Your cart is currently empty!
SayPro Sample a minimum of 200 data points per data source, with an emphasis on high-priority areas like marketing data, user engagement metrics, and sales data.
SayPro is a Global Solutions Provider working with Individuals, Governments, Corporate Businesses, Municipalities, International Institutions. SayPro works across various Industries, Sectors providing wide range of solutions.
Email: info@saypro.online Call/WhatsApp: + 27 84 313 7407
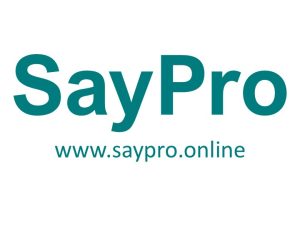
SayPro: Sample a Minimum of 200 Data Points per Data Source
Objective: To ensure robust data quality evaluation across key performance metrics, SayPro will sample at least 200 data points from each data source, with a specific focus on high-priority areas such as marketing data, user engagement metrics, and sales data. This process will help to ensure that the data used for decision-making is accurate, reliable, and aligned with organizational goals.
Step-by-Step Process for Data Sampling
1. Define the Data Sources to Be Sampled
Identify the key data sources that require sampling. For SayPro, focus on high-priority areas that have a direct impact on marketing strategy, user engagement, and sales performance:
- Marketing Data: Information collected from marketing campaigns (e.g., ad spend, ROI, conversion rates, impressions, CTR).
- User Engagement Metrics: Data from website interactions, mobile app activity, and social media engagement (e.g., page views, session duration, social shares, click-through rates).
- Sales Data: Sales figures from CRM systems, order history, and customer purchase behavior (e.g., revenue, total orders, average transaction value).
2. Establish Sampling Criteria
Set clear criteria to guide the selection of data points for each data source. These criteria will ensure that the sample is representative of the entire data set:
- Random Sampling: Ensure a random selection of data points to avoid bias.
- Time Range: Ensure the sample includes data from various time periods (e.g., weekly, monthly) to assess trends and outliers.
- Diversity of Metrics: Include data points that represent a range of key metrics within each source (e.g., campaign performance, user actions, sales outcomes).
- Priority Metrics: Focus more heavily on metrics that have a direct impact on decision-making (e.g., conversion rates in marketing, user retention in engagement metrics, and total revenue in sales data).
3. Select Data Points from Each Data Source
For each data source, choose at least 200 data points according to the established sampling criteria:
For Marketing Data:
- Ad Spend: Randomly select data points from marketing campaigns to ensure a representative sample of different ad platforms, campaign types, and time periods.
- Conversion Rates: Include data from a mix of high-performing and low-performing campaigns to understand the full range of performance.
- Impressions and Click-Through Rates (CTR): Choose campaigns across different channels (e.g., digital ads, email marketing, social media).
For User Engagement Metrics:
- Page Views: Randomly sample data from various landing pages and key website sections.
- Session Duration: Select a variety of user sessions (short and long) to evaluate engagement across different content types.
- Social Shares: Choose data points representing a diverse set of content and engagement levels (e.g., highly shared vs. less popular posts).
For Sales Data:
- Revenue: Sample a variety of orders, including high and low-value transactions, to assess the overall sales performance.
- Total Orders: Select data points across different time periods (daily, weekly, monthly) to observe trends in order volume.
- Average Transaction Value: Analyze data across different customer segments or product categories to get a complete view of average order values.
4. Assess Data Quality for the Sampled Points
Perform quality checks on the 200 sampled data points for each data source. For each data set, check for:
- Accuracy: Verify that the data correctly represents the actual values or actions it is meant to track (e.g., checking if revenue data matches sales records).
- Completeness: Ensure that there are no missing or incomplete values within the sampled data points.
- Consistency: Confirm that the formatting and naming conventions are consistent (e.g., uniform date formats, consistent campaign names).
- Timeliness: Check if the data is up to date and relevant (e.g., if user engagement metrics reflect the most recent activity, or if sales data reflects current product prices).
- Validity: Ensure that the sampled data points adhere to predefined standards (e.g., valid email addresses or correctly calculated conversion rates).
5. Document Findings and Flag Issues
After completing the sampling and quality checks, document any discrepancies, errors, or anomalies identified in the data. For example:
- Marketing Data: Incorrect ad spend reporting, missing or incorrect conversion rates, discrepancies in CTR.
- User Engagement Metrics: Missing page views for some key landing pages, incorrect session duration records, incomplete social shares data.
- Sales Data: Inaccurate revenue figures, missing orders, discrepancies in average transaction values.
6. Generate Reports for Data Quality Assessment
Prepare detailed reports that summarize the findings from the sampled data points. The report should include:
- Introduction: Overview of the data sources sampled (marketing, user engagement, and sales).
- Methodology: Explanation of the sampling process, criteria, and selection.
- Findings: A summary of any data quality issues identified during the sampling process, organized by data source.
- Recommendations: Suggested corrective actions for any identified issues, such as refining data entry practices, improving tracking codes, or standardizing reporting formats.
7. Make Recommendations for Data Improvement
Based on the findings, collaborate with relevant teams to propose actionable steps to address any identified data quality issues. For example:
- Marketing Data: Review campaign tracking methods to ensure that all data points (e.g., ad spend, conversions) are recorded consistently. Consider using automated reporting tools to minimize human error.
- User Engagement Metrics: Investigate tracking discrepancies and ensure that user interactions are captured correctly across all platforms. Set up automated alerts for missing data.
- Sales Data: Implement stricter validation rules for order entries and ensure that sales data is pulled from a unified system to avoid inconsistencies between platforms.
8. Monitor and Re-assess
To ensure continuous improvement in data quality:
- Ongoing Monitoring: Set up periodic checks to monitor data quality across the sampled data points, adjusting for any trends or changes.
- Follow-up Assessments: After corrective actions are implemented, conduct follow-up assessments to ensure that improvements have been successfully integrated into the data collection and reporting processes.
Example Data Sampling Summary
Marketing Data Sampling
- Sampled Data Points: 200 campaign performance data points across digital ads, email marketing, and social media platforms.
- Key Findings:
- 10% of campaigns had missing or incomplete conversion rate data.
- 5% of campaigns showed discrepancies between reported impressions and actual ad spend.
- Recommendations: Improve data entry processes for conversion tracking and ensure uniform data reporting templates for all campaigns.
User Engagement Metrics Sampling
- Sampled Data Points: 200 data points across website traffic (page views), session duration, and social media engagement metrics.
- Key Findings:
- 7% of page views data were missing from high-traffic landing pages.
- 3% of social shares data were inaccurate due to incorrect tracking codes.
- Recommendations: Conduct a review of tracking setups for social shares and page views, and implement automated checks for missing data.
Sales Data Sampling
- Sampled Data Points: 200 records across customer orders, total revenue, and average transaction value.
- Key Findings:
- 5% of revenue records had discrepancies due to inconsistent product pricing entries.
- 8% of transactions were missing order values in CRM systems.
- Recommendations: Ensure that product prices are correctly updated in CRM systems and implement stricter order entry validation.
Conclusion
By sampling a minimum of 200 data points from each key data source, SayPro can comprehensively evaluate data quality across marketing, user engagement, and sales data. This systematic approach helps identify issues early and take corrective actions to improve the accuracy, consistency, and completeness of the data, leading to better decision-making and more effective strategy execution.
Leave a Reply
You must be logged in to post a comment.