Monthly Progress Report: A summary of key metrics and a comparison against targets.
- Quarterly Review: A detailed report on overall performance, including trends and insights.
- Annual Report: A comprehensive evaluation of all implemented cost-management strategies, summarizing the impact on cost savings, operational efficiency, and ROI.
6. Continuous Improvement and Adjustments
The monitoring framework should include mechanisms for continuous improvement. If the data shows that the strategies are not achieving the desired outcomes, adjustments should be made.
Strategies for Continuous Improvement:
- Regular Review Meetings: Host quarterly meetings with relevant stakeholders to review performance against KPIs and decide on adjustments if necessary.
- Feedback Loops: Encourage team members, employees, and other stakeholders to provide feedback on the implementation process and outcomes.
- Adjustments: Based on the performance data and feedback, propose changes to the strategies. For example, if procurement savings arenโt meeting targets, consider renegotiating contracts or exploring additional suppliers.
7. Evaluation and Reporting
Once the monitoring framework is in place, it’s essential to periodically evaluate the overall impact of the cost management strategies and report findings back to stakeholders.
Key Components of Evaluation:
- Effectiveness: Assess whether the cost management strategies have achieved their intended goals (e.g., reduced costs, improved ROI).
- Efficiency: Determine if the strategies have led to more efficient resource use and operational processes.
- Sustainability: Evaluate whether the cost reductions and efficiencies are sustainable in the long term.
Final Evaluation Report Components:
- Summary of KPIs: Overview of each KPI, whether targets were met, and the reason for any variances.
- Recommendations for Refinement: Based on monitoring data, provide suggestions for refining strategies or adjusting implementation plans.
- Long-term Impact: Assess whether the strategies have had a positive long-term impact on financial health, operational performance, and organizational sustainability.
8. Example Monitoring Framework Template
Key Performance Indicator (KPI) Target Data Source Frequency of Monitoring Responsible Party Monitoring Tools Total Operational Cost Savings 15% reduction Financial reports Monthly, Quarterly Finance Team Power BI, Google Sheets Procurement Cycle Time 20% reduction Procurement records Monthly, Quarterly Procurement Manager ERP system, Excel Resource Utilization Rate 90% utilization Operations data Monthly, Quarterly Operations Team Custom dashboard Productivity Rate 10% increase Employee timesheets Monthly, Quarterly HR Team Time tracking software Waste Reduction 10% decrease Production records Monthly, Quarterly Operations Team Manufacturing software Return on Investment (ROI) 10% increase Financial analysis Quarterly, Annually Finance Team Financial software -
SayPro Prepare Reports and Recommendations
Executive Summary
Provide a concise overview of the reportโs key findings, objectives, and recommendations. The executive summary should be easy to digest for busy stakeholders, such as business leaders or policymakers.
Components:
- Purpose of the Research: Briefly explain the purpose of the research (e.g., improving cost management in targeted sectors).
- Key Findings: Summarize the most important insights gathered from the analysis, such as inefficiencies, areas of potential cost reduction, and best practices.
- Core Recommendations: Highlight the top recommendations for cost reduction and efficiency improvement.
2. Introduction
Set the context for the report by explaining the background, objectives, and scope of the research.
Components:
- Background: Why is cost management a priority, and how does it relate to current economic, industry, and policy challenges?
- Research Objectives: Clearly state the objectives of the research, such as identifying inefficiencies in cost management and suggesting strategies for improvement.
- Scope: Define which sectors or areas the research covered (e.g., business operations, government programs, community initiatives).
3. Methodology
Detail the methods used to gather data and analyze cost management practices across the targeted sectors.
Components:
- Data Collection: Explain how data was gathered (e.g., surveys, interviews, case studies, financial reports).
- Analysis Techniques: Describe the tools and frameworks used to analyze the data (e.g., cost-benefit analysis, ROI calculations, industry benchmarking).
- Stakeholder Input: Mention any consultations or workshops held with stakeholders (business leaders, financial experts, government officials).
4. Findings and Analysis
Present the main findings from the research. This section should be organized into key themes or areas, with data to support the conclusions.
Components:
- Current Cost Management Practices: Describe existing cost structures and management approaches across the targeted sectors.
- Inefficiencies and Cost Overruns: Identify areas where costs are high or management practices are inefficient. Include data to support these claims (e.g., high procurement costs, inefficient energy use, underutilized resources).
- Economic Impacts: Discuss how factors like inflation, global supply chain issues, or changes in consumer behavior have influenced cost structures.
- Best Practices: Highlight successful strategies or industry benchmarks that can be adapted to improve cost management.
5. Recommendations for Cost Management Improvements
Provide actionable recommendations to address the identified inefficiencies. These should be practical and tailored to the specific needs of the sectors involved.
Components:
- Cost Reduction Strategies: Propose strategies to cut costs without sacrificing service quality. For example, โOptimize staffing by implementing flexible work arrangements and cross-training employees.โ
- Budgeting and Procurement Improvements: Recommend ways to improve budgeting accuracy and reduce waste in procurement processes (e.g., adopting more competitive bidding processes, bulk purchasing).
