Your cart is currently empty!
SayPro Data Quality Assurance Reports: A report ensuring the accuracy and reliability of the data, including validation checks and verification procedures.
SayPro is a Global Solutions Provider working with Individuals, Governments, Corporate Businesses, Municipalities, International Institutions. SayPro works across various Industries, Sectors providing wide range of solutions.
Email: info@saypro.online Call/WhatsApp: + 27 84 313 7407
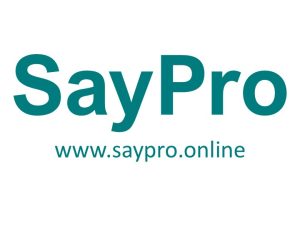
SayPro Data Quality Assurance Reports
Objective:
The goal of the SayPro Data Quality Assurance Reports is to ensure that all data used for analysis, reporting, and decision-making within the organization is accurate, reliable, and consistent. This report will detail the validation checks, verification procedures, and quality control mechanisms implemented to maintain high data integrity across all departments.
1. Report Structure Overview
A. Executive Summary
- Overview of Data Quality Efforts: A brief summary of the data quality initiatives, highlighting the scope, objectives, and importance of maintaining high data integrity.
- Key Findings: A snapshot of the results of the quality assurance checks, including any significant issues identified, and the overall state of data reliability.
- Actionable Insights: High-level recommendations for further improving data quality based on the findings.
B. Data Quality Framework
- Data Collection Standards: An overview of the standards and procedures for data collection, ensuring that data is captured in a consistent and accurate manner across departments.
- Standardized Data Formats: Consistency in data entry formats to avoid discrepancies (e.g., consistent date formats, currency symbols, etc.).
- Data Sources: List of primary data sources used across SayPro and how they are integrated into reporting systems.
C. Data Validation Procedures
- Validation Checks: A detailed explanation of the validation checks conducted to ensure data is accurate.
- Data Range Validation: Ensuring data entries are within acceptable ranges (e.g., sales amounts, quantities, financial figures).
- Consistency Checks: Ensuring that related data points match (e.g., verifying that total sales match individual product sales).
- Uniqueness Checks: Identifying and resolving duplicates within datasets (e.g., multiple records for the same customer or transaction).
- Completeness Checks: Identifying and addressing missing or incomplete data entries.
D. Data Verification Processes
- Source Verification: Verification of data sources to ensure that they are reliable and consistent.
- Third-party Data: Confirming the accuracy of external data sources used (e.g., market data, customer feedback).
- Internal Data: Cross-checking internal data entries against the original source to verify their authenticity.
- Cross-Department Verification: Ensuring that data shared between departments (e.g., sales and finance) matches and aligns.
- Example: Sales figures from the sales team should match financial reports from the finance department.
2. Data Quality Metrics and Analysis
A. Key Data Quality Metrics
- Accuracy: Percentage of data entries that are accurate and match the source data.
- Completeness: The proportion of data that is complete and free from missing or incomplete fields.
- Consistency: The degree to which data values are consistent across different systems and sources.
- Timeliness: The ability to collect and update data in a timely manner for decision-making purposes.
- Uniqueness: The extent to which data entries are unique, without duplication or overlap.
B. Data Quality Issues Identified
- Accuracy Issues: Detailed documentation of any inaccuracies found in the data, including the type of error (e.g., wrong customer information, incorrect transaction amounts) and the corrective actions taken.
- Completeness Gaps: Instances where data is incomplete, such as missing values or records that were not properly captured. Provide the reasons and actions taken to fill these gaps.
- Inconsistencies: Descriptions of any inconsistencies found across different systems or data sources, and how these were resolved.
- Duplication Problems: Instances where duplicate entries were found, and actions taken to eliminate them.
C. Data Quality Trends
- Trends in Data Quality: A summary of how data quality has evolved over the reporting period. This can include improvements, consistency, or areas where issues have increased.
- Root Cause Analysis: In cases of recurring data quality issues, perform a root cause analysis to determine if these are due to system limitations, human error, or other factors.
3. Data Quality Assurance Tools and Technologies
A. Tools and Software
- Data Cleansing Tools: Overview of the tools used for data cleansing and validation (e.g., data validation scripts, data integrity software).
- Automated Validation Systems: Explanation of automated systems that monitor data quality in real-time or on a scheduled basis (e.g., dashboards that track data accuracy or consistency).
- Manual Audits: Details on any manual verification processes carried out by employees or departments to complement automated checks.
B. Process Improvements
- Process Automation: Recommendations for automating data quality assurance tasks to reduce manual effort and improve efficiency.
- System Upgrades: Suggestions for system improvements (e.g., software upgrades or database restructuring) to better support data quality management.
4. Data Quality Improvements and Recommendations
A. Key Areas for Improvement
- Training: Offering additional training for employees on how to correctly enter, manage, and validate data. This could include workshops on the importance of data quality and the impact on organizational performance.
- Data Collection Processes: Streamlining data collection processes across departments to minimize errors and improve accuracy from the start.
- Integration Between Systems: Improving the integration between disparate systems (e.g., CRM, ERP, HR systems) to ensure that data is consistent across the board.
B. Proposed Solutions
- Enhanced Data Validation: Propose new or improved validation techniques to ensure higher data quality, such as stricter validation rules or advanced AI-powered data analysis.
- Standardization of Data: Recommendations for more standardized data entry procedures across all departments, ensuring uniformity in how data is recorded and shared.
C. Long-Term Data Quality Strategy
- Ongoing Monitoring: Implementing continuous monitoring of data quality to detect and resolve issues as they arise.
- Data Governance Framework: Establishing a comprehensive data governance policy that sets clear rules for data management, including responsibilities, standards, and procedures to ensure long-term data integrity.
5. Compliance with Data Regulations
- Legal Compliance: Ensuring that data management and quality assurance procedures are in line with applicable data protection laws (e.g., GDPR, CCPA).
- Privacy and Security: Overview of how data quality assurance measures align with privacy and security regulations to protect sensitive and personal information.
6. Conclusion and Next Steps
- Summary of Findings: A recap of the data quality assurance activities and results, highlighting improvements made and areas still requiring attention.
- Actionable Next Steps: Concrete steps to address identified issues, optimize the data quality assurance processes, and implement long-term improvements.
- Stakeholder Engagement: The next steps will include communicating the findings and recommended actions to all relevant stakeholders for feedback and further refinement.
Objective: The SayPro Data Quality Assurance Reports will act as a comprehensive framework for managing and enhancing data quality, ensuring that all data used for decision-making is reliable, accurate, and aligned with organizational standards. This will help SayPro to make better-informed decisions and maintain the trust of both internal and external stakeholders.
Leave a Reply
You must be logged in to post a comment.