Your cart is currently empty!
SayPro Flag and report any data anomalies in at least 20% of the sampled data, focusing on common issues such as incorrect entries or missing values.
SayPro is a Global Solutions Provider working with Individuals, Governments, Corporate Businesses, Municipalities, International Institutions. SayPro works across various Industries, Sectors providing wide range of solutions.
Email: info@saypro.online Call/WhatsApp: + 27 84 313 7407
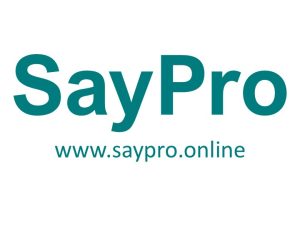
SayPro: Flag and Report Data Anomalies in At Least 20% of Sampled Data
Objective: To ensure that SayPro’s data is clean and reliable, this process focuses on flagging and reporting any data anomalies found in at least 20% of the sampled data. Common issues include incorrect entries, missing values, and inconsistencies that could affect decision-making and strategy development.
Step-by-Step Process for Flagging and Reporting Data Anomalies
1. Define the Scope of the Data Samples
To evaluate data quality, at least 200 data points should be sampled from each of the following high-priority areas:
- Marketing Data (e.g., campaign performance, ad spend, conversion rates)
- User Engagement Metrics (e.g., page views, session duration, social shares)
- Sales Data (e.g., revenue, total orders, average transaction value)
2. Establish Criteria for Identifying Data Anomalies
Create a set of standard criteria for flagging anomalies in the data samples. Common data quality issues to look for include:
- Incorrect Entries: Data that is inaccurate, inconsistent, or doesn’t adhere to established standards (e.g., invalid numbers, misformatted dates).
- Missing Values: Data points that should be present but are missing (e.g., empty fields, unreported metrics).
- Outliers: Data points that fall far outside the expected range and may indicate errors (e.g., unusually high or low conversion rates).
- Inconsistent Formatting: Data entries that don’t follow the established format or naming conventions (e.g., inconsistent use of date formats or inconsistent campaign names).
- Duplicate Data: Repeated data entries that lead to inflated metrics or reporting inaccuracies (e.g., multiple entries for the same sale or user interaction).
- Data Mismatches: Discrepancies between related data points (e.g., mismatched customer IDs, incorrect product codes).
3. Sampling Process
- Sample Size: Randomly select a minimum of 200 data points from each of the three data sources: marketing data, user engagement metrics, and sales data.
- Sample Representation: Ensure that the sample includes a variety of time periods, metrics, and data categories to get a comprehensive view of the data quality.
4. Identify and Flag Anomalies
During the review of the sampled data, identify anomalies based on the established criteria. For each data source, flag any data points that exhibit the following:
- Incorrect Entries: Flag entries that are incorrect or don’t match the expected format. For example:
- Marketing Data: Incorrect campaign names, misreported impressions or clicks, ad spend figures that don’t match actual financial records.
- User Engagement Metrics: Incorrect session duration (e.g., unusually high or low), invalid user ID entries, misattributed social media shares.
- Sales Data: Incorrect revenue values, missing or incorrect product codes, discrepancies between total sales and recorded transactions.
- Missing Values: Flag any missing or empty fields. For example:
- Marketing Data: Missing conversion rates, unreported ad spend data.
- User Engagement Metrics: Missing session duration, absent social media engagement data.
- Sales Data: Missing total revenue or order values, incomplete customer data.
- Outliers: Flag data points that fall outside expected ranges. For example:
- Marketing Data: Conversion rates that are unusually high or low (e.g., a conversion rate of 300%).
- User Engagement Metrics: Session duration values that are disproportionately long or short (e.g., 0-second sessions or sessions lasting several days).
- Sales Data: Orders with unusually large or small values (e.g., a $100,000 sale in a product category that typically sells for $50).
- Inconsistent Formatting: Flag any data points that don’t adhere to established formatting rules or naming conventions. For example:
- Marketing Data: Campaign names that use different formats (e.g., “Spring Sale 2025” vs. “Spring2025Sale”).
- User Engagement Metrics: Date formatting inconsistencies (e.g., “MM-DD-YYYY” vs. “YYYY-MM-DD”).
- Sales Data: Product codes listed with different formats or missing standard prefixes.
