Your cart is currently empty!
SayPro Provide a 100 process for conducting regular data assessments for ensuring data accuracy.
SayPro is a Global Solutions Provider working with Individuals, Governments, Corporate Businesses, Municipalities, International Institutions. SayPro works across various Industries, Sectors providing wide range of solutions.
Email: info@saypro.online Call/WhatsApp: + 27 84 313 7407
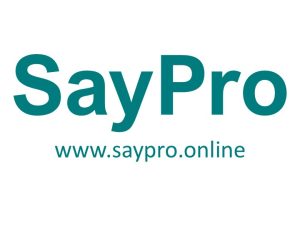
SayPro: 100-Step Process for Conducting Regular Data Assessments to Ensure Data Accuracy
Ensuring data accuracy is critical for decision-making, reporting, and strategic planning across all departments at SayPro. Below is a detailed 100-step process for conducting regular data assessments to maintain the highest standards of data accuracy.
Phase 1: Preparation for Data Assessment (Steps 1–20)
- Identify the Key Data Sources
- List all the primary data sources (e.g., CRM systems, marketing platforms, user engagement systems, sales data).
- Define Data Accuracy Standards
- Establish clear guidelines for what constitutes accurate data, including format consistency, completeness, and timeliness.
- Develop Data Quality Metrics
- Define measurable indicators for data accuracy (e.g., error rate, data consistency, timeliness of updates).
- Set Assessment Frequency
- Determine how often assessments will be conducted (e.g., weekly, monthly, quarterly).
- Form a Data Quality Team
- Assemble a team of data analysts, M&E specialists, and subject matter experts to oversee the data assessment process.
- Create Data Assessment Checklist
- Develop a comprehensive checklist that covers all aspects of data quality: completeness, consistency, accuracy, timeliness, and relevance.
- Review Existing Data Handling Procedures
- Analyze how data is collected, stored, and processed within various departments to understand where issues may arise.
- Identify Data Accuracy Risks
- Assess potential risks to data accuracy, such as human error in data entry or system integration issues.
- Develop Data Validation Rules
- Create automated or manual rules that will be used to check data validity during the assessment process.
- Establish Data Collection Guidelines
- Ensure there are clear guidelines for all teams involved in data entry, detailing formatting, data entry processes, and data storage.
- Plan for Data Access and Permissions
- Ensure all data collectors and reviewers have the appropriate access to the data they need to assess and validate.
- Document Data Workflow Processes
- Map out the workflow for how data is collected, processed, and stored to ensure understanding and consistency.
- Select Tools for Data Assessment
- Choose appropriate tools or software that can automate data quality checks, such as data validation tools, error detection software, and reporting tools.
- Communicate Data Assessment Plan to Teams
- Share the data assessment plan with relevant teams, so they understand their role in ensuring data accuracy.
- Set Up a Timeline for Assessment
- Define the timeline for completing the data assessment, ensuring ample time to review all necessary data points.
- Prepare Data Assessment Documentation
- Create templates or forms for documenting the findings of the data assessment, including any issues or anomalies.
- Allocate Resources for Data Assessment
- Ensure that there are sufficient resources, including time and personnel, to carry out a thorough assessment.
- Review Historical Data Quality Reports
- Analyze past data quality reports to understand trends, issues, and improvements that have already been made.
- Create a Data Anomaly Tracking System
- Develop a system to track anomalies or issues identified in previous assessments to ensure they are addressed in subsequent assessments.
- Establish Clear Reporting Structures
- Ensure there is a clear structure for reporting findings from the data assessment, including who needs to be informed of issues and how they will be addressed.
Phase 2: Data Sampling and Analysis (Steps 21–40)
- Determine Sampling Size
- Define the size and scope of data samples for the assessment, ensuring it’s large enough to represent the entire dataset.
- Select Data Sets for Review
- Choose a representative sample of datasets from various departments, ensuring that all key sources are covered.
- Perform Random Sampling
- Randomly select data entries for review to avoid bias and ensure a comprehensive assessment.
- Assess Data Completeness
- Check if all required data fields are filled out and if any entries are missing or incomplete.
- Check for Duplicate Entries
- Review the data to ensure that there are no duplicate entries or redundancy that could lead to incorrect conclusions.
- Validate Data Consistency
- Ensure that data is consistent across different systems and platforms, checking for discrepancies between similar data sources.
- Review Data Formatting
- Verify that all data conforms to the prescribed formats (e.g., date formats, numerical ranges, currency symbols).
