Your cart is currently empty!
Author: Sphiwe Sibiya
SayPro is a Global Solutions Provider working with Individuals, Governments, Corporate Businesses, Municipalities, International Institutions. SayPro works across various Industries, Sectors providing wide range of solutions.
Email: info@saypro.online Call/WhatsApp: Use Chat Button ๐
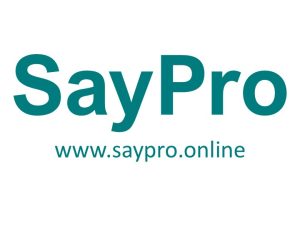
-
SayPro Organizing meetings and presentations to explain the results of the analysis to key stakeholders (e.g., government officials, industry leaders, NGOs).
SayPro Understand Your Audience
- Government Officials: Theyโre often focused on policy implications, public impact, feasibility, and budget constraints. They need clear, data-backed recommendations and an understanding of how your analysis affects the public or specific populations.
- Industry Leaders: Theyโre interested in practical, business-oriented results, including market trends, cost-benefit analysis, and implementation strategies. Focus on how the analysis can improve industry performance or profitability.
- NGOs: Non-governmental organizations often prioritize social impact, equity, and alignment with their missions. Theyโll want to know how your findings can affect the communities they serve and how they can collaborate on solutions.
SayPro Set Clear Objectives for the Meeting
- What do you want to achieve? Are you informing stakeholders, soliciting feedback, or gaining buy-in for your recommendations?
- Key Takeaways: Determine the three to five main messages or outcomes you want stakeholders to walk away with. This could be understanding the findings, agreeing on next steps, or committing to specific actions.
SayPro Choose the Right Format
- One-on-One Meetings: If you need to have in-depth discussions with specific individuals, such as a policymaker or industry leader, one-on-one meetings may be most effective.
- Workshops: If you want to gather input and feedback on your analysis, organizing a workshop where stakeholders can discuss and brainstorm is useful. This also encourages collaboration.
- Large Presentations: For broader stakeholder groups, a more formal presentation with slides, charts, and discussion segments is ideal.
- Roundtable Discussions: For interactive discussions, where you want to allow for Q&A and ensure a collaborative environment, a roundtable format can work well.
SayPro Plan the Agenda
Structure your meeting to ensure it flows logically and allows for time for key discussions and feedback.
SayPro Example Agenda for a Meeting:
- Introduction (5-10 minutes)
- Briefly introduce yourself, your role, and the purpose of the meeting.
- Provide a brief overview of the analysis and its importance.
- Presentation of Key Findings (15-20 minutes)
- Highlight the most important insights from your analysis. Use visuals (charts, graphs) to make data easy to understand.
- Be concise but clear in explaining the implications of your findings.
- Recommendations (10-15 minutes)
- Provide specific, actionable recommendations based on your analysis.
- Make sure recommendations are practical, and explain why theyโre relevant to the stakeholders.
- Discussion/Q&A (15-30 minutes)
- Allow time for stakeholders to ask questions or provide feedback. This section is crucial for gauging their perspectives and ensuring engagement.
- Address concerns, clarify points, and invite suggestions.
- Next Steps (5-10 minutes)
- Outline the immediate actions needed and any follow-up steps.
- Set expectations for timelines and responsibilities.
- Closing (5 minutes)
- Summarize key points, express appreciation for participation, and confirm the next steps or actions.
SayPro Prepare the Presentation Materials
- Visual Aids: Use slides, charts, and graphs to illustrate your findings and make complex data easier to understand. Make sure they are simple, clean, and not overloaded with information.
- Handouts/Reports: Prepare a concise handout or summary of your findings and recommendations that stakeholders can review after the meeting. If the analysis is more detailed, consider giving them the full report or key sections.
- Clear Messaging: Keep your slides and visuals focused on the main takeaways. Avoid text-heavy slides and instead use bullet points or diagrams to highlight key points.
Tip: Keep the design of the presentation professional and consistent. Avoid distractions like overly bright colors or fonts that are hard to read.
SayPro Anticipate Questions and Concerns
- Be Ready with Evidence: Stakeholders might question the methodology, data sources, or assumptions behind your analysis. Be prepared to explain these clearly and provide evidence or references.
- Address Concerns Upfront: If you anticipate concerns about feasibility, budget, or other potential challenges, be proactive in addressing them during your presentation or Q&A.
SayPro Engage Stakeholders During the Meeting
- Invite Participation: Encourage stakeholders to ask questions, provide feedback, and engage in the discussion. The more engaged they are, the more likely they are to take ownership of the recommendations.
- Facilitate Dialogue: If possible, encourage a two-way conversation rather than a one-sided presentation. Ask questions, invite suggestions, and foster a collaborative atmosphere.
SayPro Follow Up After the Meeting
- Send a Thank-You Note: After the meeting, send a brief thank-you email expressing appreciation for their time and input.
- Provide Materials: Attach the presentation, handouts, or a summary of key points from the meeting for their reference.
- Outline Next Steps: Summarize the action items or commitments made during the meeting. This reinforces the momentum and ensures follow-through.
SayPro Adapt Based on Feedback
- Use the feedback from stakeholders to refine your analysis or recommendations. They may have valuable insights that you can incorporate into the next phase of your work.
- If a stakeholder expresses concerns or has questions, address these promptly to maintain trust and credibility.
SayPro Monitor and Report on Progress
- If your presentation leads to specific actions, monitor the progress and keep stakeholders informed about developments. Regular updates can help maintain engagement and ensure accountability.
Example of an Effective Presentation Flow:
- Title Slide: “Policy Recommendations for Sustainable Urban Development”
- Introduction:
- Why urban sustainability is critical now.
- The scope of the analysis (e.g., environmental impact, economic feasibility, social equity).
- Key Findings:
- 20% of the city’s energy consumption comes from outdated infrastructure.
- Over 30% of the population lacks access to green spaces.
- Cost of inaction: potential economic losses due to climate-related disasters in the next decade.
- Recommendations:
- Invest $50 million in energy-efficient infrastructure upgrades over 5 years.
- Increase the number of parks and green spaces by 10% by 2030.
- Launch public-private partnerships to foster sustainable construction practices.
- Q&A:
- Open the floor for questions. Be prepared with data and possible rebuttals.
- Next Steps:
- Schedule follow-up meetings with key stakeholders for action planning.
- Coordinate with local agencies to prepare a detailed implementation plan.
- Closing:
- Thank everyone for their time and emphasize the importance of immediate action.
-
SayPro Writing clear and actionable reports that policymakers, stakeholders, and the public can easily understand and use.
SayPro Know Your Audience
- Policymakers: They need clear, evidence-based recommendations that can guide decision-making. Avoid jargon, but use appropriate terminology that aligns with their expertise.
- Stakeholders: This group can include community leaders, business owners, or advocacy groups. Tailor the report to their interests and concerns while offering actionable insights.
- The Public: The report should be clear, simple, and free of technical language. It should convey the key issues and what actions need to be taken in an accessible way.
SayPro Structure Your Report Clearly
A well-organized report is essential for effective communication. Hereโs a suggested structure:
- Title Page: Include the title, date, and your contact information.
- Executive Summary: Summarize the reportโs key points, findings, and recommendations. Keep it brief (no more than a page), and make it compelling so the reader can quickly grasp the most important details.
- Table of Contents: Include a table of contents if the report is lengthy, to help readers navigate.
- Introduction: Provide a brief background of the issue, its significance, and the purpose of the report.
- Methodology (if applicable): Explain how data was collected or analyzed, especially if the report is based on research, surveys, or interviews.
- Findings/Analysis: Present the data, insights, or analysis in a clear, logical order. Use bullet points, charts, and graphs where necessary to simplify complex information.
- Recommendations: Present clear, actionable recommendations. Be specific about what should be done, who should do it, and by when.
- Conclusion: Wrap up the report by summarizing the main points and re-emphasizing the importance of the recommendations.
- Appendices (if necessary): Include any supporting materials, such as data tables, charts, or detailed reports that can be referenced.
SayPro Keep It Concise and Focused
- Avoid long-winded explanations. Stick to the essentials and only include relevant information. Policymakers and stakeholders often have limited time, so clarity is crucial.
- If the report is long, break it up into digestible sections with clear subheadings.
SayPro Use Simple and Direct Language
- Write in plain language to ensure accessibility for all audiences. Avoid jargon, technical terms, or overly complex sentences.
- Be direct. Focus on what matters most and avoid unnecessary details.
SayPro Present Data Effectively
- Use graphs, charts, and tables to present quantitative data in a visually compelling way. A picture is worth a thousand words, so make sure your data is easy to understand.
