Your cart is currently empty!
SayPro Advanced Data Analysis: Apply advanced statistical techniques and tools, such as regression analysis and machine learning, to analyze data and assess the effectiveness of marketing campaigns in driving engagement, conversions, and ROI.
SayPro is a Global Solutions Provider working with Individuals, Governments, Corporate Businesses, Municipalities, International Institutions. SayPro works across various Industries, Sectors providing wide range of solutions.
Email: info@saypro.online Call/WhatsApp: + 27 84 313 7407
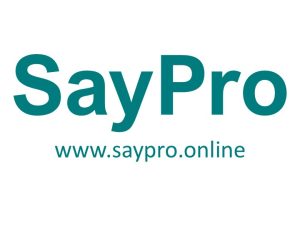
SayPro Advanced Data Analysis: Apply Advanced Statistical Techniques and Tools
Overview
To gain deeper insights into the effectiveness of marketing campaigns, SayPro utilizes advanced data analysis techniques, such as regression analysis and machine learning. These methods allow for a more granular understanding of how various marketing efforts influence key performance indicators (KPIs) like engagement, conversions, and return on investment (ROI). By leveraging these sophisticated tools, SayPro can assess the causal relationships between marketing strategies and business outcomes, identify trends, and optimize campaigns for greater impact.
Key Objectives
- Understand Campaign Impact: Identify how different marketing activities and touchpoints contribute to engagement, conversions, and ROI.
- Refine Marketing Strategies: Use data insights to improve decision-making around budget allocation, targeting, and messaging.
- Enhance Predictive Capabilities: Use advanced analytics techniques to predict future performance and optimize ongoing campaigns.
- Measure True ROI: Assess the true return on investment from marketing efforts, considering both direct and indirect factors.
Advanced Data Analysis Techniques
- Regression Analysis:
- Purpose: Determine the relationship between independent variables (marketing activities) and dependent variables (outcomes such as conversions or ROI).
- Types of Regression Used:
- Linear Regression: Used for understanding linear relationships between marketing efforts (e.g., ad spend) and performance metrics (e.g., conversions).
- Multiple Regression: Useful when evaluating the impact of multiple marketing factors on a particular outcome (e.g., how email campaigns, social media engagement, and paid ads together affect website traffic).
- Logistic Regression: Employed when the outcome variable is binary, such as whether a user converted or not (purchase, sign-up, etc.).
- Process:
- Identify the key marketing activities and performance metrics to be analyzed (e.g., paid ads spend, website traffic, conversions).
- Apply regression models to analyze the relationships and quantify how marketing efforts influence business outcomes.
- Use the model to predict the impact of future marketing activities and optimize strategies accordingly.
- Benefits:
- Uncover hidden patterns in the data, such as the most influential factors driving conversions or customer engagement.
- Provide statistical validation for decisions on where to allocate marketing resources for the highest impact.
- Allow for targeted adjustments to underperforming campaigns by focusing on key drivers of success.
- Machine Learning:
- Purpose: Leverage algorithms to uncover complex patterns in large datasets, providing more accurate insights and enabling predictive analysis.
- Common Machine Learning Models Used:
- Decision Trees: Identify decision rules to classify customer behavior or predict conversion likelihood based on historical data.
- Random Forests: Improve accuracy by combining multiple decision trees to assess which factors most influence conversions or ROI.
- Support Vector Machines (SVM): Used for classification tasks, such as segmenting audiences based on purchasing behavior or campaign responses.
- Clustering (e.g., K-means, Hierarchical): Segment customers based on their behavior or demographic data, helping to tailor marketing messages and strategies.
- Neural Networks: Used to analyze more complex relationships in the data, often employed for advanced tasks like predicting customer churn or lifetime value.
- Process:
- Feature Selection: Identify the relevant features (variables) that will be used for training the model, such as customer demographics, campaign details, and user behavior.
- Model Training: Train the machine learning model using historical data, adjusting parameters to improve prediction accuracy.
