Your cart is currently empty!
SayPro Study Data:Comprehensive data from the relevant studies conducted on target populations within the period.
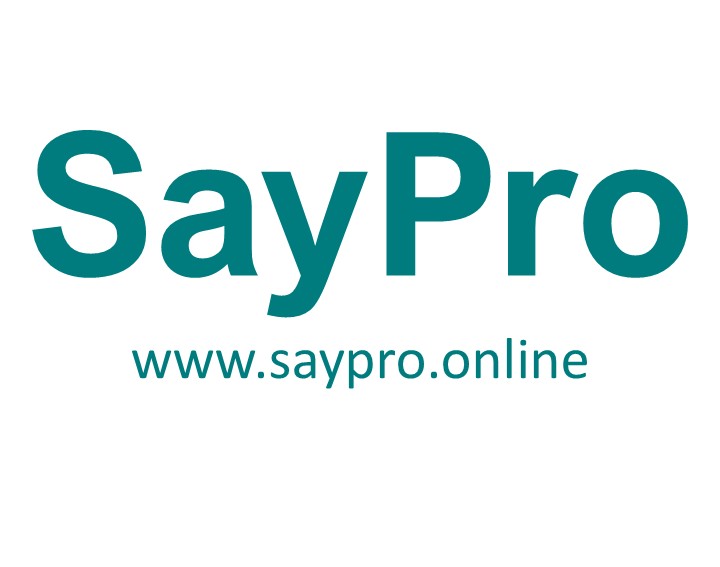
SayPro Study Data: Comprehensive Data from Relevant Studies Conducted on Target Populations Within the Period
In the context of SayPro’s Monitoring, Evaluation, and Learning (MEL) process, study data serves as the foundation for understanding the impact of various programs on the target populations. The collection and analysis of comprehensive study data are critical for evaluating how well interventions have been implemented and whether they are achieving the intended outcomes.
Below is a detailed breakdown of the types of data collected, the methods used to ensure that data is comprehensive, and how this data can be applied to inform programmatic decisions at SayPro:
1. Types of Study Data Collected
SayPro collects data from a variety of research methodologies and evaluation techniques to form a well-rounded understanding of program impact. The data can be broadly categorized into the following:
a) Demographic Data
Purpose: Demographic data helps identify the characteristics of the target populations and segments within them. It is essential for understanding who benefits from a program and ensuring that interventions are reaching the right people.
- Age
- Gender
- Socioeconomic status (income, employment, etc.)
- Location (urban vs. rural, region-specific data)
- Educational background
- Ethnic and cultural factors
Example: Collecting data on the age, gender, and educational background of participants in an education-focused program to assess whether the intervention is reaching the most underserved communities.
b) Baseline Data
Purpose: Baseline data represents the initial status of key indicators before program implementation. It is used to compare changes over time to assess the impact of the intervention.
- Knowledge, attitudes, and practices (KAP) surveys: These surveys gauge the baseline knowledge, attitudes, and practices of the target population.
- Health metrics: Data on health outcomes such as disease prevalence, maternal health indicators, etc.
- Behavioral indicators: Measures related to specific behaviors the program aims to influence (e.g., vaccination rates, sanitation habits, financial literacy).
Example: For a health program aimed at reducing malnutrition, baseline data would include statistics on the prevalence of malnutrition in the target population before the intervention.
c) Process Data
Purpose: Process data tracks program implementation activities and outputs. This data monitors how well the program is being executed and ensures that activities are carried out according to the planned schedule.
- Training sessions conducted (number, duration, attendance)
- Outreach efforts (e.g., the number of community meetings or materials distributed)
- Service delivery metrics (e.g., number of individuals receiving medical services or accessing educational resources)
Example: For a vocational training program, process data would include the number of people enrolled, the types of training offered, and the frequency of classes held.
d) Outcome Data
Purpose: Outcome data measures the immediate effects of the program on the target population. This data typically assesses changes in knowledge, attitudes, and behaviors that result from program activities.
- Change in knowledge or skills (measured through tests or surveys)
- Changes in attitudes or perceptions (assessed through surveys or interviews)
- Behavioral changes (e.g., increased use of healthcare services, improved financial decision-making)
Example: After completing a literacy program, outcome data would measure the increase in reading and writing skills, as assessed through pre- and post-test surveys.
e) Impact Data
Purpose: Impact data assesses the long-term outcomes of the program. This data helps determine whether the program has brought about the desired changes in the community or population over a sustained period of time.
- Long-term health outcomes (e.g., reduced child mortality, improved nutritional status)
- Economic outcomes (e.g., increased employment, improved income levels)
- Social outcomes (e.g., changes in social behavior, community cohesion, empowerment)
Example: For a livelihoods program, impact data would include follow-up surveys to track whether participants have maintained or increased their income levels a year or two after completing the program.
f) Qualitative Data
Purpose: Qualitative data provides deeper insight into the contextual factors and personal experiences that might not be captured through quantitative measures. This data is invaluable for understanding the why behind observed changes in behavior or outcomes.