- Ensure that your visual aids are clearly labeled, with concise explanations to avoid confusion.
- Include only relevant data points, and highlight trends or patterns that support your findings and recommendations.
SayPro Use Bullet Points and Lists
- Break down complex information into digestible chunks using bullet points or numbered lists. This makes the report easier to skim and helps emphasize key takeaways.
SayPro Provide Actionable Recommendations
- Be specific: Offer clear, practical steps that can be taken. For example, instead of saying, “Increase funding for education,” say, “Allocate 5% more of the annual budget to public school systems, focusing on teacher training and student support services.”
- Prioritize recommendations: Rank them by importance or feasibility, so readers know what actions should be taken first.
- Set timelines and responsibilities: Whenever possible, specify who should take action and by when.
SayPro Emphasize Key Findings and Implications
- Highlight the most critical findings from your analysis and make sure these are clearly linked to the recommendations.
- Explain the implications of not taking action. What are the risks or consequences of inaction?
SayPro Ensure Transparency and Credibility
- Cite your sources. Whether youโre using data, expert opinions, or research findings, always include references to ensure credibility.
- If there are any uncertainties or limitations in the data or analysis, acknowledge them upfront. Being transparent about these aspects builds trust.
SayPro Proofread and Edit
- Ensure the report is free from typos, errors, or unclear wording. This improves readability and professionalism.
- Edit for clarity. Reread your report and ask someone unfamiliar with the topic to check if the key points are easy to understand.
SayPro Incorporate Executive Summaries or Infographics for Quick Access
- Provide a one-page executive summary with bullet points or a visual summary (like an infographic) for busy readers who may not read the entire report.
- This can include key findings, recommendations, and a brief overview of the issue.
SayPro Provide Recommendations for Communication
- If appropriate, offer suggestions on how to communicate the findings and recommendations to broader audiences. This could involve media campaigns, public forums, or digital outreach.
Example Report Outline
Title:
“Improving Access to Affordable Healthcare: Policy Recommendations for 2025”SayPro Executive Summary:
This report examines the current state of healthcare accessibility and proposes a series of actionable recommendations to improve affordability and access for underserved populations. Key findings include a rising number of uninsured individuals and significant regional disparities in care access. Recommendations include expanding Medicaid eligibility, investing in telemedicine infrastructure, and offering subsidies to low-income individuals.SayPro Introduction:
Access to affordable healthcare remains a critical challenge for millions of Americans. Despite various reforms over the last decade, disparities persist, particularly in rural areas. This report aims to explore these issues and provide actionable solutions.SayPro Findings:
- Uninsured Rate: 15% of the population remains uninsured, with a disproportionate impact on rural communities.
- Regional Disparities: Access to healthcare is significantly lower in rural areas, with 20% fewer healthcare providers per capita compared to urban areas.
- Telemedicine: Telemedicine adoption has increased by 30%, but infrastructure remains inadequate in many areas.
SayPro Recommendations:
- Expand Medicaid: Broaden eligibility to include more low-income individuals in states that have not expanded Medicaid.
- Invest in Telemedicine: Fund initiatives that increase broadband access and telemedicine infrastructure in underserved regions.
- Subsidies for Low-Income Individuals: Implement sliding-scale subsidies for individuals earning below the federal poverty line to reduce out-of-pocket healthcare costs.
Conclusion:
Implementing these recommendations will help improve healthcare access, reduce disparities, and ensure that all individuals, regardless of income or location, can receive the care they need. -
SayPro Offering recommendations to improve the policy or to guide future policymaking efforts.
SayPro Conduct a Comprehensive Policy Review
- Assess current policy effectiveness: Evaluate how well the existing policy has been working. What are its successes and challenges? Identify gaps and areas for improvement.
- Consult relevant stakeholders: Gather input from various stakeholders, including policymakers, affected communities, experts, and advocacy groups. This helps ensure that different perspectives are considered.
SayPro Data-Driven Decision Making
- Use evidence and research: Base your recommendations on credible data, research, and case studies. Policymaking should be informed by empirical evidence to maximize the likelihood of success.
- Identify trends: Look at long-term trends that might affect the policy (e.g., demographic shifts, technological advancements, or environmental changes).
SayPro Clarify the Policy Goals
- Define clear objectives: Ensure that the policy’s goals are clear, measurable, and realistic. For example, if the policy aims to reduce unemployment, what specific target (e.g., a 5% reduction) and timeline will be set?
- Ensure alignment with broader goals: Check that the policy aligns with national, regional, or international priorities (e.g., Sustainable Development Goals, climate action targets).
SayPro Propose Specific Policy Changes
- Short-term and long-term strategies: Offer a combination of immediate actions and long-term strategies. Short-term solutions might address urgent needs, while long-term strategies can focus on systemic change.
- Innovative solutions: Consider creative and innovative policy options, including technological advancements, cross-sector collaboration, or new regulatory approaches.
- Resource allocation: Recommend adjustments to resource distribution, ensuring that adequate funding, personnel, and tools are available for implementation.
SayPro Address Implementation and Accountability
- Ensure feasibility: Make sure your recommendations are realistic in terms of implementation. This includes assessing budget constraints, political will, and institutional capacity.
- Create monitoring and evaluation frameworks: Suggest methods for tracking the progress of the policy over time. This could include performance indicators, impact assessments, and regular reviews.
- Provide clear accountability structures: Who will be responsible for each aspect of the policy’s implementation? Establish clear roles and oversight mechanisms to ensure transparency.
SayPro Consider Equity and Inclusion
- Equity considerations: Ensure that the policy benefits all groups, particularly marginalized or disadvantaged communities. Propose measures to mitigate potential disparities.
- Promote inclusive decision-making: Advocate for involving a diverse range of voices in the policy-making process, ensuring that all affected groups are represented.
SayPro Review Potential Risks
- Anticipate unintended consequences: Identify potential risks or negative side effects that might arise from implementing the policy. Offer strategies to mitigate these risks.
- Adaptability: Recommend that policies be flexible enough to adapt to changing circumstances or unexpected challenges.
SayPro Foster Collaboration
- Encourage intergovernmental cooperation: Policies often require coordination between different levels of government (e.g., federal, state, local) or between various sectors. Foster collaboration to streamline efforts.
- Leverage partnerships with the private sector and civil society: In many cases, businesses, non-profits, and community organizations can play a vital role in policy implementation.
SayPro Communicate Clearly
- Engage with the public: Ensure that policy changes and the reasons behind them are communicated effectively to the public. Clear communication can help gain public support and participation.
- Policy transparency: Advocate for transparency in the policymaking process. This ensures that the public is informed about the policy’s goals, the reasoning behind it, and the expected outcomes.
SayPro Future-Proof the Policy
- Incorporate flexibility: Design the policy with room for adjustment as new information, technologies, or social changes emerge.
- Anticipate future challenges: Consider potential challenges that could arise in the future (e.g., economic recessions, climate change, demographic shifts) and propose measures to proactively address them.
Example Recommendations (Generic):
- Policy on Climate Change: Implement stronger incentives for renewable energy adoption, expand investment in green technology research, and promote community-based climate resilience programs. Ensure that vulnerable populations receive targeted support to adapt to climate impacts.
- Healthcare Policy: Increase access to mental health services, particularly in underserved areas, by expanding telehealth options and integrating mental health care into primary care settings. Ensure affordability through subsidies for low-income individuals and families.
-
SayPro Presenting data-driven conclusions about theย successย orย failureย of the policy in achieving its intended goals.
SayPro Presenting Data-Driven Conclusions about the Success or Failure of a Policy in Achieving Its Intended Goals
When assessing the effectiveness of a policy intervention, it is crucial to present clear, data-driven conclusions that objectively evaluate whether the policy achieved its intended goals. These conclusions should be based on rigorous analysis, using appropriate statistical methods and comparing relevant data before and after the policy’s implementation. Below is a framework for presenting such conclusions.
SayPro Summary of the Policy’s Goals and Objectives
Start by briefly restating the goals and objectives of the policy intervention. This ensures that the audience understands the intended outcomes against which the policyโs success will be measured.
- Policy Description: Provide a brief overview of the policy being evaluated, including its purpose, scope, and target population.
- Example: “The policy aimed to reduce air pollution in urban areas by implementing stricter emissions regulations for industrial facilities.”
- Intended Outcomes: Outline the specific goals that the policy sought to achieve.
- Example: “The intended outcomes of the policy were a 20% reduction in air pollution levels within one year, improved public health indicators, and increased compliance by industrial facilities.”