- Assess Timeliness of Data
- Check whether the data is up-to-date, ensuring that outdated information has been replaced or updated accordingly.
- Cross-Reference with External Sources
- Compare internal data with external sources to verify its accuracy, especially for key data points like customer information or market trends.
- Look for Trends and Patterns
- Analyze data for unusual trends or patterns that may indicate errors or inaccuracies.
- Identify Outliers
- Detect any data outliers that may be due to errors, such as values that fall outside of expected ranges.
- Perform Validation Checks
- Run predefined validation checks on the data, such as checking for acceptable ranges or cross-verifying data against known values.
- Check for Missing Values
- Identify any critical fields that are missing values and flag them for follow-up.
- Assess Data Integration Quality
- Review how well data is integrated across systems, ensuring smooth data flow between departments and platforms.
- Verify Data Sources
- Ensure that data comes from trusted, accurate, and reliable sources, and check for any issues with data provenance.
- Review User Input Quality
- Check for user-generated data input errors (e.g., mistyped fields or incorrect selections) that may have impacted data quality.
- Assess Data Structure and Organization
- Review how data is structured and organized within systems to ensure it is logical and easy to access.
- Check System Logs for Errors
- Review system logs for any errors or warnings related to data collection and entry processes.
- Verify Historical Data Trends
- Compare current data with historical trends to identify any inconsistencies or anomalies that might indicate data quality issues.
- Perform Cross-Departmental Data Checks
- Ensure that the data being used across departments (e.g., marketing, sales, finance) aligns and is consistent.
Phase 3: Reporting and Documentation (Steps 41–60)
- Document Findings
- Record all findings from the data assessment, including any identified issues or discrepancies.
- Create Data Quality Reports
- Prepare detailed reports summarizing the data assessment results, highlighting areas of concern, and noting the impact of any identified issues.
- Use Data Dashboards for Reporting
- Use data dashboards to visualize the assessment results, providing stakeholders with a clear overview of data quality metrics.
- Tag Issues by Severity
- Tag and categorize the identified data issues based on their severity (e.g., critical, high, medium, low).
- Record Root Causes of Data Issues
- For each identified issue, document the root cause (e.g., data entry error, system malfunction, process breakdown).
- Track Recurrent Issues
- Identify recurring issues that require attention and indicate systemic problems in the data collection process.
- Provide Actionable Recommendations
- Provide specific, actionable recommendations for improving data quality, including process changes, training, or system improvements.
- Establish Correction Deadlines
- Set realistic deadlines for addressing the identified issues and improving data quality.
- Prepare Stakeholder Updates
- Prepare a concise update for stakeholders, summarizing key findings and actions to be taken based on the data assessment.
- Report Data Quality Metrics
- Report on key metrics such as data accuracy, completeness, consistency, and timeliness.
- Review Assessment Findings with Key Teams
- Hold meetings with relevant teams (e.g., data collection, marketing, sales) to discuss the findings and determine next steps.
- Create Action Plans for Each Department
- Develop action plans for departments based on assessment results, focusing on how they can improve data quality moving forward.
- Set Priorities for Improvement
- Prioritize issues based on their impact on decision-making, compliance, or operational efficiency.
- Initiate Corrective Actions
- Begin implementing corrective actions, such as process changes, additional training, or adjustments to systems.
- Share Reports with Senior Management
- Provide senior management with a summary of the data assessment and improvement plans.
- Distribute Reports to Data Collection Teams
- Share detailed reports with data collection teams, ensuring they understand the issues and the actions needed to correct them.
- Document Data Quality Procedures
- Maintain thorough documentation of data quality procedures, including standards, checklists, and validation rules.
- Implement Feedback Loops
- Ensure that feedback from stakeholders is incorporated into future data quality assessments.
- Review Data Quality Improvement Progress
- Schedule periodic reviews of data quality improvement initiatives to ensure that corrective actions are being implemented effectively.
- Establish Follow-Up Assessment Cycles
- Set up follow-up assessments to evaluate whether improvements are successful and whether additional corrective actions are needed.
Phase 4: Continuous Improvement and Monitoring (Steps 61–80)
- Schedule Ongoing Monitoring
- Set up continuous monitoring of data inputs and outputs to detect any emerging issues before they become widespread.
- Create Data Quality Dashboards
- Develop real-time dashboards to monitor key data quality metrics on an ongoing basis.
- Track Improvements Over Time
- Keep track of improvements in data quality metrics over time to assess the effectiveness of implemented changes.
- Update Validation Rules Regularly
- Periodically review and update validation rules based on new business needs or emerging data challenges.
