Your cart is currently empty!
SayPro Data Analysis: Use statistical tools and techniques to analyze data for emerging patterns, trends, and key insights.
SayPro is a Global Solutions Provider working with Individuals, Governments, Corporate Businesses, Municipalities, International Institutions. SayPro works across various Industries, Sectors providing wide range of solutions.
Email: info@saypro.online Call/WhatsApp: + 27 84 313 7407
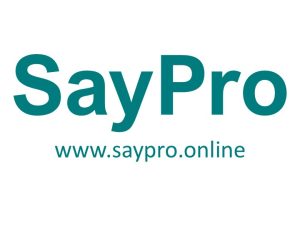
SayPro Data Analysis: Using Statistical Tools and Techniques to Analyze Data for Emerging Patterns, Trends, and Key Insights
Data analysis is a critical step in transforming raw data into valuable insights that can guide decision-making, strategic planning, and business improvements. For SayPro, this process involves applying statistical tools and techniques to identify patterns, trends, and key insights from both internal and external data sources. The goal is to extract actionable insights that can improve organizational performance, optimize processes, and enhance decision-making across all levels of the business.
Key Steps in SayPro’s Data Analysis Process:
- Data Preparation:
- Data Cleaning: Before any analysis can take place, it is crucial to clean the data. This involves removing errors, handling missing values, dealing with outliers, and standardizing formats. Clean data ensures that the analysis is accurate and reliable.
- Data Transformation: Transforming data into a usable format (e.g., normalization, categorization, or aggregation) is key to preparing it for analysis. This may involve structuring data into time series, categorizing variables, or performing calculations to create new variables.
- Exploratory Data Analysis (EDA): EDA is a process of visually and statistically exploring the data to understand its structure, detect any anomalies, and form hypotheses about what patterns might exist.
- Summary Statistics:
- Measures of Central Tendency: Calculating the mean, median, and mode to understand the central value of the data.
- Measures of Dispersion: Calculating variance, standard deviation, and interquartile ranges to assess the spread or variability of the data.
- Visualizations:
- Histograms & Bar Charts: These help to visualize the distribution of individual variables and understand how data points are spread across categories or intervals.
- Box Plots: A powerful tool to identify outliers and understand the distribution of data within quartiles.
- Scatter Plots: Used to visualize relationships between two variables, helping to identify any correlation or patterns.
- Summary Statistics:
- Statistical Tools and Techniques for Data Analysis:a. Descriptive Statistics:
- Descriptive statistics summarize and describe the features of the data. This includes calculating the mean, median, mode, variance, and standard deviation.
- It helps to understand the distribution, spread, and central tendency of the data, providing a snapshot of key metrics like sales, performance scores, or customer satisfaction ratings.
- Hypothesis Testing: Hypothesis tests help determine whether observed patterns or trends in data are statistically significant or if they occurred by chance. Common tests include:
- t-tests (for comparing means between two groups).
- ANOVA (for comparing means between three or more groups).
- Chi-Square Test (for categorical variables).
- Confidence Intervals: These intervals provide a range in which the true population parameter lies with a certain level of confidence (e.g., 95%). This helps managers understand the level of uncertainty in the analysis.
- Regression analysis is used to examine the relationship between dependent and independent variables. For example, a company might use regression to predict sales based on factors like marketing spend, seasonality, or customer demographics.
- Linear Regression: Models the relationship between two continuous variables, such as how advertising spend affects sales.
- Multiple Regression: Uses multiple independent variables to predict a dependent variable. For example, predicting customer satisfaction based on service quality, response time, and pricing.
- Logistic Regression: Used when the dependent variable is categorical (e.g., predicting customer churn: “Yes” or “No”).
- Pearson Correlation: Measures the linear relationship between two continuous variables. For example, understanding how closely the number of customer complaints is related to product returns.
- Spearman’s Rank Correlation: Used when data is non-parametric or when the relationship between variables is not linear.
- Correlation analysis helps identify the strength and direction of relationships, guiding decision-makers on where changes or improvements may have the greatest impact.
- Trend Analysis: Identifies underlying trends in the data, such as increasing sales or decreasing customer satisfaction over time.
- Seasonal Decomposition: Decomposes time series data into trend, seasonal, and residual components. This can help predict future trends and optimize resource allocation during peak or off-peak periods.
