Your cart is currently empty!
SayPro Data Analysis Reports:To summarize the findings from data analysis and highlight areas for improvement.
SayPro is a Global Solutions Provider working with Individuals, Governments, Corporate Businesses, Municipalities, International Institutions. SayPro works across various Industries, Sectors providing wide range of solutions.
Email: info@saypro.online Call/WhatsApp: + 27 84 313 7407
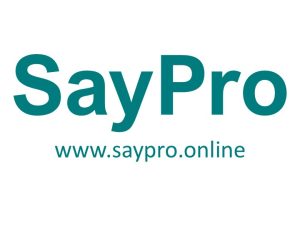
SayPro Data Analysis Reports: Summarizing Findings and Highlighting Areas for Improvement
Data analysis reports are essential for transforming raw data into actionable insights that inform decision-making and drive improvements. The goal of these reports is to provide a clear, concise summary of data analysis findings, identify trends, and highlight key areas for improvement in the organization’s processes, performance, or outcomes.
Below is a structured SayPro Data Analysis Report Template designed to ensure that all essential findings and insights are clearly communicated, and areas for improvement are effectively identified and addressed.
1. Title Section
- Report Title: “Data Analysis Report – [Specific Focus/Area]”
- Date: [Insert Date]
- Prepared By: [Name(s) of the author(s)]
- Version Number: [e.g., Version 1.0]
- Approval Status: [Pending, Approved, In Progress]
Purpose: This section provides basic information about the report, including its title, date of creation, version, and the author(s) responsible for the analysis.
2. Executive Summary
- Overview: A brief summary of the purpose of the analysis, key findings, and the areas of improvement identified. This section should provide a snapshot of the most important insights from the data.
- Key Findings: A high-level summary of the most significant trends or patterns observed in the data.
- Recommended Actions: A concise list of suggested next steps or improvements based on the findings.
Purpose: The executive summary allows stakeholders to quickly understand the key points of the report without needing to read the entire document. It highlights the most important aspects of the analysis and sets the stage for the more detailed sections that follow.
3. Introduction
- Objective of the Analysis: Clearly define the purpose of the data analysis. What questions were you trying to answer? What specific problem or opportunity was being addressed?
- Example: “This analysis aims to identify inefficiencies in the customer support process, specifically focusing on response times and ticket resolution rates.”
- Scope: Describe the scope of the data analysis, including the time period, data sources, and any limitations or constraints.
- Example: “The analysis covers customer support data from January 1, 2025, to March 31, 2025, and focuses on the number of tickets received, response times, and customer satisfaction scores.”
Purpose: The introduction helps set the context for the data analysis, ensuring that readers understand why the analysis was conducted and what the expected outcomes were.
4. Data Collection and Methodology
- Data Sources: List and describe the sources of data used in the analysis.
- Example: “The data was collected from the company’s Helpdesk system, customer satisfaction surveys, and support team reports.”
- Methodology: Outline the methods and techniques used to analyze the data (e.g., statistical analysis, regression analysis, trend analysis, etc.).
- Example: “Data was analyzed using time-series analysis to assess changes in ticket volume and response times over the past quarter. Regression models were applied to identify factors affecting customer satisfaction scores.”
- Data Quality Considerations: Briefly mention the quality of the data (e.g., completeness, accuracy) and any potential issues or biases.
- Example: “The data used was complete for the analysis period, but some customer satisfaction survey responses were missing or incomplete.”
Purpose: This section explains how the data was collected and analyzed, ensuring transparency and clarity regarding the methodology and any limitations in the data quality.
5. Key Findings
This section provides a detailed overview of the main insights derived from the data analysis. It should include both quantitative and qualitative findings, supported by visualizations such as charts, graphs, and tables.
- Trend Analysis: Identify key trends or patterns in the data over time.
- Example: “The average response time for support tickets has increased by 10% over the past three months.”
- Comparative Analysis: Compare data from different groups, periods, or other relevant categories.
- Example: “Tickets from high-priority customers have a 20% higher resolution time than those from low-priority customers.”