- Model Testing and Validation: Use unseen data (test set) to validate the model’s performance and ensure it generalizes well to new, unseen data.
- Prediction and Optimization: Apply the trained model to predict future campaign performance, customer behavior, and outcomes. This can help optimize targeting, personalize content, and improve resource allocation.
- Benefits:
- Automation: Automate the process of identifying patterns and predicting future trends, which can streamline decision-making.
- Real-time Optimization: Use real-time data to continuously update and improve machine learning models, enabling ongoing optimization of marketing campaigns.
- Personalization: Deliver more personalized marketing content by identifying unique customer segments and tailoring strategies to their behavior or preferences.
- Improved Forecasting: Provide accurate, data-driven forecasts about campaign performance, helping to set realistic expectations and inform strategic decisions.
- A/B Testing and Multivariate Testing:
- Purpose: Test variations of marketing campaigns to determine which performs best and optimize based on data.
- Process:
- For A/B Testing, test two variations of a campaign (e.g., different headlines, ad creatives, or call-to-action buttons) and measure which one drives more conversions.
- For Multivariate Testing, test multiple variables simultaneously to determine how different combinations of changes impact performance.
- Benefits:
- Provides concrete data to support decision-making, minimizing subjective judgments in campaign adjustments.
- Helps identify the most effective marketing elements and improve conversion rates by focusing on what resonates most with the target audience.
- Time Series Analysis:
- Purpose: Analyze trends over time to predict future performance and spot emerging patterns.
- Application:
- Assess how seasonal fluctuations, marketing campaigns, or external events affect performance metrics like sales or web traffic.
- Forecast future demand, conversions, or revenue based on historical trends.
- Benefits:
- Provides accurate, time-based predictions for key metrics, allowing for proactive strategy adjustments.
- Helps optimize campaign timing and resource allocation by predicting when demand for products or services will peak.
Key Metrics and KPIs Analyzed with Advanced Techniques
- Engagement Metrics:
- Click-through rates (CTR) for ads or emails.
- Social media engagement (likes, shares, comments).
- Time on site and pages per session (indicating content relevance).
- Customer lifetime value (CLV) and engagement duration.
- Conversion Metrics:
- Conversion rate: The percentage of visitors who complete a desired action (purchase, sign-up).
- Cost per acquisition (CPA): The cost of acquiring each customer or lead.
- Return on Ad Spend (ROAS): The revenue generated for every dollar spent on advertising.
- Customer retention rate and churn rate: Key indicators of customer loyalty and satisfaction.
- Return on Investment (ROI):
- Marketing ROI: Measures the total return relative to the marketing spend.
- Attribution Analysis: Assigns credit to various touchpoints in the customer journey to understand how each marketing channel contributes to conversions.
- Incrementality: Measures the additional impact of marketing activities beyond what would have occurred without them.
Process for Implementing Advanced Data Analysis
- Data Collection:
- Gather data from all relevant marketing channels and touchpoints (e.g., website, social media, email campaigns, CRM systems).
- Data Preprocessing:
- Clean and transform data to ensure accuracy and consistency. Handle missing values, outliers, and irrelevant data.
- Model Selection:
- Choose the appropriate statistical or machine learning models based on the marketing goals and data type.
- Model Training:
- Train the model using historical data, optimizing for key performance metrics (e.g., conversions, engagement).
- Evaluation and Validation:
- Assess the model’s accuracy and validate using test data to ensure reliability.
- Implementation:
- Apply the insights from the model to ongoing campaigns. Continuously monitor and adjust based on new data and performance feedback.
Conclusion
Advanced data analysis using techniques like regression analysis, machine learning, and time series analysis enables SayPro to assess the effectiveness of marketing campaigns with precision. By understanding the relationships between marketing activities and outcomes, SayPro can optimize strategies, maximize ROI, and improve customer engagement. These insights support data-driven decision-making and provide a competitive edge in the rapidly changing marketing landscape.
Leave a Reply
You must be logged in to post a comment.