- Technological Innovations: Suggest the integration of technology for automation and process improvement (e.g., implementing software for real-time tracking of expenses, using AI for supply chain optimization).
- Resource Allocation Strategies: Recommend strategies for reallocating resources more effectively to maximize ROI (e.g., reducing overhead costs by outsourcing non-core activities).
- Staff Training and Development: Suggest training programs to upskill employees in cost-conscious decision-making.
6. Implementation Plan
Outline a clear, step-by-step plan for implementing the recommended strategies. This section should include timelines, responsible parties, and necessary resources.
Components:
- Timeline: Provide an estimated timeline for implementing each recommendation. For example, “Procurement process overhaul to be completed within 3 months.”
- Responsible Parties: Identify who will be responsible for each action (e.g., department heads, financial officers).
- Resources Needed: Specify any resources required for implementation, such as new software, training programs, or additional personnel.
- Performance Metrics: Define how the success of each strategy will be measured (e.g., cost savings, improved ROI, efficiency gains).
7. Monitoring and Evaluation Framework
Propose a system for tracking the effectiveness of the implemented strategies over time.
Components:
- Key Performance Indicators (KPIs): Identify KPIs to monitor progress, such as cost savings achieved, reduction in operational inefficiencies, or improvements in ROI.
- Review Schedule: Set a schedule for regular progress reviews (e.g., quarterly check-ins).
- Adjustment Mechanisms: Include suggestions for how to refine or adjust strategies if they are not yielding the desired results.
8. Conclusion
Summarize the overall findings and reiterate the importance of the recommendations for achieving long-term cost-effectiveness.
9. Appendices (if applicable)
Include any additional materials, such as detailed data tables, charts, interview summaries, or survey results, that support the analysis and recommendations.
Example Structure for the Report:
SayPro Monthly April SCRR-14: SayPro Monthly Research Cost Management
- Executive Summary
- Key findings
- Core recommendations
- Introduction
- Background of the research
- Research objectives and scope
- Methodology
- Data collection methods
- Analytical techniques
- Findings and Analysis
- Current cost management practices
- Identified inefficiencies and cost-overrun areas
- Economic impact on cost structures
- Industry best practices
- Recommendations for Cost Management Improvements
- Cost-reduction strategies
- Budgeting and procurement improvements
- Technological innovations
- Resource allocation strategies
- Staff training and development
- Implementation Plan
- Detailed action steps, timeline, and responsible parties
- Monitoring and Evaluation Framework
- KPIs, review schedule, and adjustment mechanisms
- Conclusion
- Summary of findings and importance of recommendations
- Appendices
- Supporting data, charts, or surveys
-
SayPro Consult Stakeholders
Define Stakeholder Groups
Start by identifying the different stakeholder groups whose input will be critical to the development of cost-reduction strategies. Common stakeholder groups include:
- Business Leaders and Executives: They are responsible for strategic decision-making and will have insights into organizational goals and constraints.
- Department Heads and Managers: They are directly involved in day-to-day operations and can provide practical feedback on cost-saving measures.
- Financial Experts or CFOs: They can assess the feasibility of proposed strategies from a financial perspective.
- Government and Policy Makers: If relevant, their perspective is crucial in understanding regulatory requirements and public funding impacts.
- Employees: Gathering input from the workforce can help identify areas for cost reduction that may not be immediately obvious to leadership.
2. Plan and Structure the Consultation Sessions
For effective stakeholder engagement, plan the workshops or meetings with clear objectives and an organized structure:
- Objective: The primary goal is to gather input on cost-reduction strategies, ensuring they are feasible, acceptable, and aligned with organizational goals.
- Agenda:
- Introduction: Explain the purpose of the session and provide context for the proposed cost-reduction strategies.
- Presentation of Findings: Share preliminary findings from the cost analysis, including identified inefficiencies and proposed cost-reduction strategies.
- Feedback Collection: Use different methods to collect feedback (e.g., surveys, group discussions, open Q&A).
- Group Work (Optional): Break attendees into smaller groups to brainstorm additional ideas or discuss the feasibility of proposed strategies.
- Summary and Next Steps: Summarize key takeaways, outline the next steps in the consultation process, and thank participants for their input.
- Format: Choose the most suitable format depending on the stakeholder group and the available time. Consider virtual meetings, in-person workshops, or hybrid formats.
3. Tools and Techniques for Gathering Input
- Surveys and Questionnaires: Distribute surveys prior to or after the session to gather specific feedback on cost-reduction strategies. Keep the surveys focused on key areas like cost-saving priorities, challenges with implementation, or areas of concern.
- Facilitated Group Discussions: Allow stakeholders to share thoughts in a facilitated discussion, ensuring all voices are heard. You can use tools like SWOT analysis (Strengths, Weaknesses, Opportunities, Threats) to structure these discussions.
- Interactive Voting or Polling: Use live polling tools (like Mentimeter or Slido) to gauge stakeholder opinions on key strategies in real-time.
- Workshops/Breakout Sessions: Break stakeholders into smaller groups to work on specific cost-reduction topics. For example, one group can focus on staffing optimization, while another looks at energy efficiency.