- In-depth interviews with participants, community leaders, or stakeholders
- Focus group discussions (FGDs) to explore community-level perceptions
- Case studies that track individual or household-level changes over time
Example: Conducting focus group discussions with participants of a gender-based violence prevention program to understand the program’s impact on community attitudes toward gender equality.
2. Methods to Ensure Comprehensive Data Collection
To ensure that the data collected is comprehensive, accurate, and actionable, SayPro employs various data collection methods that are appropriate to the specific needs of each program and study. These methods include:
a) Surveys and Questionnaires
Surveys and questionnaires are commonly used for collecting both quantitative and qualitative data from a large number of participants. Well-designed surveys can provide insights into demographic details, behavior changes, attitudes, and program outcomes.
- Closed-ended questions for quantitative analysis (e.g., Likert scales, multiple-choice questions)
- Open-ended questions for qualitative insights (e.g., personal experiences, feedback on program activities)
Example: Distributing a post-program survey to assess participants’ satisfaction and perceived effectiveness of a vocational training course.
b) Interviews
In-depth interviews are often conducted with key informants (e.g., program participants, community leaders, stakeholders) to gather rich qualitative data. Interviews allow for exploration of personal stories and the impact of the program from an individual’s perspective.
- Semi-structured interviews allow flexibility while ensuring consistency across respondents.
- Key informant interviews (KIIs) target specific individuals who can provide unique insights into the program’s outcomes.
Example: Interviewing community health workers to understand the challenges they face in delivering a health program, and whether these challenges impact program success.
c) Focus Group Discussions (FGDs)
FGDs are used to gather qualitative insights from a group of participants to explore a particular issue, behavior, or topic in more detail. They are useful for uncovering shared community perceptions, barriers, and enabling factors for success.
- Group dynamics can encourage open conversation and lead to insights that might not emerge in individual interviews.
Example: Conducting an FGD with farmers in a rural area to discuss the impact of an agricultural training program on their farming practices.
d) Administrative and Program Data
This type of data is collected directly from program records or administrative databases, such as attendance logs, service usage records, and activity reports.
- Service delivery data: Tracks the volume of services provided (e.g., number of medical consultations, number of students enrolled).
- Operational data: Includes program output metrics such as resources spent, number of materials distributed, etc.
Example: Collecting data on the number of individuals who received malaria prevention education and follow-up data on those who adopted protective behaviors.
e) Observation
Direct observation is another method used to collect qualitative data on program activities. It involves monitoring participants in natural settings or during program interventions to understand real-time behavior and engagement.
Example: Observing participants in a community-based health education program to assess their engagement and understanding of key messages.
3. Data Storage and Management
To ensure data is comprehensive, consistent, and reliable, SayPro follows a robust data storage and management system that allows easy access and protection of data throughout the process.
Key Considerations:
- Data Quality Control: Ensuring that all collected data is checked for accuracy, consistency, and completeness. This includes reviewing data entry, ensuring proper coding, and conducting periodic audits.
- Data Security: Using encrypted systems and maintaining strict protocols for data access to ensure that sensitive information is protected.
- Data Integration: Combining data from different sources (e.g., surveys, interviews, focus groups) into a unified dataset to enable comprehensive analysis.
Example: Using a cloud-based data management platform like DHIS2 or KoboToolbox to store, manage, and analyze data in real-time, while ensuring that it is backed up and secure.
4. Application of Study Data in Programmatic Decisions
The comprehensive study data collected plays a critical role in informing programmatic decisions at SayPro. This data is used to:
- Measure program effectiveness: Data provides evidence of whether programs are achieving the intended outcomes, helping stakeholders decide whether to scale, modify, or discontinue programs.
- Identify gaps or challenges: By analyzing data, SayPro can identify areas where programs may not be working as expected and suggest corrective actions.
- Advocate for funding or support: Comprehensive data supports advocacy efforts by providing strong evidence of program impact, which can be used to secure continued or increased funding.
- Improve program design: Findings from data analysis help inform future program designs, ensuring they are more targeted, efficient, and impactful.
Example: After analyzing post-program surveys, SayPro may identify that a certain segment of the population is not engaging with the program due to specific barriers (e.g., transportation issues, lack of awareness). This data can be used to refine outreach strategies or modify program activities to increase engagement.
Conclusion
The comprehensive study data collected through a variety of methodologies plays a critical role in understanding the effectiveness of SayPro’s programs. This data helps measure outcomes, track changes in the target population, and inform future decision-making. By ensuring the accuracy, quality, and comprehensiveness of this data, SayPro can make well-informed decisions that ultimately lead to more impactful interventions for the populations it serves.
Leave a Reply
You must be logged in to post a comment.