- Introduce Real-Time Alerts
- Set up automated alerts for critical data issues, such as missing data or discrepancies between systems.
- Benchmark Data Quality Progress
- Establish benchmarks for data quality and track progress toward meeting those benchmarks.
- Encourage Data Quality Culture
- Foster a culture of data quality across teams by emphasizing its importance in decision-making and training employees regularly.
- Refine Data Collection Processes
- Continuously refine data collection processes based on feedback from assessments and changing organizational needs.
- Identify and Address Data Gaps
- Regularly assess data gaps and work with teams to close them by improving data collection methods.
- Evaluate Vendor and Tool Performance
- Assess the performance of third-party tools and vendors used in data collection to ensure they meet accuracy standards.
- Conduct Peer Reviews
- Organize peer reviews of data assessment processes to ensure they remain effective and comprehensive.
- Regularly Review Data Integrity Policies
- Periodically review and update data integrity policies to ensure they remain aligned with industry best practices.
- Enhance Training for Data Teams
- Provide ongoing training to data collection and analysis teams to improve their ability to maintain accurate data.
- Collaborate Across Departments
- Foster collaboration between departments to share insights and best practices for maintaining data accuracy.
- Evaluate Data Entry Tools
- Regularly assess the performance and effectiveness of data entry tools and software, and make necessary improvements.
- Implement Automated Data Cleaning
- Where possible, implement automated tools to clean and validate data at the point of entry.
- Track Cost of Poor Data Quality
- Quantify the financial or operational impact of poor data quality to emphasize the need for continuous improvement.
- Monitor Data Entry Trends
- Track trends in data entry quality, identifying whether certain teams or processes are prone to more errors.
- Benchmark Against Industry Standards
- Compare your organization’s data quality practices with industry standards to identify areas for improvement.
- Engage in Continuous Improvement Initiatives
- Continuously engage in initiatives to improve data quality, ensuring that it evolves with the organization’s needs.
Phase 5: Final Reporting and Decision-Making (Steps 81–100)
- Prepare Summary Reports
- Summarize all assessment findings, improvements made, and recommendations for further action.
- Present Findings to Leadership
- Present data quality findings to leadership, emphasizing the importance of maintaining accurate data.
- Review Effectiveness of Corrective Actions
- Evaluate whether corrective actions taken after previous assessments were effective in improving data quality.
- Determine ROI of Data Quality Efforts
- Analyze the return on investment (ROI) of your data quality initiatives, such as improved decision-making, reduced errors, or cost savings.
- Conduct Final Assessment
- Perform a final review of the data quality assessments, evaluating whether improvements have been sustained.
- Provide Final Recommendations
- Offer any final recommendations based on the entire assessment cycle to further enhance data accuracy.
- Update Data Quality Procedures
- Update internal procedures to reflect any changes that have been made based on the assessment results.
- Share Success Stories
- Share successful improvements and examples with other departments to promote a focus on data quality.
- Document Long-Term Data Improvement Plans
- Develop a long-term plan for continuous data quality improvements, factoring in future needs and organizational growth.
- Monitor Adoption of Changes
- Ensure that changes are adopted consistently across the organization, monitoring compliance with new standards.
- Track Data Quality Trends Over Time
- Regularly track improvements in data quality to demonstrate the effectiveness of your strategies.
- Conduct Follow-Up Reviews
- Set up a schedule for follow-up reviews to ensure that data quality is consistently maintained.
- Provide Training for New Hires
- Ensure that new employees understand the importance of data accuracy and are trained on best practices.
- Strengthen Data Governance Practices
- Strengthen data governance policies and frameworks to maintain accuracy across the organization.
- Foster a Data-Driven Culture
- Encourage all teams to prioritize data quality and accuracy in their daily tasks.
- Reinforce Communication Across Teams
- Keep the lines of communication open between teams to address any new data quality challenges.
- Conduct Data Quality Audits
- Set up periodic data quality audits to ensure that standards are being met and maintained.
- Celebrate Data Quality Milestones
- Celebrate improvements and milestones achieved in data quality to motivate teams.
- Incorporate Feedback Loops
- Incorporate feedback from assessments into the next round of data quality initiatives to make improvements.
- Evaluate and Close the Assessment Cycle
– Once all corrective actions are completed, evaluate the overall success of the data assessment process and close the cycle, preparing for the next round.
By following this 100-step process, SayPro can ensure that data accuracy is consistently maintained, enabling better decision-making, improved operational efficiency, and more reliable business insights.
Leave a Reply
You must be logged in to post a comment.