SayPro Data Collection and Methodology
Explain the data collection process and the methods used to evaluate the policyโs impact. This section provides transparency and credibility to the findings.
- Data Sources: Specify the data sources used for analysis (e.g., government reports, surveys, industry records, health statistics).
- Example: “Air quality data were sourced from government environmental monitoring agencies, while health data were collected from hospital records and public health surveys.”
- Analysis Methods: Outline the statistical methods used to compare pre- and post-policy outcomes in the treatment and control groups.
- Example: “We used Difference-in-Differences (DiD) analysis to compare the changes in air pollution levels between the treatment (policy-affected) and control (unaffected) regions.”
SayPro Presentation of Key Findings
Present the results of your analysis, clearly showing the impact of the policy on the defined metrics. Organize the findings around the key goals of the policy.
a. Objective 1: Reduction in Pollution Levels
- Results: Present the measured changes in pollution levels in both the treatment and control groups.
- Example: “In the treatment region, air pollution levels decreased by 18% within the first 12 months of policy implementation, compared to a 5% reduction in the control region.”
- Statistical Significance: Report whether the changes are statistically significant and discuss the confidence in the results.
- Example: “The difference in reduction between treatment and control regions was statistically significant, with a p-value of 0.02, indicating that the policy contributed to the observed decline in pollution.”
SayPro Objective 2: Improvement in Public Health
- Results: Present any health-related improvements, such as reductions in respiratory diseases or hospital visits.
- Example: “There was a 15% reduction in hospital admissions for respiratory conditions in the treatment region, while no significant change was observed in the control region.”
- Statistical Significance: Ensure that the health improvements are compared and tested for significance.
- Example: “The reduction in hospital admissions in the treatment group was statistically significant (p-value < 0.05), while the control group showed no significant changes.”
SayPro Objective 3: Compliance with Emission Standards
- Results: Show how well industrial facilities in the treatment region complied with the new emission standards.
- Example: “Compliance rates among industrial facilities in the treatment region improved by 30% after the policy was implemented, compared to only 5% improvement in the control region.”
- Statistical Significance: Include tests that measure the significance of the compliance changes.
- Example: “The difference in compliance rates between the treatment and control regions was significant, with a p-value of 0.01, indicating that the policy effectively improved industrial compliance.”
SayPro Evaluation of Success or Failure Based on the Policyโs Goals
Based on the data and analysis, assess whether the policy achieved its intended goals. This should be an evidence-based evaluation, considering both the positive and negative aspects.
SayPro Successes
- Goal Achievement: Summarize the areas where the policy was successful.
- Example: “The policy successfully reduced air pollution levels and improved public health outcomes in the targeted regions. These results suggest that the policy achieved its primary goal of improving air quality and protecting public health.”
- Key Drivers: Identify the factors that contributed to the policyโs success.
- Example: “The high rate of compliance with the new emissions standards was a key factor in reducing pollution levels.”
SayPro Areas for Improvement
- Unmet Goals: Acknowledge any goals that were not fully achieved.
- Example: “While the policy reduced pollution levels by 18%, the targeted 20% reduction was not fully realized. Additionally, long-term improvements in public health have yet to be observed.”
- Challenges: Highlight any challenges or barriers encountered during policy implementation.
- Example: “Some industries in the treatment region faced significant financial burdens from the new regulations, which affected their ability to comply fully with the emissions standards.”
SayPro Broader Context and Indirect Effects
Consider any indirect effects or broader impacts that might have influenced the policy’s success or failure. This helps contextualize the results and provides a more holistic view.
- External Factors: Discuss any external factors that may have affected the outcomes.
- Example: “During the policy implementation period, the treatment region also experienced economic downturns, which may have affected industrial activity and pollution levels.”
- Spillover Effects: Evaluate whether the policy had positive or negative spillover effects on other regions or sectors.
- Example: “Some neighboring regions outside the treatment area reported improvements in air quality, suggesting that the policy may have indirectly affected surrounding areas.”
SayPro Recommendations for Future Policy Adjustments
Based on the analysis, provide actionable recommendations for improving or refining the policy for future implementation. These recommendations should be grounded in data and informed by the challenges encountered.
- Enhance Implementation: If certain aspects of the policy did not achieve the desired outcomes, suggest ways to improve them.
- Example: “To achieve the 20% reduction in pollution, we recommend stricter enforcement of emission standards and additional financial incentives for businesses to comply.”
- Address Barriers: Identify any barriers that hindered the policyโs full success and propose solutions.
- Example: “Industries that struggled to comply with emissions standards may benefit from subsidies or grants to offset the costs of compliance, helping them transition more smoothly.”
- Long-Term Monitoring: Suggest mechanisms for long-term monitoring to assess the continued effectiveness of the policy.
- Example: “To assess long-term health improvements, we recommend continuing to monitor hospital admissions for respiratory conditions in the treatment region for at least another two years.”
7. Conclusion
Conclude by summarizing the key findings and providing an overall assessment of the policyโs effectiveness.
- Success: If the policy achieved its intended goals, emphasize its positive outcomes.
- Example: “In conclusion, the policy was successful in reducing pollution levels and improving public health outcomes, demonstrating that stringent emissions regulations can lead to tangible environmental and health benefits.”
- Failure or Partial Success: If the policy did not fully achieve its goals, explain why and suggest how to adjust future interventions.
- Example: “While the policy partially succeeded in reducing pollution, the lower-than-expected reduction rate and challenges in industry compliance suggest that further adjustments are needed to improve its effectiveness.”
Final Thoughts
Data-driven conclusions should be presented in a clear, objective, and transparent manner. By providing evidence of success or failure based on measurable outcomes, you ensure that the policy’s impact is understood in a comprehensive context. This approach enables policymakers to make informed decisions about the future of the policy, whether that involves scaling it, adjusting it, or discontinuing it altogether.
- Policy Description: Provide a brief overview of the policy being evaluated, including its purpose, scope, and target population.
-
SayPro Analyzing differences in key metrics between these groups, isolating the influence of the policy from other variables.
SayPro Analyzing Differences in Key Metrics Between Treatment and Control Groups to Isolate the Influence of the Policy
Analyzing the differences in key metrics between treatment and control groups is essential to evaluate the impact of a policy intervention. The primary goal is to determine whether any observed changes in the treatment group can be attributed to the policy, rather than to other confounding factors. This involves isolating the effect of the policy from other variables that could influence the outcome.
Hereโs a detailed approach for conducting such an analysis:
SayPro Define the Key Metrics and Outcomes
Start by clearly defining the key metrics that you wish to evaluate, which should be directly linked to the objectives of the policy intervention. These metrics could include:
- Economic Outcomes: Employment rates, income levels, business activity, or GDP growth.
- Social Outcomes: Education levels, access to healthcare, crime rates, or housing quality.
- Environmental Outcomes: Pollution levels, resource consumption, or biodiversity.
- Behavioral Outcomes: Changes in consumer or business behavior, adoption rates of new technologies, or compliance with new regulations.
Ensure that the metrics are measurable, relevant, and aligned with the policy’s goals.
SayPro Establish Baseline Metrics for Both Treatment and Control Groups
Before implementing the policy, gather baseline data on the key metrics for both the treatment and control groups. This allows you to compare changes in the outcome variables before and after the policy is implemented.
- Data Collection: Collect pre-policy data on the same metrics for both the treatment and control groups. Ideally, the baseline should be collected over the same time period for both groups to avoid time-related discrepancies.
- Control for External Variables: At this stage, control for any external factors that may affect the baseline data (e.g., seasonal trends, economic cycles, external policies). This step ensures the baseline metrics are comparable.
SayPro Implement the Policy and Monitor Key Metrics
Once the baseline data is collected, implement the policy in the treatment group and continue to monitor the key metrics in both groups over time.
- Treatment Group: The group that is directly impacted by the policy intervention.
- Control Group: The group that is not impacted by the policy but is otherwise similar to the treatment group.
SayPro Conduct Post-Policy Data Collection
After the policy has been implemented, collect data on the same key metrics for both the treatment and control groups. This should be done at regular intervals (e.g., 6 months, 1 year) to assess both short-term and long-term effects.
- Timing of Data Collection: Data should be collected at specific points after the policy implementation (e.g., immediately after, 6 months after, 1 year after) to observe the timing of effects.
- Consistency in Data Sources: Use the same data sources and measurement methods as those used for the baseline data to ensure consistency.
SayPro Statistical Analysis to Analyze the Differences
Once the post-policy data is collected, use statistical techniques to compare the differences between the treatment and control groups while accounting for other variables. This helps isolate the effect of the policy from other factors.