- Duplicate Data: Flag any data points that appear more than once in the dataset. For example:
- Marketing Data: Duplicate entries for the same campaign or ad.
- User Engagement Metrics: Duplicate session entries for the same user.
- Sales Data: Multiple entries for the same sale.
- Data Mismatches: Flag instances where related data points don’t match up. For example:
- Marketing Data: Discrepancies between ad spend and impressions (e.g., high impressions with no corresponding ad spend).
- User Engagement Metrics: Mismatched user IDs across different platforms.
- Sales Data: Mismatched customer IDs or missing product information in orders.
5. Document the Anomalies
For each flagged anomaly, create a report documenting the following information:
- Data Source: Identify which data source the anomaly is from (e.g., marketing, user engagement, sales).
- Data Points: Provide a description of the flagged data point (e.g., “Conversion rate of 300% for Campaign A”).
- Type of Anomaly: Specify the type of anomaly identified (e.g., “Missing Value,” “Outlier,” “Incorrect Entry”).
- Impact: Briefly explain the potential impact of the anomaly on decision-making or performance evaluation (e.g., “Incorrect conversion rate reporting could lead to misinformed campaign adjustments”).
- Suggested Action: Recommend corrective actions for resolving the anomaly (e.g., “Review tracking setup for Campaign A” or “Update data entry processes”).
6. Calculate the Percentage of Anomalies
Calculate the percentage of flagged anomalies in the total sample. For example, if you sampled 200 data points and flagged 40 anomalies, the percentage would be: Percentage of anomalies=(Number of anomalies flaggedTotal data points sampled)×100\text{Percentage of anomalies} = \left( \frac{\text{Number of anomalies flagged}}{\text{Total data points sampled}} \right) \times 100
For a goal of flagging anomalies in at least 20% of the sampled data, you would need to flag at least 40 anomalies in the 200 data points.
7. Generate a Data Quality Assessment Report
Prepare a comprehensive report summarizing the findings from the flagged anomalies. This report should include:
- Overview: A summary of the data sources and the sampling process.
- Anomaly Breakdown: A detailed list of all flagged anomalies, categorized by type (e.g., incorrect entries, missing values, outliers).
- Impact Assessment: An analysis of how these anomalies could potentially affect business decisions, marketing strategies, and sales performance.
- Recommendations: Actionable steps to resolve the issues, such as revising data entry guidelines, improving tracking mechanisms, or implementing data validation tools.
8. Collaborate with Relevant Teams for Resolution
Once the anomalies have been flagged and reported, work with the respective teams (e.g., marketing, IT, sales) to take corrective actions:
- Data Entry Teams: Implement stricter validation and review processes for data entry.
- Marketing Team: Review campaign tracking and ensure consistent reporting.
- Sales Team: Validate CRM and order entry systems to eliminate duplicate or incorrect data.
- IT and Development Teams: Work to identify and fix any tracking issues or data collection bugs that may be causing anomalies.
Example Report of Flagged Data Anomalies
Marketing Data
- Anomaly Type: Missing Value
- Data Point: Conversion rate for “Spring Sale 2025” campaign is missing.
- Impact: This missing data could lead to inaccurate ROI calculations and decision-making regarding the success of the campaign.
- Action: Review the tracking system for the “Spring Sale 2025” campaign and ensure that all metrics are properly recorded.
User Engagement Metrics
- Anomaly Type: Outlier
- Data Point: Session duration of 10,000 seconds recorded for a user (likely an error).
- Impact: An incorrect session duration could distort engagement metrics and lead to improper user experience optimization.
- Action: Investigate tracking setup to correct session time anomalies.
Sales Data
- Anomaly Type: Duplicate Data
- Data Point: The same customer purchase order (Order ID: 123456) appears twice in the CRM system.
- Impact: Duplicate entries may overestimate revenue and lead to inaccurate sales performance reporting.
- Action: Review order entry procedures to identify and prevent duplicate entries in the future.
Conclusion
By flagging and reporting anomalies in at least 20% of the sampled data, SayPro ensures that data quality issues are detected and addressed early. This process helps maintain the integrity of key data sources and provides accurate information for decision-making, ultimately improving the reliability of marketing, user engagement, and sales strategies.
Leave a Reply
You must be logged in to post a comment.