- Feedback Forms: After the session, send out detailed feedback forms where participants can provide more thoughtful, individual responses.
4. Analyze and Prioritize Stakeholder Feedback
Once you have gathered the input, analyze the feedback to identify:
- Common Themes: Look for repeated suggestions or concerns that need to be addressed in the final cost-reduction strategies.
- Feasibility and Impact: Consider the feasibility of implementing the suggested strategies, as well as their potential impact on cost savings, service quality, and long-term sustainability.
- Stakeholder Buy-in: Note which strategies received the strongest support and which encountered resistance. This will help you prioritize and refine your approach.
5. Communicate the Next Steps
After consulting stakeholders, ensure they are kept informed about how their input will be integrated into the cost-reduction strategies. Communicate the following:
- Thank You: Express appreciation for their time and insights.
- Summary of Feedback: Provide a summary of the feedback received and how it will influence the final cost-reduction strategies.
- Implementation Timeline: Outline the next steps, including how and when the strategies will be implemented, and any further consultations that may occur.
- Opportunities for Ongoing Engagement: If relevant, offer opportunities for stakeholders to remain involved in monitoring the impact of the strategies.
6. Example Stakeholder Consultation Agenda
Hereโs an example agenda for a stakeholder consultation session:
Stakeholder Consultation Session Agenda: Cost-Reduction Strategies
Date: [Insert Date]
Time: [Insert Time]
Location: [Insert Location or Virtual Platform]
- Welcome & Introduction (10 minutes)
- Brief introduction of the purpose of the session
- Overview of the cost-reduction strategies
- Presentation of Preliminary Findings (20 minutes)
- Overview of the current cost structure and identified inefficiencies
- Proposed strategies for cost reduction
- Group Discussion (30 minutes)
- Open discussion on proposed strategies, including potential challenges, benefits, and feasibility
- Facilitated by a moderator, focusing on specific themes (e.g., staffing, procurement, energy efficiency)
- Breakout Sessions (30 minutes)
- Break into smaller groups to discuss specific areas (e.g., staffing optimization, procurement strategies)
- Groups present their ideas to the larger group
- Interactive Polling & Q&A (20 minutes)
- Live poll on key strategies
- Open floor for questions and feedback
- Summary & Next Steps (10 minutes)
- Summarize the key takeaways from the session
- Outline the next steps in refining the cost-reduction strategies
- Provide contact details for any follow-up questions
7. Post-Consultation
After the stakeholder consultation, ensure that the information collected is integrated into the final strategy report. Additionally:
- Update the proposed strategies based on the feedback received.
- Ensure stakeholders are notified of changes or refinements made as a result of the consultation.
- Continue the dialogue to maintain engagement throughout the implementation phase.
-
SayPro Develop Cost-Reduction Strategies
Assess Current Cost Structures
Before proposing strategies, itโs crucial to have a detailed understanding of the existing cost structure. Start by:
- Analyzing Current Expenses: Review financial statements, cost reports, and budgets to identify the major areas of spending.
- Categorizing Costs: Separate costs into fixed (e.g., rent, salaries) and variable (e.g., production materials, commissions). This will help prioritize areas to focus on.
- Identifying High-Cost Areas: Pinpoint departments, processes, or services that are consuming the most resources.
2. Short-Term Cost-Reduction Strategies
These are quick wins that can help save money without affecting service quality or business operations:
A. Negotiate with Suppliers
- Strategy: Revisit supplier contracts to negotiate better terms or seek alternative suppliers for more competitive prices.
- Action: Compare prices, volume discounts, and delivery terms to reduce costs on raw materials, utilities, or services.
B. Optimize Staffing and Labor Costs
- Strategy: Review staffing levels and payroll to identify areas where labor costs can be reduced.
- Action: Implement flexible work arrangements, cross-train employees for multiple roles, or adjust working hours to reduce overtime costs.
C. Reduce Energy and Utility Costs
- Strategy: Focus on energy-saving initiatives to reduce electricity, water, and heating costs.
- Action: Invest in energy-efficient lighting, HVAC systems, and machinery, as well as encourage employee awareness of reducing waste.
D. Eliminate Non-Essential Expenditures
- Strategy: Cut out or reduce unnecessary expenses that donโt directly contribute to productivity or growth.
- Action: Audit discretionary spending on office supplies, subscriptions, or services, and eliminate or reduce those that are not critical.
3. Medium-Term Cost-Reduction Strategies
These strategies involve more detailed analysis and may take time to implement, but they will have a lasting impact.
A. Process Optimization and Efficiency Improvements
- Strategy: Streamline internal processes to eliminate bottlenecks and inefficiencies.
- Action: Implement Lean or Six Sigma methodologies to analyze workflows, reduce waste, and improve efficiency in production, administration, or customer service.
B. Outsourcing Non-Core Activities
- Strategy: Outsource functions that are not central to the organizationโs core business (e.g., IT, HR, or customer support).
- Action: Contract third-party vendors for functions such as payroll processing, IT management, or logistics, which can provide specialized expertise at lower costs.