SayPro Difference-in-Differences (DiD) Analysis
Difference-in-Differences (DiD) is a commonly used method to compare the changes in outcomes between treatment and control groups before and after the policy intervention. It helps control for time-invariant confounders and general time trends.
- Steps:
- Calculate the difference in the key metrics between the pre- and post-policy periods for the treatment group.
- Calculate the difference in the key metrics between the pre- and post-policy periods for the control group.
- The difference-in-differences is the difference between these two differences.
Formula:DiD=(Post-treatmentTreatmentโPre-treatmentTreatment)โ(Post-treatmentControlโPre-treatmentControl)\text{DiD} = (\text{Post-treatment}_\text{Treatment} – \text{Pre-treatment}_\text{Treatment}) – (\text{Post-treatment}_\text{Control} – \text{Pre-treatment}_\text{Control})DiD=(Post-treatmentTreatmentโโPre-treatmentTreatmentโ)โ(Post-treatmentControlโโPre-treatmentControlโ)
- Interpretation: The DiD estimate represents the average treatment effect on the treated (ATT) or the causal effect of the policy, isolating the policy impact from common trends affecting both groups.
SayPro Regression Analysis with Control Variables
To account for other confounding variables that might influence the key metrics, conduct a regression analysis that includes control variables (e.g., economic conditions, demographic factors, external interventions).
- Model Structure:Y=ฮฑ+ฮฒโ
Treatment+ฮณโ
Post-policy+ฮดโ
TreatmentรPost-policy+ฮธโ
X+ฯตY = \alpha + \beta \cdot \text{Treatment} + \gamma \cdot \text{Post-policy} + \delta \cdot \text{Treatment} \times \text{Post-policy} + \theta \cdot X + \epsilonY=ฮฑ+ฮฒโ
Treatment+ฮณโ
Post-policy+ฮดโ
TreatmentรPost-policy+ฮธโ
X+ฯตWhere:
- YYY is the key metric (outcome variable).
- Treatment is a binary variable (1 if the region is in the treatment group, 0 if in the control group).
- Post-policy is a binary variable (1 for post-policy period, 0 for pre-policy).
- Treatment ร Post-policy is the interaction term capturing the policy effect.
- X represents a vector of control variables.
- ฯต\epsilonฯต is the error term.
- Interpretation: The coefficient on the interaction term (ฮด\deltaฮด) gives the effect of the policy after controlling for other variables.
SayPro Propensity Score Matching (PSM)
If treatment and control groups differ on observable characteristics, you can use Propensity Score Matching (PSM) to match treatment units with control units that have similar propensity scores (probability of receiving treatment based on observed covariates).
- Steps:
- Estimate the propensity scores using logistic regression or other methods based on observed characteristics.
- Match treated regions with control regions having similar propensity scores.
- Analyze the differences in key metrics between matched pairs.
- Interpretation: This method helps reduce selection bias and ensures the comparison between treatment and control groups is fair, adjusting for pre-treatment differences.
SayPro Control for Confounders and Bias
In addition to the methods above, it is essential to identify and control for any confounders that could influence the outcomes. These may include:
- External Policies: Ensure that other policies introduced during the study period in both the treatment and control regions are accounted for.
- Economic Shocks: Account for any major economic events (e.g., recessions, global trade disruptions) that may influence the outcomes independently of the policy.
- Seasonality: Some metrics, such as employment or economic activity, may have seasonal variations that need to be accounted for.
One way to do this is by including relevant control variables in the regression models or adjusting for seasonality through fixed effects or time-series methods.
SayPro Interpret Results and Isolate Policy Impact
Once the statistical analysis is complete, interpret the results:
- Statistical Significance: Determine whether the changes observed in the treatment group are statistically significant, meaning they are unlikely to have occurred by chance.
- Magnitude of Effects: Assess the magnitude of the effect size (e.g., how much the policy changed employment rates or income levels in the treatment group compared to the control group).
- Attribution to the Policy: Ensure that the differences in outcomes are causally attributed to the policy rather than to other confounding factors.
- Policy Impact: If the results show a significant and positive difference between the treatment and control groups, the policy can be considered effective. However, if no significant difference is found, it suggests that the policy had little or no impact, or other factors may have masked its effects.
SayPro Report Findings and Make Recommendations
Finally, report the findings of your analysis:
- Results Summary: Present the key metrics and the changes observed in both the treatment and control groups, highlighting the effects of the policy.
- Policy Implications: Based on the findings, provide recommendations on whether the policy should be continued, adjusted, or abandoned.
- Recommendations for Future Research: Suggest areas where further research or data collection is needed, such as exploring long-term effects or evaluating the policy’s impact on different subgroups.
Conclusion
By analyzing differences in key metrics between treatment and control groups, you can isolate the impact of a policy intervention and evaluate its effectiveness. Using statistical methods like Difference-in-Differences (DiD), regression analysis, and Propensity Score Matching (PSM), you can control for confounding variables and ensure that the observed changes are genuinely due to the policy. This rigorous approach helps policymakers make informed decisions based on evidence and contributes to more effective policy design and implementation.
-
SayPro Developing control and treatment groups to compare regions affected by the policy with those that were not.
SayPro Developing Control and Treatment Groups to Compare Regions Affected by the Policy with Those That Were Not
Developing control and treatment groups is a fundamental aspect of evaluating the effectiveness of a policy intervention. By comparing regions or groups affected by the policy (treatment group) with those that are not (control group), we can assess the causal effects of the policy on various outcomes. This process allows for a clearer understanding of whether observed changes in the treatment regions can be attributed to the policy, as opposed to other factors.
Here is a step-by-step guide on how to develop control and treatment groups for such a comparison:
SayPro Define the Policy and Its Goals
Before developing the control and treatment groups, you need to clearly define the policy and its objectives. This will guide the selection of the treatment and control groups and ensure that the analysis is aligned with the policyโs intended outcomes.
- Policy Description: What is the policy intervention? (e.g., a new tax incentive, environmental regulation, education reform)
- Target Population: Who is the policy intended to affect? (e.g., low-income households, businesses, schools)
- Desired Outcomes: What are the measurable outcomes expected from the policy? (e.g., employment rates, educational attainment, health outcomes)
SayPro Define Treatment and Control Groups
The treatment group consists of regions or populations that are directly affected by the policy, while the control group includes regions or populations that are similar but not subject to the policy. The goal is to ensure that both groups are as similar as possible except for the intervention, allowing for a valid comparison.
a. Treatment Group Selection
- Criteria for Selection: Identify regions (e.g., cities, districts, provinces, or countries) or individuals that will be directly impacted by the policy. These regions should be targeted for policy implementation.
- Example: If the policy is aimed at reducing air pollution through stricter emissions standards, the treatment group may consist of cities or industrial zones where these new standards are being enforced.
- Baseline Comparability: Ensure that the treatment regions are similar to control regions in
- }|0 key characteristics before the policy is implemented. For example, treatment regions should have similar demographics, economic conditions, and environmental factors.
- Example: If the policy aims to improve healthcare access, ensure that treatment regions have similar healthcare infrastructure as control regions before the policy rollout.
SayPro Control Group Selection
- Matching Criteria: Select regions or populations that are as similar as possible to the treatment group but are not affected by the policy. This is crucial for controlling for confounding variables (factors that could influence the outcome independently of the policy).
- Example: If a policy impacts urban areas, a control group could consist of similar, nearby urban areas that are not subject to the policy.
- Exclusion Criteria: Make sure that regions in the control group do not receive the policy intervention during the evaluation period. This could involve geographic areas, industries, or population groups that are not included in the policy implementation.
- External Factors: Ensure the control group does not experience similar interventions or external factors during the study period that could confound the results.
- Example: If the policy being studied is a financial stimulus, ensure that the control regions do not receive similar economic interventions from other sources (e.g., a national stimulus program).
SayPro Ensure Baseline Comparability
To ensure that the treatment and control groups are comparable, itโs essential to examine their characteristics before the policy is implemented. This can help identify any pre-existing differences that might affect the outcomes, allowing you to account for them in the analysis.
- Pre-Treatment Data Collection: Gather data on key variables (e.g., economic performance, health outcomes, employment rates) before the policy is implemented in both the treatment and control groups.
- Example: If evaluating a job training program, collect baseline data on employment rates, average income, and education levels before the policy intervention in both treatment and control regions.
- Matching Methods: Use statistical matching techniques (e.g., propensity score matching, nearest neighbor matching, or covariate matching) to pair treatment regions with similar control regions based on key characteristics. This helps to ensure that any differences in outcomes can be more confidently attributed to the policy rather than pre-existing differences between the groups.
