Your cart is currently empty!
SayPro Provide examples of best practices for data analysis in policy recommendation development.
SayPro is a Global Solutions Provider working with Individuals, Governments, Corporate Businesses, Municipalities, International Institutions. SayPro works across various Industries, Sectors providing wide range of solutions.
Email: info@saypro.online Call/WhatsApp: + 27 84 313 7407
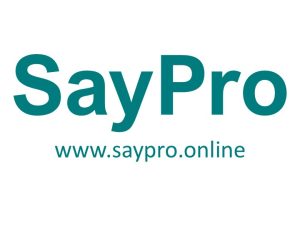
SayPro Use of Mixed-Methods Approach
- Best Practice: Combine both quantitative (numerical data) and qualitative (descriptive data) methods to gain a holistic understanding of the issue at hand.
- Example: SayPro could use employee satisfaction surveys (quantitative) alongside focus group discussions or interviews (qualitative) to understand the factors influencing employee engagement and retention.
SayPro Data Validation and Quality Assurance
- Best Practice: Ensure data accuracy and reliability by implementing robust data validation processes, such as cross-checking sources and using standardized measurement tools.
- Example: Before analyzing employee performance metrics, SayPro could validate the data by cross-referencing it with external benchmarks or historical data to ensure its accuracy and consistency.
SayPro Establish Clear Key Performance Indicators (KPIs)
- Best Practice: Define specific KPIs to measure the success of policies once implemented. This allows for targeted analysis and tracking of progress toward policy goals.
- Example: SayPro could establish KPIs like reduced turnover rates, improved productivity, or higher employee satisfaction scores as measurable outcomes to evaluate the effectiveness of a new employee retention policy.
SayPro Scenario Analysis and Forecasting
- Best Practice: Use scenario analysis to explore the potential outcomes of different policy options under varying conditions, helping to identify the most viable recommendations.
- Example: If SayPro is considering changes to its benefits package, scenario analysis could help forecast how changes in employee benefits might impact productivity, retention, and costs under different economic conditions.
SayPro Data Segmentation and Customization
- Best Practice: Segment data based on relevant factors (e.g., department, employee tenure, demographic) to provide a more granular understanding of the issues and to tailor policy recommendations.
- Example: SayPro could analyze data on employee turnover by department, age group, or length of employment to understand the factors driving turnover in specific segments and create targeted interventions.
SayPro Time-Series Analysis
- Best Practice: Use time-series analysis to assess trends over time and forecast future trends based on historical data. This helps identify emerging patterns and inform forward-looking policy recommendations.
- Example: SayPro could analyze the trend of employee retention over the past few years to predict future turnover rates and develop proactive retention strategies.
SayPro Cross-Referencing with External Data
- Best Practice: Compare internal data with relevant external data, such as industry reports or regional economic data, to gain insights into broader trends and align recommendations with external factors.
- Example: SayPro could compare its employee satisfaction data with industry benchmarks to see how it stacks up against competitors and adjust its policies accordingly.
SayPro Data Visualization for Clear Communication
- Best Practice: Use clear, accessible visualizations (e.g., graphs, charts, infographics) to present complex data in a way that stakeholders can easily understand and use for decision-making.
- Example: SayPro could use bar graphs or pie charts to illustrate employee satisfaction levels across different departments, making it easier for decision-makers to identify areas for improvement.
SayPro Use of Advanced Analytics and Machine Learning
- Best Practice: Leverage advanced analytical techniques such as machine learning or predictive modeling to uncover hidden patterns and insights from complex data.
- Example: SayPro could use predictive modeling to identify employees at risk of leaving the company and develop targeted interventions based on factors like job satisfaction, tenure, and performance.
SayPro Engagement with Stakeholders in the Data Analysis Process
- Best Practice: Engage stakeholders early in the data analysis process to ensure that the data being analyzed is relevant and the findings are aligned with their needs.
- Example: SayPro could involve department heads and team leaders in identifying key metrics that should be analyzed to develop recommendations that address specific challenges within their teams.
SayPro Continuous Monitoring and Iterative Analysis
- Best Practice: Regularly monitor the data and conduct ongoing analysis to refine policies based on emerging trends, ensuring that policies remain relevant and effective.
- Example: After implementing a new policy, SayPro could continuously monitor employee feedback and performance metrics, making adjustments to the policy as new insights emerge over time.
SayPro Benchmarking Against Best Practices
- Best Practice: Regularly benchmark against industry best practices and high-performing organizations to ensure that policy recommendations reflect current trends and standards.
- Example: SayPro could review employee retention strategies used by top-performing companies in the industry and adapt their own practices based on these findings.
SayPro Transparent and Collaborative Data Sharing
- Best Practice: Ensure that data analysis is transparent and shared with relevant stakeholders, allowing for collaborative review and feedback. This builds trust and improves the final policy recommendations.
- Example: SayPro could make data analysis reports available to key internal teams, allowing them to provide input and refine the policy recommendations before final decisions are made.
Leave a Reply
You must be logged in to post a comment.