Your cart is currently empty!
SayPro “Suggest 100 ways to analyze monitoring data to identify areas for strategic adjustments.”
SayPro is a Global Solutions Provider working with Individuals, Governments, Corporate Businesses, Municipalities, International Institutions. SayPro works across various Industries, Sectors providing wide range of solutions.
Email: info@saypro.online Call/WhatsApp: + 27 84 313 7407
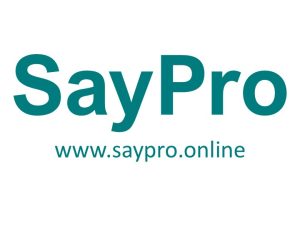
Here is a comprehensive list of 100 ways to analyze monitoring data to identify areas for strategic adjustments in programs. These methods can help uncover insights from monitoring feedback, allowing for data-driven decision-making and program improvements.
1. Descriptive Analysis
- Summarize key performance indicators (KPIs) to track overall progress.
- Calculate averages of core program metrics to identify general trends.
- Examine trends over time to detect patterns in program performance.
- Compare baseline data with current results to measure progress against initial expectations.
- Calculate the variance between expected and actual outcomes to assess alignment.
- Use frequency distributions to understand how often particular outcomes occur.
- Break data into categories (e.g., gender, age group, location) to see how different segments are performing.
- Conduct a cohort analysis to assess the performance of specific groups over time.
- Measure percentage changes to see shifts in key indicators.
- Perform cross-sectional analysis to compare different program components or geographic regions.
2. Data Visualization
- Create trend graphs to visually track performance over time.
- Use bar charts to compare results across different categories or groups.
- Develop heat maps to identify geographic areas with performance disparities.
- Generate pie charts to understand the distribution of various program outcomes.
- Create scatter plots to explore relationships between two or more variables.
- Use histograms to visualize the distribution of outcomes across different data points.
- Implement line graphs to track changes in program data at regular intervals.
- Use dashboards to display real-time program performance metrics.
- Create box plots to visualize the range and distribution of key metrics.
- Generate radar charts to show multi-variable comparisons across different program components.
3. Comparative Analysis
- Compare performance against baseline targets to identify gaps.
- Benchmark performance against other similar programs to gauge effectiveness.
- Evaluate performance against industry standards to assess relative success.
- Compare sub-group performances (e.g., rural vs. urban) to determine focus areas.
- Compare different time periods (e.g., monthly, quarterly) to detect shifts.
- Cross-compare different interventions to identify which is most effective.
- Evaluate regional differences in performance to prioritize interventions.
- Compare costs vs. benefits across program activities to improve resource allocation.
- Analyze program performance by season to determine if timing adjustments are needed.
- Compare program outputs to outcomes to assess the effectiveness of the activities.
4. Correlation and Causality
- Perform correlation analysis to understand relationships between different variables.
- Use regression analysis to quantify relationships between input variables and outcomes.
- Analyze correlation between resource allocation and program success to optimize budget use.
- Identify the root causes of low performance by analyzing patterns and relationships.
- Assess how external factors (e.g., economic changes, policy shifts) impact program performance.
- Use multivariate analysis to examine the impact of multiple factors on outcomes.
- Conduct path analysis to model and test direct and indirect relationships between variables.
- Use causal inference methods to determine the cause-and-effect relationships.
- Conduct statistical significance tests to verify the reliability of observed patterns.
- Perform sensitivity analysis to assess how changes in key variables impact results.
5. Segmentation Analysis
- Segment data by demographic factors (e.g., age, gender) to identify different impacts.
- Break data into geographical regions to compare results in different locations.
- Segment data by beneficiary type (e.g., individual vs. group beneficiaries).
- Use behavior-based segmentation to analyze how different groups interact with the program.
- Segment by risk factors (e.g., vulnerable populations) to tailor strategies.
- Group data by service or intervention type to assess which services yield the best results.
- Identify the most and least successful segments to refine targeting strategies.
- Conduct a cohort analysis based on timing (e.g., early vs. late participants).
- Segment by program phase (e.g., initiation, growth, sustainability) to identify stage-specific challenges.