- Propensity Score Matching: Estimate the likelihood (propensity) of being in the treatment group based on observed characteristics, and match treated and untreated regions with similar propensities.
SayPro Control for Confounding Variables
Confounding variables are factors that could influence the outcomes in both the treatment and control groups, leading to biased results if not controlled for. It is important to identify and account for these variables in your analysis.
- Common Confounders: These might include factors like local economic conditions, demographic characteristics, or external interventions that could affect both the treatment and control regions.
- Data Sources: Use additional data sources (e.g., census data, economic reports, public health data) to track and control for potential confounders.
- Statistical Controls: In regression models, include control variables that account for these confounding factors to isolate the effect of the policy.
- Example: If studying the effect of a policy on income growth, include variables like industry mix, education levels, and employment rates as controls to account for factors that could independently affect income.
SayPro Monitor External Influences and Spillover Effects
In some cases, policies may have indirect effects that spill over into control regions, especially if the regions are geographically close or share economic ties.
- Spillover Effects: A policy that affects one region might influence neighboring regions or industries. For example, an environmental regulation in one city might reduce pollution in neighboring areas even if they are not subject to the policy.
- Monitoring Spillover: Monitor the control regions during the study period to ensure that no unintended spillover effects occur. If spillovers are suspected, adjust the analysis to account for them.
- Boundary Effects: Ensure that the regions selected for control are sufficiently far from the treatment regions to avoid any overlap in effects, but still share relevant characteristics that make them suitable comparisons.
SayPro Conduct the Evaluation
Once the treatment and control groups are defined and baseline data is collected, the policy can be implemented. After a sufficient period of time, data on the key outcomes can be collected again to evaluate the policyโs impact.
- Post-Treatment Data Collection: After the policy intervention has been in place for a specified period, gather data on the same key outcome variables from both the treatment and control regions.
- Example: Collect data on employment, health outcomes, or education attainment in both regions after the policy is implemented.
- Longitudinal Design: If possible, track the outcomes over time (e.g., 6 months, 1 year, or more) to observe both short-term and long-term effects.
- Statistical Analysis: Use statistical methods, such as difference-in-differences (DiD) or regression analysis, to compare changes in outcomes between the treatment and control groups over time. These methods can help isolate the policyโs effect from other confounding factors.
- Difference-in-Differences (DiD): A common method for evaluating policy impacts is DiD, which compares the pre- and post-treatment differences in outcomes between the treatment and control groups.
SayPro Interpret Results and Make Recommendations
After conducting the analysis, interpret the results to determine whether the policy achieved its intended outcomes in the treatment group compared to the control group.
- Effectiveness: Did the policy lead to measurable improvements in the desired outcomes (e.g., improved employment rates, reduced pollution)?
- Unintended Consequences: Were there any negative or unexpected outcomes in the treatment group that were not observed in the control group?
- Recommendations: Based on the findings, provide recommendations for future policy design, adjustments, or expansions.
Conclusion
Developing control and treatment groups is a crucial step in evaluating the effectiveness of a policy. By carefully selecting comparable regions or populations, controlling for confounding variables, and using appropriate statistical methods, you can isolate the impact of the policy and assess its true effectiveness. This rigorous approach
-
SayPro Analyzing the sensitivity of the models to different assumptions and variables, ensuring robustness and accuracy.
SayPro Analyzing the Sensitivity of Models to Different Assumptions and Variables
Analyzing the sensitivity of models to different assumptions and variables is a critical step in understanding the robustness and accuracy of the model’s predictions. Sensitivity analysis helps to identify how changes in key inputs (variables, assumptions, parameters) affect the model’s outputs. By performing sensitivity analysis, you can assess which factors have the most influence on the model, identify potential sources of uncertainty, and ensure that the model’s predictions are reliable and can withstand variations in real-world conditions.
Here’s a step-by-step approach to conducting sensitivity analysis and ensuring the robustness and accuracy of your models:
SayPro Define the Purpose of Sensitivity Analysis
Before diving into the analysis, clarify why you are performing sensitivity analysis. The goals typically include:
- Identifying Key Drivers: Determine which variables or assumptions have the most significant impact on the model’s outputs.
- Testing Robustness: Assess the model’s stability and how sensitive the results are to changes in assumptions or inputs.
- Uncertainty Quantification: Understand the degree of uncertainty in the model’s predictions due to variability in input parameters.
- Model Validation: Validate the model by testing how well it performs under different conditions.
SayPro Identify Key Assumptions and Variables
Identify the assumptions and variables that are likely to influence the model’s outputs. These might include:
SayPro Economic Variables:
- Interest Rates: How sensitive is the model to changes in economic conditions (e.g., monetary policy changes)?
- Inflation Rates: How does inflation affect long-term economic outcomes?
- Investment Levels: How do shifts in private or public investment impact economic growth?
SayPro Social Behavior Variables:
- Consumer Preferences: How do changes in consumer behavior (e.g., towards sustainable products) affect economic outcomes?
- Policy Compliance: What happens if compliance rates with new policies are lower or higher than expected?
- Social Norms: How do shifts in social attitudes (e.g., towards climate change or education) affect the model?
SayPro Demographic Variables:
- Population Growth: How do changes in birth rates or migration affect long-term population dynamics?
- Age Structure: How does the age distribution affect labor force participation or health outcomes?
- Migration Patterns: What happens if migration flows are higher or lower than expected?
SayPro Environmental Variables:
- Resource Availability: How do changes in the availability of natural resources (e.g., water, energy) influence long-term sustainability?
- Emissions: How sensitive is the model to shifts in emission reduction efforts or technological advancements?
SayPro Choose Sensitivity Analysis Methods
There are several methods for conducting sensitivity analysis, depending on the complexity of the model and the data available. Some common methods include:
SayPro One-At-A-Time (OAT) Sensitivity Analysis
In One-At-A-Time (OAT) analysis, you change one input variable at a time while keeping all other variables constant to examine the effect on the output.
- Steps:
- Select the input variable you want to test.
- Change this variable by a specific amount (e.g., ยฑ10% of its baseline value).
- Observe how the model output changes in response to the altered input.
- Repeat this process for other variables.
- Pros: Simple to implement, useful for getting a quick understanding of the modelโs response to individual variables.
- Cons: Does not capture interactions between variables, so it may overlook the combined effects of changes in multiple inputs.
SayPro Multi-Variable Sensitivity Analysis
Instead of changing one variable at a time, multi-variable sensitivity analysis changes multiple variables simultaneously to observe how they interact and impact the model’s outcome. This approach is more reflective of real-world situations where several factors change concurrently.
- Steps:
- Select a range of input variables and define plausible ranges for each.
- Use a method (such as Latin Hypercube Sampling or Monte Carlo Simulation) to sample combinations of variables from within their defined ranges.
- Run the model with each combination of inputs and observe the distribution of outputs.
- Pros: Captures the combined effects of changes in multiple variables, providing a more comprehensive view of model sensitivity.
- Cons: More computationally intensive and complex.
SayPro Monte Carlo Simulation
Monte Carlo simulations use random sampling to simulate a range of possible outcomes by varying multiple input parameters. This method is effective for quantifying uncertainty and testing the modelโs robustness under different conditions.
- Steps:
- Define probability distributions for the uncertain parameters (e.g., a normal distribution for interest rates, a uniform distribution for population growth rates).
- Randomly sample values from the defined distributions to generate a set of inputs.
- Run the model for each set of inputs, recording the corresponding output.
- Analyze the range of possible outcomes to assess the modelโs robustness and the degree of uncertainty in the results.
- Pros: Provides a detailed view of the uncertainty in the model’s predictions and can help quantify the likelihood of different outcomes.
- Cons: Computationally intensive and requires substantial data to define the probability distributions accurately.
SayPro Sensitivity Indices (e.g., Sobol Indices)
Sobol indices and other global sensitivity analysis methods are useful when you need to quantify the contribution of each input parameter to the variability in model outputs.
- Steps:
- Run simulations across a large number of input combinations.
- Use the Sobol method (or similar techniques) to calculate sensitivity indices, which quantify how much each input variable contributes to the variance in the output.
- Pros: Provides a more formal, quantitative understanding of the importance of each input parameter and its interactions.
- Cons: Can be complex to implement, especially for high-dimensional models.
SayPro Perform Sensitivity Analysis and Interpret Results
Once you have chosen the sensitivity analysis method, run the analysis and interpret the results:
SayPro Identify Key Drivers
- Sensitivity of Outputs: Determine which variables have the greatest effect on the modelโs output. These key drivers should be closely monitored or refined to improve model accuracy.