C. Invest in Technology and Automation
- Strategy: Implement technology solutions to automate repetitive tasks and improve productivity.
- Action: Invest in software, AI tools, and automation that can handle administrative tasks like invoicing, inventory management, or customer inquiries.
D. Consolidate Vendor Relationships
- Strategy: Reduce the number of vendors and negotiate bulk contracts to receive volume discounts.
- Action: Evaluate current vendor contracts and consolidate purchases to fewer suppliers, securing better pricing and reducing procurement complexity.
4. Long-Term Cost-Reduction Strategies
These strategies are strategic and aimed at ensuring the organizationโs long-term financial health while maintaining quality and operational capacity.
A. Redesign the Business Model for Efficiency
- Strategy: Evaluate and adjust the overall business model to reduce overhead and increase scalability.
- Action: If feasible, consider shifting from a traditional brick-and-mortar setup to an online platform, reducing rent and utilities, or transitioning to a more agile workforce.
B. Invest in Sustainability and Green Technologies
- Strategy: Implement sustainable practices that lead to long-term cost savings.
- Action: Invest in renewable energy sources (solar panels, wind power), reduce waste, and optimize transportation logistics to lower long-term environmental costs.
C. Improve Financial Forecasting and Budgeting
- Strategy: Strengthen financial planning and monitoring to identify inefficiencies in the early stages.
- Action: Use advanced financial forecasting tools to predict cash flow, identify potential cost overruns, and reallocate resources before issues arise.
D. Foster a Culture of Continuous Improvement
- Strategy: Build a culture of cost consciousness and efficiency across all levels of the organization.
- Action: Provide regular training on cost-effective practices, encourage employee participation in cost-reduction ideas, and implement continuous improvement (Kaizen) initiatives.
5. Implement and Monitor Cost-Reduction Strategies
Once youโve designed the strategies, itโs important to implement them effectively and track their progress:
A. Set Clear Goals and KPIs
- Define specific, measurable targets for cost reduction (e.g., 10% reduction in energy costs, 5% reduction in supply chain expenses).
- Monitor key performance indicators (KPIs) like cost per unit, operating margins, supply chain costs, and labor efficiency.
B. Prioritize Strategies
- Prioritize cost-reduction strategies based on potential savings, ease of implementation, and alignment with strategic objectives.
- Tackle high-impact, low-cost changes first to demonstrate quick results and build momentum for larger initiatives.
C. Continuous Monitoring and Feedback
- Establish a monitoring framework to regularly assess the impact of cost-reduction efforts.
- Adjust strategies based on feedback, market changes, or performance data.
6. Example Cost-Reduction Strategy Proposal
Hereโs an example of how a proposed cost-reduction strategy might look:
Objective: Reduce Operational Costs by 15% in the Next 12 Months
Proposed Strategies:
- Staffing Optimization:
- Action: Conduct a staffing audit and implement a cross-training program for employees.
- Expected Savings: 5% reduction in overtime and temporary staffing costs.
- Energy Efficiency:
- Action: Install energy-efficient LED lighting and smart thermostats across facilities.
- Expected Savings: 3% reduction in electricity costs.
- Vendor Consolidation:
- Action: Negotiate bulk contracts with key suppliers for discounts.
- Expected Savings: 4% reduction in procurement costs.
- Technology Integration:
- Action: Implement a customer relationship management (CRM) system to automate administrative tasks.
- Expected Savings: 3% reduction in administrative labor costs.
Timeline:
- Quarter 1: Staffing audit and vendor negotiations.
- Quarter 2: Energy efficiency upgrades and CRM implementation.
- Quarter 3 & 4: Ongoing monitoring and optimization.
Key Performance Indicators:
- Reduction in energy costs (kWh).
- Reduction in staffing overtime and temporary labor.
- Improved procurement cost-efficiency.
- Increased automation in administrative tasks.
-
SayPro Research Best Practices
Define the Scope of Best Practices
Identify the industries or sectors that are most relevant for your research, such as:
- Manufacturing: Best practices for reducing production costs, optimizing supply chains, and improving operational efficiency.
- Healthcare: Practices that focus on cost reduction without compromising patient care, optimizing labor, and managing resource allocation.
- Government/Public Sector: Approaches for optimizing public spending, improving budget management, and reducing waste in government programs.
- Non-Profit Sector: Cost-effective strategies for running social programs and community initiatives while maintaining quality service delivery.
2. Conduct Industry Analysis
Study the leading companies and organizations in the identified sectors to understand how they manage costs. Focus on:
- Case Studies: Look for real-world examples where organizations have successfully improved cost management. Key areas to look at include cost-saving initiatives, process optimizations, technology implementations, and financial strategies.
- Industry Reports: Review reports and white papers from industry leaders, consultancy firms (e.g., McKinsey, PwC), and trade associations that highlight best practices in cost management.
- Benchmarking: Compare cost structures and strategies across similar organizations to identify what high-performing companies are doing differently.