- Correlations or Causal Relationships: If applicable, highlight any correlations or relationships identified between variables.
- Example: “There is a strong positive correlation between ticket resolution time and customer satisfaction scores. A reduction in resolution time leads to a 15% increase in satisfaction.”
- Performance Metrics: Present key performance indicators (KPIs) that were analyzed, such as response times, resolution times, customer satisfaction scores, etc.
- Example: “The average customer satisfaction score is 3.8 out of 5, which represents a 5% decline from the previous quarter.”
Purpose: This section presents the core findings from the data analysis, providing stakeholders with a clear understanding of what the data reveals about the current state of operations or processes.
6. Areas for Improvement
Based on the findings, this section highlights specific areas where improvements are needed. It connects the data insights to actionable items, providing a foundation for future steps.
- Area 1: Response Time:
- Issue: The average response time for customer support tickets has exceeded the target by 12%.
- Suggested Improvement: “Implement a priority-based ticketing system to ensure high-priority tickets are handled first.”
- Area 2: Customer Satisfaction:
- Issue: Customer satisfaction has decreased by 5% in the past quarter, with slow ticket resolution being the leading cause.
- Suggested Improvement: “Provide additional training to support staff on faster ticket resolution techniques and improve knowledge base accessibility.”
- Area 3: Ticket Volume:
- Issue: There is a consistent increase in the volume of tickets, leading to backlogs.
- Suggested Improvement: “Implement an automated chatbot to handle basic customer inquiries, reducing the load on human agents.”
Purpose: This section directly links the data findings to areas of improvement, providing a clear action plan to address identified weaknesses.
7. Recommendations for Action
Based on the areas for improvement, this section provides detailed, specific recommendations for action. These should be aligned with the organization’s strategic goals and should be prioritized based on their potential impact.
- Recommendation 1: Implement a more efficient ticket categorization and prioritization system.
- Rationale: This will ensure high-priority tickets are addressed first, reducing overall response times.
- Recommendation 2: Invest in additional training for support staff to improve ticket resolution speed and accuracy.
- Rationale: This will improve customer satisfaction by reducing resolution time and improving the quality of responses.
- Recommendation 3: Introduce a new customer support software with advanced analytics capabilities to track performance metrics in real-time.
- Rationale: This will provide better insights into performance, allowing for more agile adjustments and continuous improvement.
Purpose: This section converts the analysis into actionable strategies, providing clear recommendations that are based on data and aimed at improving organizational performance.
8. Conclusion
- Summary of Findings: Recap the most significant insights from the analysis, highlighting the key areas for improvement.
- Next Steps: Outline the immediate next steps or actions that should be taken based on the report’s findings and recommendations.
- Call to Action: Encourage relevant stakeholders to take action and support the implementation of the recommendations.
Purpose: The conclusion wraps up the report, summarizing key insights and offering a clear path forward for stakeholders to follow.
9. Appendices and Supporting Data
- Raw Data: Attach or link to any raw data used in the analysis, if relevant and necessary for further reference.
- Visuals: Include charts, graphs, tables, or other visual aids that support the findings and make complex data easier to understand.
- Methodological Notes: Provide any additional details on the methods used for analysis, such as formulas or statistical models.
Purpose: This section provides transparency and allows stakeholders to verify and explore the underlying data and methods in more detail.
Example of Key Findings
- Trend Analysis:
- Average Response Time (January 2025 – March 2025): 4.5 hours (Increase from 3.8 hours in Q4 2024)
- Customer Satisfaction Score: 3.8/5 (Decline of 5% from last quarter)
- Comparative Analysis:
- Response Time for High-Priority Tickets: 6 hours
- Response Time for Low-Priority Tickets: 3 hours
- Correlations:
- Strong correlation (r = 0.85) between ticket resolution time and customer satisfaction.
Conclusion
The SayPro Data Analysis Report provides a structured way to present data findings, identify improvement areas, and recommend actionable solutions. By following this template, SayPro can ensure that data is analyzed effectively and that the insights derived from the data are used to make informed decisions that drive performance improvements.
Leave a Reply
You must be logged in to post a comment.