- Prioritize Assumptions: Focus on parameters that cause the most significant changes in outcomes and consider refining these assumptions or collecting better data for them.
SayPro Evaluate Robustness
- Model Stability: Assess how stable the model’s predictions are when input variables are varied within reasonable bounds. A robust model will exhibit relatively consistent outputs even when small changes occur in input variables.
- Thresholds: Identify any threshold effects where a small change in one variable causes a large shift in the output. This may indicate potential vulnerabilities or areas of risk.
SayPro Quantify Uncertainty
- Uncertainty in Predictions: Sensitivity analysis can reveal the uncertainty inherent in model predictions. By assessing how different inputs contribute to overall uncertainty, you can estimate the confidence intervals for key outcomes and communicate the range of possible results to stakeholders.
SayPro Refine the Model Based on Sensitivity Results
After performing the sensitivity analysis, use the results to refine and improve the model:
SayPro Address Highly Sensitive Parameters
If certain variables or assumptions have a high sensitivity, it might be necessary to:
- Collect more precise data for these parameters.
- Consider updating the model with more realistic values for those inputs.
- Incorporate additional sources of uncertainty if the assumptions were overly simplified.
SayPro Simplify the Model (if needed)
In some cases, sensitivity analysis reveals that certain variables or assumptions have little impact on the model’s outcome. In such cases, the model can be simplified by removing unnecessary complexity, improving computational efficiency without sacrificing accuracy.
SayPro Adjust for Real-World Conditions
Ensure that the model is calibrated to reflect real-world scenarios more accurately. If certain assumptions (e.g., about consumer behavior or economic growth rates) are unrealistic, update these assumptions based on current trends, expert input, or historical data.
SayPro Communicate Findings and Recommendations
The results of sensitivity analysis should be communicated clearly to stakeholders. This helps decision-makers understand which variables are most crucial for the success of the policy and where uncertainties lie.
SayPro Key Communication Points:
- Key Sensitivities: Highlight the variables that most influence the model’s outcomes.
- Uncertainty: Discuss the level of uncertainty in the modelโs predictions and explain the likelihood of different outcomes.
- Implications for Policy: Show how changes in assumptions or variables could affect policy outcomes, and use this information to guide decision-making.
Conclusion
Analyzing the sensitivity of models to different assumptions and variables is a critical step in ensuring the modelโs robustness and accuracy. By employing methods like One-At-A-Time Analysis, Monte Carlo Simulations, and Sensitivity Indices, you can identify the most influential factors, quantify uncertainty, and refine the model to make it more reliable. This process ensures that the model is capable of providing meaningful insights that are not overly sensitive to small changes in assumptions, thus supporting better, more informed decision-making.
-
SayPro Calibrating the models based on real-world data and testing their predictive capabilities.
SayPro Calibrating Models Based on Real-World Data and Testing Predictive Capabilities
Calibrating models and testing their predictive capabilities are critical steps in ensuring that policy impact simulations are accurate, reliable, and meaningful. Calibration refers to adjusting model parameters to better align with real-world observations, while testing predictive capabilities involves evaluating how well the model can forecast outcomes based on historical or unseen data. This process ensures that the models provide valuable insights that can guide decision-making in real-world contexts.
Hereโs a structured approach to calibrating simulation models and testing their predictive abilities:
SayPro Collect Real-World Data for Calibration
Before calibration, you need accurate and comprehensive real-world data that reflects the key variables influencing the model. The quality of this data is crucial because the modelโs calibration depends on it.
SayPro Types of Data to Collect:
- Historical Data: Gather data on relevant variables before the policy intervention to establish baseline conditions.
- Economic Indicators: GDP, unemployment rates, inflation, etc.
- Demographic Data: Population size, migration patterns, age distribution, etc.
- Behavioral Data: Consumption patterns, social behaviors, policy compliance rates.
- Environmental Data: Carbon emissions, resource usage, land-use changes.
- Post-Intervention Data: After the policy is implemented, continue to track the same variables to understand the immediate and long-term impacts.
- Impact of Policies: Data showing shifts in economic activity, social behavior changes, or environmental outcomes due to the policy.
- Data Granularity: Ensure the data is disaggregated enough (e.g., by region, sector, or demographic group) to capture detailed changes across different segments.
SayPro Select Calibration Methods
Calibration is the process of adjusting model parameters so that the simulated results align with real-world data. Several methods can be used for calibration, depending on the complexity of the model and the type of data available.
SayPro Methods of Calibration:
SayPro Parameter Estimation (Manual Calibration)
In simpler models, calibration might be done by manually adjusting key parameters to achieve a match between simulated results and observed data. For example:
- Adjusting the elasticity of demand in an economic model.
- Tuning a feedback loop in a system dynamics model to better reflect real-world behavior.
Steps:
- Identify Key Parameters: Determine which parameters in the model have the most significant influence on the outcomes.
- Compare Simulated and Real Data: Run the model and compare its outputs with observed real-world data.
- Adjust Parameters: Make incremental changes to the model parameters until the simulated outcomes match observed data as closely as possible.
SayPro Optimization Algorithms (Automated Calibration)
For more complex models, optimization algorithms can be used to automatically adjust parameters. This method leverages mathematical techniques to find the best set of parameters that minimizes the error between simulated and observed data.
- Techniques:
- Gradient Descent: A method that iteratively adjusts model parameters to minimize the difference between predictions and actual outcomes.
- Bayesian Inference: A probabilistic approach that allows you to update beliefs about model parameters based on observed data and prior knowledge.
- Genetic Algorithms: A heuristic optimization approach where the model parameters evolve through generations, selecting those that best match the data.
Steps:
- Define an Objective Function: This function calculates the difference between the simulated and observed results (often using mean squared error or likelihood functions).
- Run Optimization: Use an algorithm like Gradient Descent to iteratively adjust the model’s parameters to minimize this difference.
- Validate: Once parameters are optimized, validate the model with a separate data set (if available) to ensure itโs not overfitting to the training data.
SayPro Calibration Using Bayesian Methods
Bayesian calibration is ideal when there’s uncertainty in the model parameters. It allows you to update prior beliefs about parameters based on the data you collect and to quantify uncertainty in the predictions.
- Steps:
- Prior Distribution: Define a prior belief about model parameters based on expert knowledge or historical data.
- Likelihood Function: Calculate the likelihood of the observed data given the current parameters.
- Posterior Distribution: Use Bayes’ Theorem to update the prior distribution with the likelihood to get the posterior distribution.
- Simulate the Model: Use the posterior distribution to generate simulations of the policyโs impacts, accounting for uncertainty in the parameters.
SayPro Test Predictive Capabilities
Once the model has been calibrated, itโs essential to test its ability to predict future outcomes. This step ensures that the model not only fits past data but can also make accurate predictions about the effects of the policy going forward.
SayPro Steps to Test Predictive Capabilities:
SayPro Out-of-Sample Validation
Out-of-sample validation involves testing the model using data that was not included in the calibration process. This helps assess how well the model generalizes to new data and whether it can predict future events accurately.
- Steps:
- Holdout Data: Set aside a portion of your data (e.g., 20-30%) for validation purposes. This data should represent real-world outcomes after the policy has been implemented.
- Simulate Outcomes: Run the calibrated model using the parameters derived during calibration.
- Compare Predicted vs. Actual: Evaluate the model’s predictions by comparing them to the actual outcomes in the holdout data.
- Error Metrics: Use error metrics like Mean Absolute Error (MAE), Root Mean Squared Error (RMSE), or R-squared to quantify how well the model performs.
SayPro Cross-Validation
Cross-validation is another approach that involves splitting the data into multiple subsets and training and testing the model on different combinations of these subsets. This is particularly useful when the data is limited.
- Steps:
- Split Data: Divide the available data into K-folds (e.g., 10-fold cross-validation).
- Train and Test: For each fold, use K-1 folds to calibrate the model and the remaining fold to test its predictive ability.
- Average Performance: Calculate the average prediction error across all folds to get a robust estimate of the modelโs predictive performance.
SayPro Sensitivity Analysis
Sensitivity analysis tests how sensitive the modelโs predictions are to changes in input parameters. It helps identify which variables or assumptions have the greatest impact on the modelโs predictions and whether the model is robust to changes in real-world conditions.
- Steps:
- Vary Parameters: Systematically vary input parameters (e.g., economic growth rate, migration patterns) within plausible ranges.
- Evaluate Sensitivity: Assess how much the modelโs output changes in response to these variations.
- Uncertainty Quantification: Use this analysis to quantify the degree of uncertainty in the modelโs predictions.