3. Key Best Practices in Cost Management
Here are some proven cost management best practices across various industries:
A. Lean Management and Waste Reduction
- Manufacturing Sector:
- Example: Toyotaโs Lean Manufacturing approach focuses on eliminating waste (known as “Muda”) by continuously improving processes and reducing inefficiencies across the production line. This has led to lower production costs, faster production times, and higher quality products.
- Application: Identify non-value-adding activities in your processes and eliminate or streamline them. Implement regular Kaizen (continuous improvement) practices with employee involvement.
B. Strategic Sourcing and Procurement Optimization
- Retail and Consumer Goods:
- Example: Companies like Walmart and Amazon leverage economies of scale by negotiating with suppliers for bulk purchasing and long-term contracts, reducing unit costs.
- Application: Consolidate purchasing to gain volume discounts, improve supplier relationships, and explore global sourcing opportunities for competitive pricing.
C. Automation and Digital Transformation
- Finance and Accounting:
- Example: Intuit used robotic process automation (RPA) to automate repetitive tasks in accounting, reducing human errors and freeing up staff for higher-value work.
- Application: Invest in AI, automation, and digital tools that can replace manual labor and reduce overhead costs. This can be applied in accounting, HR, customer service, and supply chain management.
D. Energy Efficiency and Resource Optimization
- Manufacturing/Building Operations:
- Example: General Electric (GE) adopted energy-efficient technologies and implemented energy audits across its facilities to reduce utility costs. By improving HVAC systems and using renewable energy sources, GE cut operational costs significantly.
- Application: Audit energy consumption regularly and implement energy-efficient solutions (LED lighting, smart systems, renewable energy) to reduce utility costs. Consider retrofitting old equipment for better performance.
E. Activity-Based Costing (ABC)
- Healthcare and Non-Profit Sectors:
- Example: Healthcare organizations like Mayo Clinic have successfully used Activity-Based Costing (ABC) to allocate overhead costs more accurately and identify underperforming areas, leading to better resource allocation and cost control.
- Application: Implement ABC to allocate indirect costs more accurately, allowing you to identify costly services or departments and optimize them.
F. Demand Forecasting and Inventory Optimization
- Supply Chain Management:
- Example: Zara uses sophisticated demand forecasting techniques and maintains a flexible supply chain, enabling the company to keep its inventory lean while quickly responding to consumer preferences. This minimizes overstocking and reduces warehousing costs.
- Application: Use predictive analytics and demand forecasting tools to optimize inventory levels and reduce storage costs. Implement just-in-time inventory systems to prevent overstock and reduce waste.
G. Cross-Functional Collaboration and Communication
- Corporate/Consulting Firms:
- Example: McKinsey & Company uses cross-functional teams to share insights and reduce duplication of effort. By fostering a culture of collaboration across departments, McKinsey can identify areas where processes can be streamlined and costs reduced.
- Application: Encourage interdepartmental collaboration to share resources, eliminate redundancies, and identify opportunities for cost reduction.
H. Outsourcing and Shared Services
- Technology and Services Sectors:
- Example: IBM outsourced certain non-core functions like IT infrastructure management, allowing it to focus on higher-value activities such as research and development. Shared services and outsourcing allowed IBM to reduce overhead while maintaining flexibility.
- Application: Evaluate non-core business functions that can be outsourced to third-party vendors to reduce operational costs. Consider shared services for administrative tasks across departments.
I. Budgeting and Financial Planning Optimization
- Public Sector and Government:
- Example: Singapore employs zero-based budgeting (ZBB), where every dollar of expenditure is justified each year. This helps ensure that funds are allocated efficiently and can prevent unnecessary spending.
- Application: Use zero-based budgeting or activity-based budgeting to ensure every expense aligns with the strategic goals and priorities. Regularly revisit budget assumptions to adapt to changing economic conditions.
J. Agile Cost Management
- Software and Technology:
- Example: Spotify employs an agile methodology that helps the company rapidly respond to market shifts, optimizing development and operational costs. This flexible approach enables it to pivot quickly when necessary.
- Application: Implement agile project management and cost management practices, allowing you to adapt quickly to new business conditions without compromising efficiency.
4. Best Practices Implementation Framework
Once you’ve identified the best practices, document them in a structured framework for easy implementation:
- Prioritize Best Practices: Determine which practices align with your organizationโs strategic goals and budget constraints.
- Action Plans: Develop specific action plans for each practice, with clear steps, timelines, and responsibilities.
- Pilot Testing: Run pilot programs to test the effectiveness of these practices in a controlled environment before scaling up.
- Monitoring & Adjusting: Continuously monitor the effectiveness of these practices and make adjustments as needed to ensure long-term success.
5. Conclusion and Recommendations
- Documentation: Create a Best Practices Report that consolidates all identified best practices, case studies, and strategies for cost management. Include actionable recommendations for how these practices can be applied to your organization or sector.
- Training & Development: Offer training to employees at all levels to ensure these best practices are understood and adopted across the organization.
-
SayPro Evaluate the Economic Landscape
External Economic Factors to Consider
- Inflation: The general increase in prices affects both input and output costs.
- Global Supply Chain Disruptions: Shortages, delays, and rising costs due to disruptions in global trade or local supply chains.
