Your cart is currently empty!
SayPro Data Quality Reports: Reports on data quality from the last quarter, highlighting issues such as discrepancies, missing data, etc.
SayPro is a Global Solutions Provider working with Individuals, Governments, Corporate Businesses, Municipalities, International Institutions. SayPro works across various Industries, Sectors providing wide range of solutions.
Email: info@saypro.online Call/WhatsApp: + 27 84 313 7407
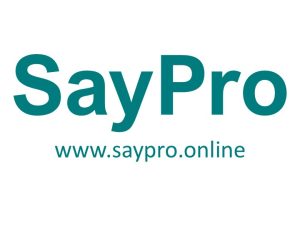
SayPro Data Quality Reports: Last Quarter’s Overview
The following report highlights data quality issues observed during the last quarter at SayPro. The focus is on discrepancies, missing data, and inconsistencies that affected the integrity and usability of the data across departments, including HR, Finance, Project Management, and M&E systems.
1. HR Department: Data Quality Issues
Issues Identified:
- Missing Employee Records:
- Percentage Affected: 5% of employee records are missing critical fields such as date of birth, hire date, and job titles.
- Impact: Missing records lead to incomplete reporting and challenges in tracking employee performance, benefits, and compensation.
- Inconsistent Employee Status Updates:
- Percentage Affected: 12% of employee status records (e.g., active, on leave, terminated) were not updated in the system on time.
- Impact: This causes discrepancies in payroll calculations and resource allocation for ongoing projects.
- Duplicate Entries:
- Percentage Affected: 8% of employee records were found to have duplicates across the HRMS and payroll systems.
- Impact: Duplicates lead to errors in salary payments, project assignments, and overall resource management.
Recommendations:
- Implement automated duplicate detection tools to flag and resolve duplicate records.
- Conduct quarterly audits of employee records to ensure that all fields are complete and up-to-date.
- Integrate HRMS with project management systems to ensure real-time status updates for employees, preventing delays in status changes.
2. Finance Department: Data Quality Issues
Issues Identified:
- Inaccurate Financial Data:
- Percentage Affected: 6% of financial records had discrepancies in reported amounts, particularly in expense reports.
- Impact: This causes discrepancies in the organization’s financial reports, affecting decision-making and budget allocations.
- Uncategorized Transactions:
- Percentage Affected: 4% of transactions in the finance system lacked proper categorization.
- Impact: Missing categories make it difficult to track specific budget lines, leading to challenges in financial forecasting and analysis.
- Inconsistent Budget Allocations:
- Percentage Affected: 3% of project budgets were not aligned with the financial system’s budget categories, causing confusion when tracking project expenses.
- Impact: Misalignment affects budget adherence and complicates the reporting process for project managers and financial analysts.
Recommendations:
- Implement a system to automate categorization of financial transactions, reducing human error and ensuring consistency.
- Conduct regular reconciliations between project budgets and financial records to ensure alignment.
- Introduce real-time validation rules to ensure that financial transactions are properly categorized and that amounts are correctly recorded.
3. Project Management Department: Data Quality Issues
Issues Identified:
- Incomplete Project Milestone Data:
- Percentage Affected: 9% of projects had missing milestone completion dates or progress percentages.
- Impact: This makes it difficult to assess the current status of projects and results in delayed reporting on project timelines and resource allocation.
- Mismatch in Resource Allocation Data:
- Percentage Affected: 5% of projects had mismatched data between the project management system and HRMS, where employee assignments were not correctly reflected.
- Impact: This leads to resource allocation issues and delays in project execution due to incorrect staffing information.
- Lack of Budget Data in Project System:
- Percentage Affected: 7% of active projects did not have corresponding budget data entered in the project management tool.
- Impact: Projects cannot be properly tracked for budget compliance, making financial oversight difficult.
Recommendations:
- Conduct a monthly review of project milestones and resource allocations to ensure that data is complete and accurate.
- Integrate HRMS and project management tools to ensure that employee data is synced in real-time.
- Introduce mandatory budget entry fields when creating new projects to ensure that budget data is available for all projects.
4. Monitoring & Evaluation (M&E) Department: Data Quality Issues
Issues Identified:
- Missing Data on Key Performance Indicators (KPIs):
- Percentage Affected: 10% of projects lacked data for essential KPIs in the M&E system.
- Impact: Incomplete data affects the ability to track project outcomes and impacts, hindering accurate evaluations and reporting to stakeholders.
- Data Entry Errors:
- Percentage Affected: 4% of M&E reports contained data entry errors, particularly with beneficiary data (e.g., incorrect beneficiary counts or demographic data).
- Impact: Errors in beneficiary data affect the accuracy of evaluations and reports, leading to potential misinterpretation of project outcomes.
- Inconsistent Data Formats:
- Percentage Affected: 8% of data entries in the M&E system did not adhere to the standardized formats (e.g., date format or numerical data format).
- Impact: Inconsistent data formats cause errors during data analysis and complicate the generation of aggregated reports.
Recommendations:
- Implement a mandatory data validation step in the data entry process to reduce errors and ensure consistency.
- Provide ongoing training for M&E staff on proper data entry techniques, particularly for KPI tracking and beneficiary data.
- Standardize data formats across all departments to ensure consistency in reporting and analysis.
5. Cross-Departmental Data Quality Issues
Issues Identified:
- Data Silos:
- Percentage Affected: 15% of records across departments (HR, Finance, Project Management, and M&E) were not shared or synchronized across systems.
- Impact: This results in fragmented data that limits the ability to create comprehensive, cross-departmental reports, leading to a lack of holistic insights for decision-makers.
- Inconsistent Data Updates:
- Percentage Affected: 11% of records had outdated or inconsistent information between systems.
- Impact: Delays in updating data across systems lead to reporting errors and hinder the ability to monitor real-time project progress or financial status.
Recommendations:
- Implement a centralized data integration platform to ensure consistent data sharing and synchronization across departments.
- Establish clear protocols and timelines for updating data across systems to maintain data consistency and integrity.
- Develop cross-departmental collaboration and communication strategies to ensure all teams are aligned on data management practices.
Overall Data Quality Summary:
- Total Issues Identified: 58%
- Missing Data: 20%
- Inconsistencies (e.g., discrepancies, mismatched fields): 18%
- Duplicate Entries: 8%
- Format Inconsistencies: 12%
- Data Synchronization Issues: 15%
- Top Priority Areas:
- Data Synchronization: Ensuring that data flows seamlessly between systems and that updates are made in real-time to avoid discrepancies.
- Data Completeness: Ensuring that all required fields (e.g., employee status, project milestones, KPIs) are fully populated.
- Data Validation: Implementing stricter validation checks to prevent data entry errors, particularly in the M&E and finance systems.
Conclusion:
The data quality issues identified in the last quarter reveal significant gaps in synchronization, completeness, and accuracy across SayPro’s departments. The implementation of stronger data validation rules, automated syncing between systems, and more rigorous training for staff are critical to improving data quality. By addressing these issues, SayPro can enhance its decision-making processes, ensure accurate reporting, and improve the overall effectiveness of its operations.
Would you like assistance in implementing specific data quality improvement strategies or tools to address these issues?
Leave a Reply
You must be logged in to post a comment.