SayPro Refine the Model Based on Validation Results
After testing the modelโs predictive capabilities, you might need to refine it to improve its accuracy.
- Addressing Overfitting: If the model performs exceptionally well on the training data but poorly on new data, it may be overfitting. Consider simplifying the model or using regularization techniques to avoid overfitting.
- Improving Calibration: If the predictive performance is lacking, revisit the calibration process. This could involve adjusting model assumptions, collecting additional data, or using alternative calibration techniques.
- Iterative Process: Model calibration and testing is an iterative process. Regularly update the model as new data becomes available or as policies change.
5. Communication and Decision Support
Once the model has been calibrated and validated, it can be used to support decision-making by predicting potential policy outcomes under various scenarios. Clear communication of the modelโs assumptions, results, and limitations is essential for policymakers to make informed decisions.
- Visualization: Use charts, graphs, and scenario analyses to present model predictions in an understandable format.
- Scenario Planning: Offer multiple policy scenarios with different assumptions to help stakeholders understand the range of possible outcomes.
- Uncertainty Assessment: Communicate the uncertainty in predictions to help stakeholders account for potential risks and unknowns.
Conclusion
Calibrating simulation models based on real-world data and testing their predictive capabilities are essential steps for ensuring the validity and usefulness of policy impact simulations. By using methods like parameter estimation, optimization algorithms, and Bayesian calibration, and testing with out-of-sample data, sensitivity analysis, and cross-validation, you can refine the models and improve their accuracy. Once validated, these mo
- Historical Data: Gather data on relevant variables before the policy intervention to establish baseline conditions.
-
SayPro Calibrating the models based on real-world data and testing their predictive capabilities.
SayPro Calibrating Models Based on Real-World Data and Testing Predictive Capabilities
Calibrating models and testing their predictive capabilities are critical steps in ensuring that policy impact simulations are accurate, reliable, and meaningful. Calibration refers to adjusting model parameters to better align with real-world observations, while testing predictive capabilities involves evaluating how well the model can forecast outcomes based on historical or unseen data. This process ensures that the models provide valuable insights that can guide decision-making in real-world contexts.
Hereโs a structured approach to calibrating simulation models and testing their predictive abilities:
SayPro Collect Real-World Data for Calibration
Before calibration, you need accurate and comprehensive real-world data that reflects the key variables influencing the model. The quality of this data is crucial because the modelโs calibration depends on it.
SayPro Types of Data to Collect:
- Historical Data: Gather data on relevant variables before the policy intervention to establish baseline conditions.
- Economic Indicators: GDP, unemployment rates, inflation, etc.
- Demographic Data: Population size, migration patterns, age distribution, etc.
- Behavioral Data: Consumption patterns, social behaviors, policy compliance rates.
- Environmental Data: Carbon emissions, resource usage, land-use changes.
- Post-Intervention Data: After the policy is implemented, continue to track the same variables to understand the immediate and long-term impacts.
- Impact of Policies: Data showing shifts in economic activity, social behavior changes, or environmental outcomes due to the policy.
- Data Granularity: Ensure the data is disaggregated enough (e.g., by region, sector, or demographic group) to capture detailed changes across different segments.
SayPro Select Calibration Methods
Calibration is the process of adjusting model parameters so that the simulated results align with real-world data. Several methods can be used for calibration, depending on the complexity of the model and the type of data available.
SayPro Methods of Calibration:
SayPro Parameter Estimation (Manual Calibration)
In simpler models, calibration might be done by manually adjusting key parameters to achieve a match between simulated results and observed data. For example:
- Adjusting the elasticity of demand in an economic model.
- Tuning a feedback loop in a system dynamics model to better reflect real-world behavior.
Steps:
- Identify Key Parameters: Determine which parameters in the model have the most significant influence on the outcomes.
- Compare Simulated and Real Data: Run the model and compare its outputs with observed real-world data.
- Adjust Parameters: Make incremental changes to the model parameters until the simulated outcomes match observed data as closely as possible.
SayPro Optimization Algorithms (Automated Calibration)
For more complex models, optimization algorithms can be used to automatically adjust parameters. This method leverages mathematical techniques to find the best set of parameters that minimizes the error between simulated and observed data.
- Techniques:
- Gradient Descent: A method that iteratively adjusts model parameters to minimize the difference between predictions and actual outcomes.
- Bayesian Inference: A probabilistic approach that allows you to update beliefs about model parameters based on observed data and prior knowledge.
- Genetic Algorithms: A heuristic optimization approach where the model parameters evolve through generations, selecting those that best match the data.
Steps:
- Define an Objective Function: This function calculates the difference between the simulated and observed results (often using mean squared error or likelihood functions).
- Run Optimization: Use an algorithm like Gradient Descent to iteratively adjust the model’s parameters to minimize this difference.
- Validate: Once parameters are optimized, validate the model with a separate data set (if available) to ensure itโs not overfitting to the training data.
SayPro Calibration Using Bayesian Methods
Bayesian calibration is ideal when there’s uncertainty in the model parameters. It allows you to update prior beliefs about parameters based on the data you collect and to quantify uncertainty in the predictions.
- Steps:
- Prior Distribution: Define a prior belief about model parameters based on expert knowledge or historical data.
- Likelihood Function: Calculate the likelihood of the observed data given the current parameters.
- Posterior Distribution: Use Bayes’ Theorem to update the prior distribution with the likelihood to get the posterior distribution.
- Simulate the Model: Use the posterior distribution to generate simulations of the policyโs impacts, accounting for uncertainty in the parameters.
SayPro Test Predictive Capabilities
Once the model has been calibrated, itโs essential to test its ability to predict future outcomes. This step ensures that the model not only fits past data but can also make accurate predictions about the effects of the policy going forward.
SayPro Steps to Test Predictive Capabilities:
SayPro Out-of-Sample Validation
Out-of-sample validation involves testing the model using data that was not included in the calibration process. This helps assess how well the model generalizes to new data and whether it can predict future events accurately.
- Steps:
- Holdout Data: Set aside a portion of your data (e.g., 20-30%) for validation purposes. This data should represent real-world outcomes after the policy has been implemented.
- Simulate Outcomes: Run the calibrated model using the parameters derived during calibration.
- Compare Predicted vs. Actual: Evaluate the model’s predictions by comparing them to the actual outcomes in the holdout data.
- Error Metrics: Use error metrics like Mean Absolute Error (MAE), Root Mean Squared Error (RMSE), or R-squared to quantify how well the model performs.
SayPro Cross-Validation
Cross-validation is another approach that involves splitting the data into multiple subsets and training and testing the model on different combinations of these subsets. This is particularly useful when the data is limited.
- Steps:
- Split Data: Divide the available data into K-folds (e.g., 10-fold cross-validation).
- Train and Test: For each fold, use K-1 folds to calibrate the model and the remaining fold to test its predictive ability.
- Average Performance: Calculate the average prediction error across all folds to get a robust estimate of the modelโs predictive performance.
SayPro Sensitivity Analysis
Sensitivity analysis tests how sensitive the modelโs predictions are to changes in input parameters. It helps identify which variables or assumptions have the greatest impact on the modelโs predictions and whether the model is robust to changes in real-world conditions.
- Steps:
- Vary Parameters: Systematically vary input parameters (e.g., economic growth rate, migration patterns) within plausible ranges.
- Evaluate Sensitivity: Assess how much the modelโs output changes in response to these variations.
- Uncertainty Quantification: Use this analysis to quantify the degree of uncertainty in the modelโs predictions.
SayPro Refine the Model Based on Validation Results
After testing the modelโs predictive capabilities, you might need to refine it to improve its accuracy.
- Addressing Overfitting: If the model performs exceptionally well on the training data but poorly on new data, it may be overfitting. Consider simplifying the model or using regularization techniques to avoid overfitting.
- Improving Calibration: If the predictive performance is lacking, revisit the calibration process. This could involve adjusting model assumptions, collecting additional data, or using alternative calibration techniques.
- Iterative Process: Model calibration and testing is an iterative process. Regularly update the model as new data becomes available or as policies change.
5. Communication and Decision Support
Once the model has been calibrated and validated, it can be used to support decision-making by predicting potential policy outcomes under various scenarios. Clear communication of the modelโs assumptions, results, and limitations is essential for policymakers to make informed decisions.
- Visualization: Use charts, graphs, and scenario analyses to present model predictions in an understandable format.
- Scenario Planning: Offer multiple policy scenarios with different assumptions to help stakeholders understand the range of possible outcomes.