- Changes in Consumer Behavior: Shifting demands for products or services, changes in spending patterns, and preferences.
- Currency Fluctuations: If working in international markets, currency exchange rates can influence the cost of imports and exports.
- Economic Recession/Recovery: Periods of contraction or growth impact consumer spending, business investment, and government spending.
- Technological Advancements: The introduction of new technologies can shift costs, either increasing initial investment or reducing long-term operational costs.
2. Impact of Inflation on Cost Management
- Cost Increases: As inflation drives up the prices of raw materials, energy, and labor, businesses will face rising operational costs.
- Example: A manufacturing company may see higher material costs, increasing production expenses.
- Impact on Profit Margins: As costs rise without a proportional increase in product pricing, profit margins will be squeezed.
- Businesses must consider adjusting pricing strategies or improving efficiency to counteract this.
- Long-term Budgeting: Inflation impacts the accuracy of long-term cost projections, requiring more frequent adjustments and agile financial planning.
Recommendation:
- Regularly adjust pricing models and perform cost assessments to pass on increased costs or find efficiencies elsewhere.
- Implement hedging strategies for essential raw materials or invest in automation to offset labor cost increases.
3. Global Supply Chain Disruptions
- Delayed Deliveries: Disruptions can lead to delays in receiving raw materials or essential goods, causing production bottlenecks or delayed product launches.
- Cost of Logistics: Increased transportation costs, including fuel surcharges, and the need to secure alternative suppliers can drive up costs.
- Inventory Shortages: Supply chain disruptions may lead to inventory shortages, driving up demand and further increasing prices of available materials.
- Geopolitical Instability: Trade barriers, tariffs, or political instability can increase the cost of imports, leading to higher costs for goods and services.
Recommendation:
- Diversify suppliers and build flexibility into the supply chain by sourcing from multiple regions.
- Use advanced supply chain management technologies to predict disruptions and adjust quickly.
- Invest in local or nearshore manufacturing to reduce reliance on global supply chains.
4. Changes in Consumer Behavior
- Shift to Online Shopping: The rise in e-commerce impacts traditional retail models and distribution costs, requiring investment in digital infrastructure and logistics.
- Value Sensitivity: Economic uncertainty often shifts consumer behavior towards lower-cost options, forcing businesses to rethink their pricing and offerings.
- Preference for Sustainability: Consumers are increasingly prioritizing sustainability, which may result in businesses needing to adjust production processes to be more eco-friendly, impacting cost structures.
- Product Demand Shifts: Changes in consumer preferences may result in fluctuating demand, requiring businesses to adjust their product lines and inventory management.
Recommendation:
- Embrace e-commerce and develop efficient digital sales channels to capitalize on the shift in consumer behavior.
- Implement cost-effective sustainability measures without compromising quality, such as energy-efficient production practices.
- Monitor consumer behavior closely to quickly respond to demand shifts, optimizing inventory and marketing strategies.
5. Currency Fluctuations
- Imports and Exports: A weaker local currency increases the cost of importing raw materials and products from abroad, while strengthening the local currency reduces export competitiveness.
- Cost Volatility: Frequent currency fluctuations make it challenging to predict costs for international operations, affecting budgeting and pricing strategies.
Recommendation:
- Consider currency-hedging strategies to mitigate the risks associated with fluctuating exchange rates.
- Focus on developing local supply chains to reduce exposure to foreign currency risk.
6. Economic Recession and Recovery
- Cost Reduction Pressure: During recessions, businesses often face the need to reduce costs to maintain profitability due to decreased demand.
- Increased Competition: Economic downturns can lead to higher competition for shrinking consumer spending, forcing businesses to lower prices or offer more attractive value propositions.
- Public Spending Cuts: Government program funding may be reduced during economic recessions, requiring businesses or non-profits relying on public funding to find alternative revenue sources or reduce service costs.
Recommendation:
- Focus on cost optimization during recessions by adopting lean management practices and focusing on high-priority, high-return activities.
- In recovery phases, leverage opportunities for expansion by investing in innovation and enhancing efficiency in operations.
7. Technological Advancements
- Automation and AI: Technological advancements can reduce labor costs, improve production processes, and enhance supply chain efficiency.
- R&D Investment: Innovations in product development and service delivery can lead to higher upfront costs but result in long-term operational cost savings.
- Cost of Adoption: The initial investment in new technologies can be high, requiring careful analysis of potential ROI.
Recommendation:
- Invest in automation and AI tools that streamline processes, reduce waste, and optimize labor costs.
- Consider incremental technology adoption to spread costs over time and ensure ROI justifies the investment.
8. Overall Impact on Cost Management
- Operational Complexity: External economic factors such as inflation, supply chain disruptions, and changes in consumer behavior add complexity to managing costs effectively.
- Pressure on Profit Margins: Many of these factors put significant pressure on profit margins, requiring businesses to adopt a more agile and responsive cost management strategy.
- Need for Strategic Forecasting: Businesses will need to develop more dynamic and flexible forecasting models that can quickly adjust to changing economic conditions.
