Your cart is currently empty!
Author: Matjie Maake
SayPro is a Global Solutions Provider working with Individuals, Governments, Corporate Businesses, Municipalities, International Institutions. SayPro works across various Industries, Sectors providing wide range of solutions.
Email: info@saypro.online Call/WhatsApp: Use Chat Button 👇
Written by
in
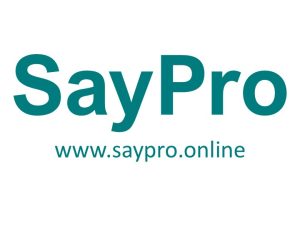