- Uncertainty Assessment: Communicate the uncertainty in predictions to help stakeholders account for potential risks and unknowns.
Conclusion
Calibrating simulation models based on real-world data and testing their predictive capabilities are essential steps for ensuring the validity and usefulness of policy impact simulations. By using methods like parameter estimation, optimization algorithms, and Bayesian calibration, and testing with out-of-sample data, sensitivity analysis, and cross-validation, you can refine the models and improve their accuracy. Once validated, these models can provide critical insights into the long-term impacts of policy decisions, helping policymakers navigate uncertainty and make more informed choices.
- Historical Data: Gather data on relevant variables before the policy intervention to establish baseline conditions.
-
SayPro Designing models that simulate the spread of policy impacts over time, considering various variables (e.g., economic, demographic).
SayPro Designing Models to Simulate the Spread of Policy Impacts Over Time
Simulating the spread of policy impacts over time requires a robust model that accounts for multiple variables such as economic factors, demographic shifts, social behavior changes, and other relevant indicators. A well-designed simulation model can help policymakers understand the long-term effects of their decisions, identify unintended consequences, and adjust strategies before implementation.
Below is an outline of how to design a simulation model for policy impact spread, along with key variables and methodologies to consider.
SayPro Define the Objective of the Model
Before designing the model, clarify the policy goal and what aspects of its spread need to be simulated. Possible objectives could include:
- Estimating long-term economic outcomes: How will a policy affect economic growth, employment, or income distribution over time?
- Modeling social behavior shifts: How will changes in laws, norms, or incentives influence individual or community behavior?
- Assessing environmental impacts: What are the indirect environmental consequences (e.g., carbon emissions reduction, resource usage) over a certain period?
- Predicting demographic shifts: How will the policy impact population dynamics, migration, or aging populations?
SayPro Identify Key Variables and Assumptions
The model needs to account for various economic, demographic, social, and environmental variables that influence the spread and long-term effects of a policy.
Economic Variables:
- GDP Growth: The overall economic output.
- Unemployment Rate: Impact on job creation or displacement.
- Income Distribution: How the policy affects different income groups.
- Investment Levels: The policy’s influence on private and public sector investment.
- Sectoral Growth: Changes in specific sectors (e.g., renewable energy, education, health).
SayPro Demographic Variables:
- Population Growth: Changes in population size and structure (age, gender, ethnicity).
- Migration Patterns: Movement of individuals within or between regions as a result of the policy (e.g., rural-to-urban migration, international migration).
- Life Expectancy: Long-term effects on health policies, such as life expectancy improvements.
- Education Levels: Shifts in educational attainment resulting from policies like school reforms.
SayPro Social Behavior Variables:
- Social Norms and Attitudes: How societal views on health, environment, or economics change in response to policy.
- Consumer Behavior: How consumption patterns (e.g., in healthcare, energy) shift in response to policy incentives.
- Civic Engagement: Changes in political participation, trust in government, or social movements.
SayPro Environmental Variables:
- Carbon Emissions: Impact of policy on emissions, especially in environmental policies.
- Resource Consumption: Changes in natural resource usage due to policies promoting sustainability.
- Biodiversity and Ecosystem Health: Long-term environmental effects, such as conservation efforts or land use changes.
SayPro Assumptions:
- Causal Relationships: Assumptions about how variables influence each other (e.g., tax incentives lead to increased business investment).
- Lag Times: How long it takes for policy effects to manifest (e.g., economic effects may take several years to be fully realized).
- Policy Scope: The geographic and demographic scope of the policy (e.g., national vs. regional policy effects).
SayPro Choose a Simulation Framework
Several types of models can simulate the spread of policy impacts. The choice depends on the complexity of the system being modeled and the data available. Below are common frameworks:
SayPro System Dynamics Modeling (SDM)
System Dynamics is ideal for modeling complex systems with feedback loops and delays, which are often present in policy impacts. It focuses on the causal relationships between variables and how these relationships evolve over time.
- Model Structure:
- Stocks: Quantities that accumulate over time (e.g., population size, capital investment, or carbon emissions).
- Flows: Rates at which stocks change (e.g., birth rates, death rates, investment rates).
- Feedback Loops: Positive or negative feedback that can accelerate or dampen the spread of impacts (e.g., an increase in employment could lead to increased consumption, stimulating further economic growth).
- Example: Modeling how a policy promoting renewable energy might gradually reduce fossil fuel dependence over several decades, impacting job creation in renewable industries, energy consumption patterns, and environmental sustainability.
SayPro Agent-Based Modeling (ABM)
Agent-based modeling simulates the interactions of individual agents (people, organizations, governments) within a system. Itโs particularly useful when the behavior of individuals or entities needs to be modeled in a detailed, heterogeneous manner.
- Model Structure:
- Agents: Individual decision-makers (e.g., consumers, firms, voters).
- Rules: How agents behave based on their attributes (e.g., economic preferences, political views).
- Interactions: How agents interact with each other (e.g., a consumerโs decision to adopt a sustainable product based on peer behavior).
- Example: Simulating how a carbon tax policy influences individual consumer behavior, with agents deciding whether to adopt energy-efficient technologies based on price incentives, social influence, and environmental awareness.
SayPro Monte Carlo Simulation
Monte Carlo simulations use random sampling to model uncertainty and predict a range of possible outcomes. This is useful for understanding how different variables and uncertainties (such as economic shocks or social behavior changes) influence the spread of policy impacts over time.
- Model Structure:
- Input Variables: Variables with uncertainty (e.g., interest rates, migration patterns).
- Probability Distributions: Defining the uncertainty in variables (e.g., using historical data or expert judgment).
- Random Sampling: Simulating thousands of scenarios to account for variability and produce a range of possible outcomes.
- Example: Estimating the economic impact of a trade policy by running simulations that account for fluctuations in global trade, domestic consumption, and inflation.
SayPro Build the Model
Once the variables and simulation framework are defined, the next step is to construct the model. This process typically involves the following steps:
SayPro Data Collection and Preprocessing
Gather data on key variables (e.g., demographic data, economic indicators, policy impacts) and preprocess it for use in the model. This may include:
- Cleaning the data to remove outliers or missing values.
- Normalizing or transforming variables for easier modeling.
SayPro Define Relationships Between Variables
Map out how different variables influence each other. For example:
- Economic growth might depend on investment, which is influenced by interest rates and government policy.
- Social behavior might be impacted by policy incentives, which change consumer choices over time.
SayPro Implement the Model Using Software
Depending on the chosen simulation framework, select appropriate software tools:
- System Dynamics: Tools like Vensim or Stella are commonly used for SDM.
- Agent-Based Modeling: Platforms like NetLogo or AnyLogic can be used.
- Monte Carlo Simulation: Tools like R or Python can be used to implement Monte Carlo simulations with specialized libraries (e.g.,
numpy
,simdkalman
).
SayPro Calibration and Validation
Once the model is constructed, itโs crucial to validate it by comparing its predictions to real-world data or outcomes from similar policies. Calibration might involve adjusting model parameters to better match observed results.
SayPro Run Simulations and Analyze Results
SayPro Baseline Scenarios:
Start with baseline simulations to observe the status quoโwhat would happen without the policy being implemented.
SayPro Policy Scenarios:
Run multiple scenarios with different policy interventions, such as:
- Changes in tax rates
- Introduction of new subsidies
- Shifts in regulation (e.g., environmental standards)
SayPro Sensitivity Analysis:
Analyze how sensitive the model is to changes in key assumptions or input variables. This can help identify which factors have the most significant influence on policy outcomes.
d. Outcome Measures:
Identify and track key outcome measures over time:
- Economic indicators (e.g., GDP growth, employment rate).
- Social changes (e.g., shifts in behavior, social equity).
- Environmental impacts (e.g., emissions reductions, resource conservation).
SayPro Interpret and Communicate Findings
Finally, interpret the results and communicate them to stakeholders, policymakers, or the public. Use visualizations (e.g., charts, graphs) and scenario analysis to present possible outcomes and their associated uncertainties.
- Scenario Comparisons: Show how different policy choices lead to varied outcomes (e.g., a high investment in renewable energy might result in faster emissions reduction compared to moderate investment).
- Risk Assessment: Highlight potential risks or unintended consequences that could arise from the policy.
Conclusion
Simulating the spread of policy impacts over time involves using sophisticated techniques such as System Dynamics, Agent-Based Modeling, and Monte Carlo Simulations to capture the complexities of economic, demographic, social, and environmental factors. By considering a wide range of variables and assumptions, policymakers can better understand the long-term effects of their policies, identify potential unintended consequences, and refine their strategies for more effective outcomes.