9. Actionable Recommendations
- Cost Resilience: Develop and implement cost resilience strategies that allow your organization to navigate economic volatility.
- Diversification: Diversify suppliers, markets, and revenue sources to mitigate risks associated with external economic factors.
- Agility in Budgeting: Implement rolling budgets that allow adjustments based on shifting economic conditions.
- Investment in Technology: Prioritize investments in technology and automation to improve efficiency and reduce reliance on costly manual processes.
-
SayPro Conduct Preliminary Cost Analysis
Define Target Sectors
Identify and define the sectors that will be analyzed. These could include:
- Business Operations
- Government Programs
- Community Initiatives
For each sector, you will want to focus on relevant sub-sectors that will provide the most insight into cost management. For example:
- For business operations, you might look at manufacturing, marketing, and logistics.
- For government programs, focus on public health, education, and infrastructure.
- For community initiatives, focus on non-profit operations, social welfare programs, etc.
2. Gather Existing Financial Data
Collect data related to costs within the identified sectors. This may include:
- Operational costs: Salaries, materials, utilities, etc.
- Capital expenditures: Equipment, infrastructure, technology investments.
- Indirect costs: Overhead, administration, and miscellaneous expenses.
Sources to consider:
- Financial statements
- Budget reports
- Internal cost accounting systems
- Interviews or surveys from key department heads and stakeholders
3. Map Out Cost Categories
Create categories for the costs across the sectors to structure the analysis. Common categories might include:
- Fixed Costs: Costs that remain constant regardless of activity (e.g., rent, utilities).
- Variable Costs: Costs that change with the level of activity (e.g., raw materials, labor hours).
- Semi-variable Costs: Costs that fluctuate within a certain range (e.g., maintenance, operational supplies).
Classifying costs will help in identifying patterns and potential inefficiencies.
4. Identify Key Cost Drivers
Analyze what factors are driving costs in each sector. For example:
- In Business: Cost of goods sold (COGS), marketing and advertising spend, supply chain inefficiencies, technological advancements, etc.
- In Government Programs: Regulatory compliance, staffing levels, technology use, service delivery costs.
- In Community Initiatives: Volunteer hours, fundraising, resource allocation, program overhead.
Understanding what drives costs will give insight into areas of improvement.
5. Perform Benchmarking
Compare the identified costs against industry standards or similar organizations to:
- Identify areas of excessive spending.
- Highlight inefficiencies or underutilized resources.
- Recognize practices where the target sector is excelling.
6. Identify Inefficiencies
Look for areas where costs can be reduced or optimized. Common inefficiencies to look out for include:
- Wasted resources: Overstaffing, underused equipment or facilities.
- Ineffective procurement practices: High supply chain costs or uncompetitive pricing.
- Budget misallocation: Misalignment between expenditure and strategic priorities.
7. Develop Preliminary Findings
Create a summary of key findings from the analysis. This could include:
- High-cost areas: Where the most significant expenditures are happening.
- Opportunity areas: Where costs could be reduced without negatively impacting quality.
- Strategic areas: Where investment is necessary to improve efficiency or return on investment (ROI).
8. Present Preliminary Recommendations
Based on the cost structure analysis, suggest preliminary recommendations for cost optimization. These could include:
- Process improvements: Streamlining workflows, adopting automation.
- Resource reallocation: Reducing spending on underperforming areas and reallocating to high-impact ones.
- Cost-reduction initiatives: Cutting back on unnecessary overhead, renegotiating contracts, or consolidating services.
9. Next Steps
- Further Detailed Analysis: If preliminary findings suggest areas with significant room for improvement, you may want to dig deeper into those areas.
- Stakeholder Feedback: Share the preliminary findings with relevant stakeholders to get feedback and refine strategies.
- Action Plan: Develop a detailed action plan based on the findings and feedback.
Output Example:
- A Preliminary Cost Analysis Report summarizing key findings, cost categories, inefficiencies, and recommendations.
- Dashboards/Graphs for visual representation of cost breakdowns.