- Moving Averages: Used to smooth out short-term fluctuations and highlight longer-term trends.
- K-Means Clustering: An unsupervised learning algorithm that groups data points into clusters based on similarity. For example, customer segmentation based on purchasing behavior.
- Hierarchical Clustering: Used to build a tree of clusters, providing insights into how different data points group together.
- Segmentation Analysis: Helps to identify distinct groups within the data (e.g., high-value customers vs. low-value customers), which is valuable for targeted marketing and personalized services.
- Decision Trees: A flowchart-like structure that helps classify data based on decisions. For instance, predicting whether a customer will buy a product based on demographic data and past behavior.
- Random Forests: An ensemble of decision trees, which improves the accuracy of predictions by combining the results of multiple trees.
- Support Vector Machines (SVM): A supervised learning algorithm used for classification tasks. It is helpful in classifying customers into categories like “Likely to churn” or “Highly satisfied.”
- Pattern Recognition and Trend Detection:
- Market Basket Analysis: Often used in retail, this technique identifies relationships between products that are frequently bought together. For example, it can help businesses offer product bundles or recommend complementary items.
- Association Rule Mining: This technique identifies patterns in large datasets and establishes relationships between variables. For example, it can find that customers who purchase a certain type of product are also likely to purchase another product.
- Anomaly Detection: Statistical techniques (e.g., Z-scores or machine learning algorithms) are used to identify outliers or anomalies that could indicate errors or significant trends (e.g., a sudden spike in website traffic or a drop in customer satisfaction).
- Data Visualization:
- Dashboards: Tools like Power BI, Tableau, or Google Data Studio can be used to create dynamic dashboards that provide an interactive and real-time overview of key performance metrics (e.g., sales trends, marketing performance, or customer feedback).
- Charts and Graphs: Visualizing data through pie charts, line charts, bar graphs, and scatter plots helps decision-makers quickly understand the insights from the analysis.
- Heatmaps: Visual representations of data in matrix form, where color gradients indicate the strength of the relationship between variables (useful in marketing analysis to understand where most engagement occurs on websites or social media).
- Predictive Analytics and Forecasting: Predictive analytics uses historical data to predict future outcomes. Statistical models, machine learning algorithms, and time series analysis techniques are used to forecast trends such as:
- Sales Forecasting: Predicting future sales based on historical sales data, marketing efforts, seasonality, and external factors like market conditions or economic shifts.
- Customer Churn Prediction: Using historical customer data to predict which customers are likely to stop using a product or service. This allows businesses to take proactive steps in retaining customers.
- Demand Forecasting: Predicting the future demand for a product or service, helping businesses optimize inventory levels and resource allocation.
Key Insights from Data Analysis for SayPro:
- Emerging Patterns:
- Identifying common behaviors or trends in customer engagement (e.g., an increase in mobile usage for online purchases, or seasonal spikes in demand for certain products).
- Recognizing shifts in customer preferences or market conditions that may inform future business strategies.
- Operational Efficiencies:
- Identifying inefficiencies in business processes or operations by analyzing performance data (e.g., delays in project timelines, overuse of resources, or low employee productivity).
- Highlighting areas for improvement in service delivery, customer support, or supply chain management.
- Customer Insights:
- Discovering customer segmentation, allowing SayPro to tailor its marketing and sales strategies to specific groups.
- Understanding the factors that drive customer satisfaction, loyalty, or churn, enabling better customer retention strategies.
- Sales and Revenue Trends:
- Analyzing past sales data to predict future sales, optimize pricing strategies, and identify cross-selling or upselling opportunities.
- Understanding the impact of external factors (e.g., economic conditions or competitor actions) on sales performance.
- Marketing Effectiveness:
- Assessing the ROI of various marketing campaigns by analyzing performance data (e.g., engagement rates, lead conversions, and cost-per-click) to optimize future marketing efforts.
- Determining the most effective channels for customer acquisition and retention.
Conclusion:
Data analysis is essential for SayPro to gain actionable insights that drive strategic decision-making and continuous improvement. By utilizing statistical tools and techniques such as regression analysis, hypothesis testing, time series analysis, and predictive modeling, SayPro can identify emerging patterns, detect trends, and extract valuable insights. These insights can inform a variety of business strategies, from marketing to customer retention and resource allocation, ultimately helping SayPro achieve its organizational goals.
Leave a Reply
You must be logged in to post a comment.