- Use cluster analysis to group similar data points and uncover hidden patterns.
6. Trend Analysis
- Conduct time-series analysis to predict future trends based on historical data.
- Identify seasonal patterns that may affect program performance and adjust timing accordingly.
- Analyze cyclical trends (e.g., economic cycles) to determine their impact on outcomes.
- Look for outliers to understand anomalies that might need special attention.
- Track deviations from expected trends to detect emerging problems early.
- Use moving averages to smooth out short-term fluctuations and focus on long-term trends.
- Compare long-term and short-term trends to assess whether immediate changes are needed.
- Monitor frequency of events or behaviors to understand the consistency of outcomes.
- Identify early warning signs of program failure through trend shifts.
- Use exponential smoothing techniques to forecast future performance based on past data.
7. Impact Evaluation
- Conduct before-and-after comparisons to assess program effectiveness.
- Use control groups to compare the program’s impact with a non-participating group.
- Calculate impact ratios to measure the relative effectiveness of interventions.
- Assess net impact by subtracting baseline performance from current performance.
- Evaluate program outcomes relative to objectives to ensure alignment.
- Conduct counterfactual analysis to determine what would have happened without the program.
- Perform contribution analysis to understand how specific activities drive program outcomes.
- Use impact assessments to measure the sustainability of results after program completion.
- Track unintended consequences (both positive and negative) that may emerge over time.
- Analyze the program’s ability to meet long-term goals through delayed outcome measurements.
8. Performance Disaggregation
- Disaggregate data by gender to ensure equitable outcomes across gender groups.
- Analyze performance by age group to identify generational differences in program impact.
- Disaggregate results by income level to ensure inclusivity and fairness in program outcomes.
- Assess regional disparities to identify areas needing focused interventions.
- Disaggregate by type of beneficiary (e.g., community vs. institutional) for more tailored strategies.
- Analyze performance by educational level to understand how literacy impacts program outcomes.
- Disaggregate data by program component to identify specific areas needing adjustment.
- Examine performance based on cultural factors to ensure programs are culturally appropriate.
- Track the performance of different delivery mechanisms (e.g., online vs. in-person).
- Disaggregate by duration of program participation to see how long-term engagement affects outcomes.
9. Qualitative Data Analysis
- Conduct thematic analysis of open-ended feedback to identify key themes or issues.
- Use sentiment analysis to assess the emotional tone of qualitative feedback.
- Analyze case studies to gain deeper insights into individual beneficiary experiences.
- Categorize qualitative feedback to highlight recurring problems or successes.
- Identify bottlenecks or inefficiencies through detailed qualitative responses.
- Use content analysis to quantify the frequency of specific words or themes in feedback.
- Conduct interviews with beneficiaries to explore unquantifiable insights.
- Perform focus group analysis to understand group dynamics and collective feedback.
- Analyze direct quotes from beneficiaries to capture powerful, narrative-based insights.
- Use participatory evaluation methods to gather feedback directly from beneficiaries on their experiences.
10. Feedback Loop & Iterative Improvements
- Implement a feedback loop system to continuously collect and incorporate data into decision-making.
- Conduct rapid data analysis after each feedback cycle to make timely adjustments.
- Regularly update data collection methods to reflect changes in program activities or environment.
- Track the effectiveness of adjustments by analyzing changes in key metrics.
- Solicit ongoing feedback from key stakeholders (e.g., staff, beneficiaries, partners) on program adjustments.
- Implement frequent check-ins with field staff to get real-time data on program challenges.
- Conduct periodic retrospectives to evaluate how well past adjustments have worked.
- Use feedback from beneficiaries to identify areas where program design may be flawed.
- Integrate feedback from monitoring into strategic meetings to inform continuous improvement.
- Create iterative improvement cycles based on regular data analysis and stakeholder input.
This list provides diverse techniques to analyze monitoring data and identify areas for strategic adjustments. By employing these methods, you can continuously adapt and improve your programs to ensure they meet their objectives and are responsive to evolving needs. Let me know if you’d like more details on any of these methods!
Leave a Reply
You must be logged in to post a comment.