-
SayPro Presentation Decks
๐ฆ Slide 1: Title Slide
Title:
SayPro Monthly April SCRR-14: Cost Management ResearchSubtitle:
Empowering Smarter Financial Strategies for Sustainable ImpactPresented by:
SayPro Economic Impact Studies Research Office
[Date]
๐ฆ Slide 2: Introduction
- Purpose of the research initiative
- Overview of the SayPro Cost Management Project
- Relevance to current economic and policy environments
๐ฆ Slide 3: Research Objectives
- Assess current cost management practices
- Identify inefficiencies
- Analyze economic and policy influences on costs
- Recommend sustainable, effective strategies
๐ฆ Slide 4: Methodology
- Literature review
- Stakeholder interviews & focus groups
- Case studies
- Financial data analysis
๐ฆ Slide 5: Key Findings โ Current Practices
- Overview of how costs are managed in business, government, and NGOs
- Gaps in budgeting, procurement, and resource planning
- Common inefficiencies and over-expenditures
๐ฆ Slide 6: Key Findings โ Stakeholder Feedback
- Cost pressures due to inflation, supply chain, and compliance changes
- Barriers to adopting new technologies
- Desire for clearer financial tracking systems
๐ฆ Slide 7: Economic & Legislative Impacts
- How inflation and resource scarcity affect cost structures
- Policy shifts increasing regulatory compliance costs
- Implications for long-term financial planning
๐ฆ Slide 8: Best Practices in Cost Management
- Top-performing strategies from local and global organizations
- Key themes: digitalization, strategic sourcing, performance-based budgeting
๐ฆ Slide 9: Proposed Strategies
- Streamline procurement with e-tools
- Implement zero-based budgeting
- Adopt shared service models
- Introduce real-time expenditure tracking
๐ฆ Slide 10: Actionable Recommendations
- Step-by-step implementation plan
- Timelines and responsible units
- Prioritization of quick wins vs long-term goals
๐ฆ Slide 11: Monitoring & Evaluation Framework
- Key indicators for tracking strategy success
- Frequency of progress reviews
- Reporting tools and dashboards
๐ฆ Slide 12: Benefits & Expected Outcomes
- Cost savings and ROI
- Improved efficiency and transparency
- Better service delivery across sectors
๐ฆ Slide 13: Next Steps
- Pilot program selection
- Final stakeholder consultations
- Launch of implementation phase
๐ฆ Slide 14: Q&A / Discussion
- Invite stakeholder questions and input
- Gather feedback for refinement
๐ฆ Slide 15: Thank You
Contact Info:
SayPro Economic Impact Studies Research Office
๐ง research@saypro.org | ๐ www.saypro.org -
SayPro Monitoring and Evaluation Plan
Monitoring and Evaluation Plan
SayPro Monthly April SCRR-14: Cost Management Initiative
Prepared by: SayPro Economic Impact Studies Research Office
1. Purpose of the M&E Plan
This Monitoring and Evaluation (M&E) Plan outlines how SayPro and its partners will:
- Track the implementation of cost management strategies,
- Measure their impact on organizational efficiency and financial sustainability,
- Continuously improve the strategies through feedback and learning.
2. Objectives
The objectives of this M&E Plan are to:
- Monitor the execution and adoption of cost management recommendations.
- Evaluate the effectiveness and efficiency of implemented strategies.
- Identify lessons learned and best practices for continuous improvement.
- Support evidence-based decision-making for stakeholders.
3. Key Performance Indicators (KPIs)
Category Indicator Target Frequency Implementation % of strategies fully implemented 90% by Q4 2025 Quarterly Budget Optimization % reduction in unnecessary expenses 15% average per department Bi-annually Procurement Efficiency % of purchases made via competitive processes 100% Monthly Financial Transparency % of departments using real-time financial tools 85% by Q3 2025 Quarterly Stakeholder Satisfaction Satisfaction rate in M&E feedback surveys 80% positive responses After each intervention Outcome Effectiveness % improvement in cost-effectiveness ratios +20% over baseline Annually
4. Monitoring Activities
Activity Description Responsible Party Timeline Implementation Check-ins Track whether each cost strategy is implemented as planned Departmental leads + M&E team Monthly Financial Performance Reviews Assess savings, ROI, and cost shifts Finance team Quarterly Dashboard Updates Visualize key data points using a centralized digital dashboard IT + M&E Office Bi-weekly Procurement Audits Evaluate procurement practices for compliance and efficiency Procurement Officer Twice a year Staff Feedback Surveys Collect feedback on ease, utility, and challenges of new systems HR + M&E Coordinator Post-implementation
5. Evaluation Strategy
5.1 Baseline Evaluation
- Conducted before implementation to assess current cost structures, inefficiencies, and budget flows.
5.2 Mid-Term Evaluation
- Performed halfway through the plan (e.g., Q3 2025) to assess progress, barriers, and initial outcomes.
5.3 Final Impact Evaluation
- Measures the full impact of cost management strategies on financial sustainability and service quality.
- Includes comparative analysis with baseline data.
6. Tools and Methods
Tool Purpose Cost Analysis Templates Standard format for tracking cost changes Financial Dashboards Real-time visualization of cost metrics Surveys and Interviews Qualitative feedback from stakeholders Document Reviews Verification of policy changes, contracts, reports Case Studies Detailed look at high-performing units or pilots
7. Reporting and Communication
Report Type Content Audience Schedule M&E Progress Reports Updates on KPIs, implementation, insights Internal leadership, SayPro Board Quarterly Stakeholder Briefings Simplified updates with visuals Government partners, community leaders Semi-annually Final Evaluation Report Comprehensive results and recommendations Funders, executive teams, partners Q1 2026
8. Risks and Mitigation Strategies
Potential Risk Mitigation Strategy Resistance to new monitoring tools Provide training and ongoing technical support Inconsistent data collection Standardize templates and assign clear responsibilities Budget limitations for evaluations Integrate M&E into project funding from the outset
9. Roles and Responsibilities
Role Responsibility M&E Coordinator Overall management of the monitoring plan Departmental Leads Ensure implementation tracking within departments Finance Analysts Data entry and financial trend analysis Stakeholder Engagement Lead Gathering and synthesizing external feedback